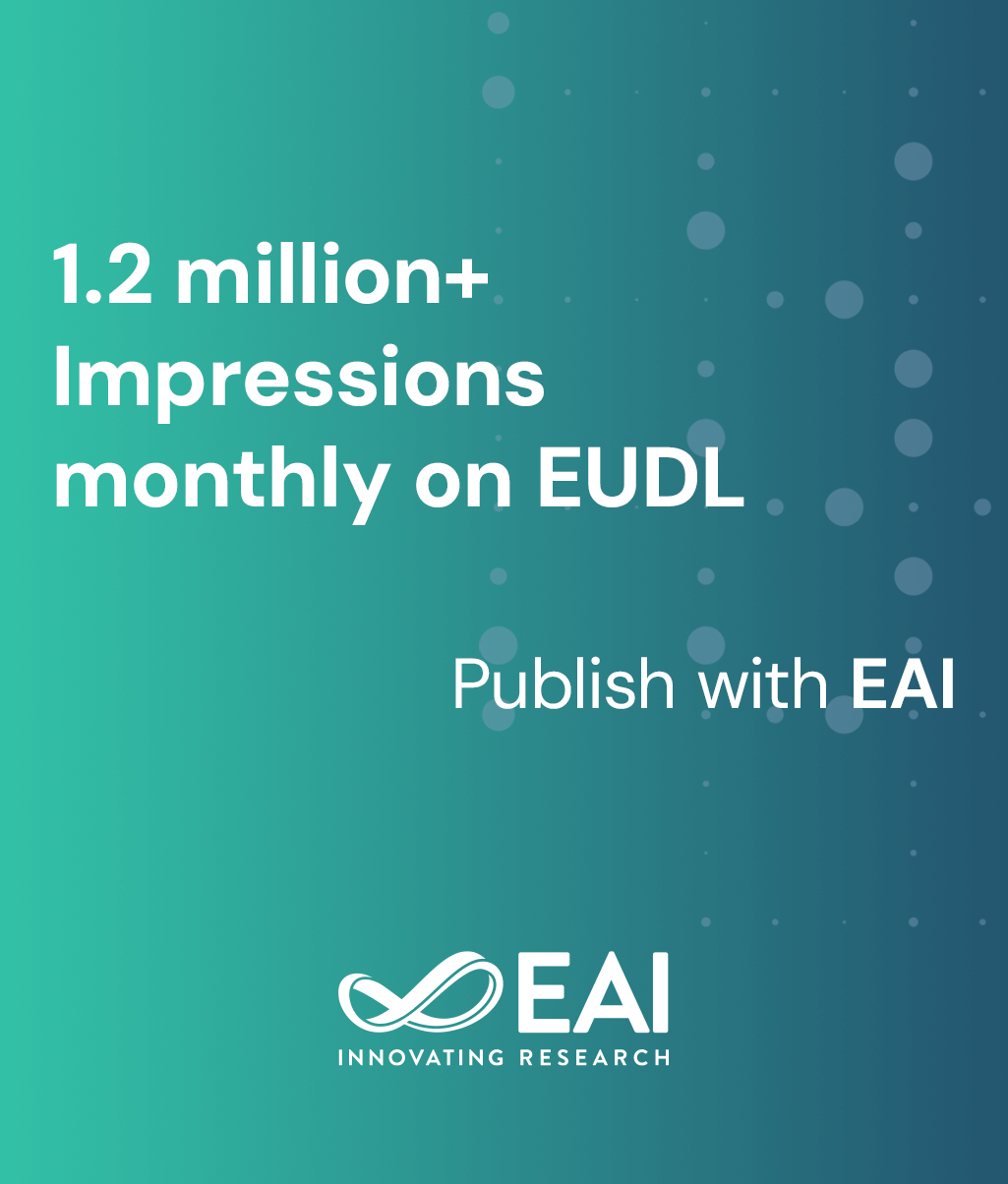
Research Article
Towards Multi-Model Big Data Road Traffic Forecast at Different Time Aggregations and Forecast Horizons
@ARTICLE{10.4108/ew.v9i39.1187, author={Riccardo Martoglia and Gabriele Savoia}, title={Towards Multi-Model Big Data Road Traffic Forecast at Different Time Aggregations and Forecast Horizons}, journal={EAI Endorsed Transactions on Energy Web}, volume={9}, number={39}, publisher={EAI}, journal_a={EW}, year={2022}, month={5}, keywords={Big Data Analytics, Time Series, Traffic Forecast, Time Aggregation, ARIMA, Apache Spark, Machine Learning}, doi={10.4108/ew.v9i39.1187} }
- Riccardo Martoglia
Gabriele Savoia
Year: 2022
Towards Multi-Model Big Data Road Traffic Forecast at Different Time Aggregations and Forecast Horizons
EW
EAI
DOI: 10.4108/ew.v9i39.1187
Abstract
Due to its usefulness in various social contexts, from Intelligent Transportation Systems (ITSs) to the reduction of urban pollution, road traffic prediction represents an active research area in the scientific community, with strong potential impact on citizens’ well-being. Already considered a non-trivial problem, in many real applications an additional level of complexity is given by the large amount of data requiring Big Data domain technologies. In this paper, we present the first steps of a novel approach integrating both classic and machine learning models in the Spark-based big data architecture of the H2020 CLASS project, and we perform preliminary tests to see how usually little-considered variables (different data aggregation levels, time horizons and traffic density levels) influence the error of the different models.
Copyright © 2022 R. Martoglia et al., licensed to EAI. This is an open access article distributed under the terms of the Creative Commons Attribution license (http://creativecommons.org/licenses/by/3.0/), which permits unlimited use, distribution and reproduction in any medium so long as the original work is properly cited.