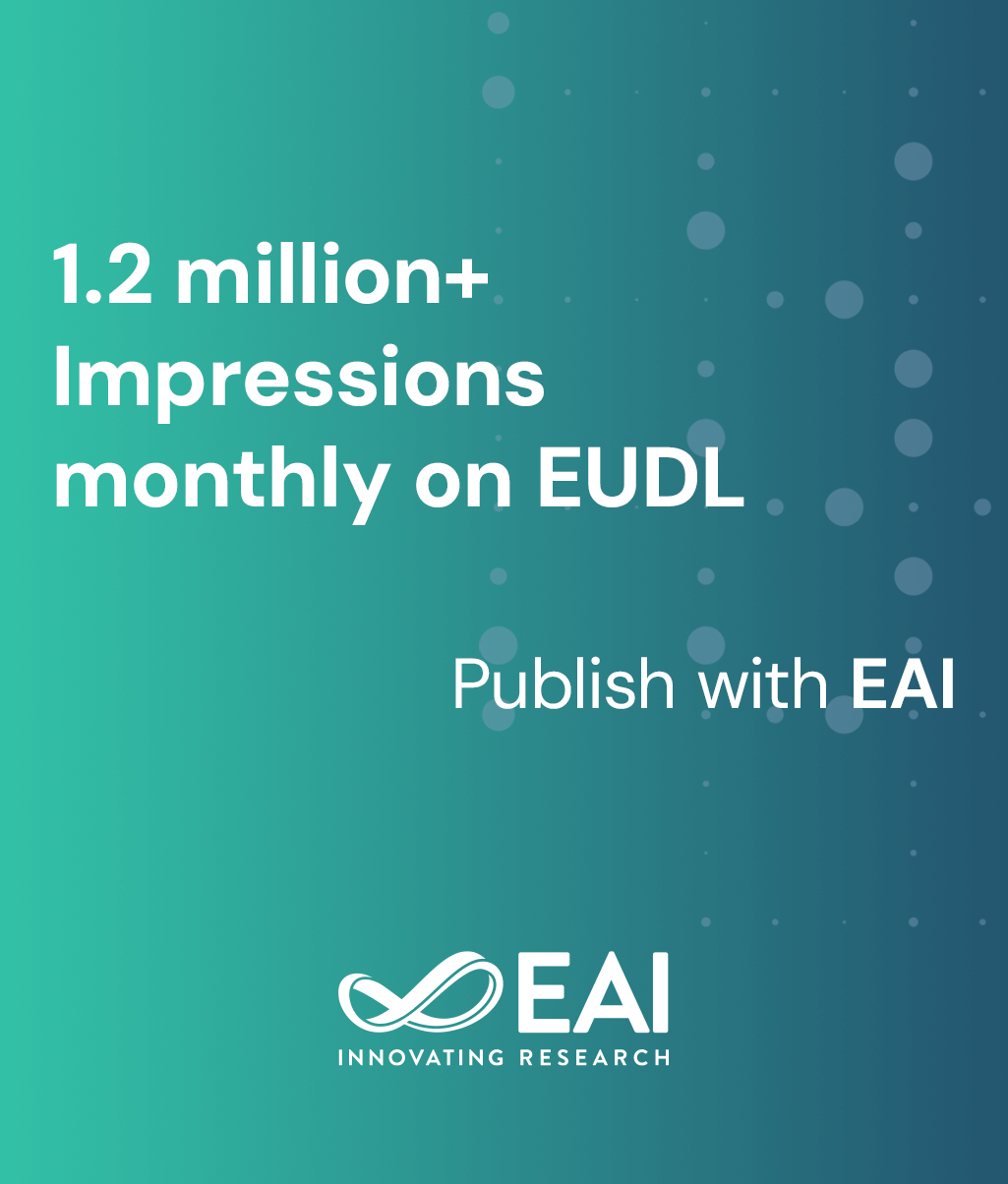
Editorial
Reinforcement Learning Data-Driven Optimal Load-Frequency Control for Power Systems
@ARTICLE{10.4108/ew.7500, author={Yi Zhao}, title={Reinforcement Learning Data-Driven Optimal Load-Frequency Control for Power Systems}, journal={EAI Endorsed Transactions on Energy Web}, volume={12}, number={1}, publisher={EAI}, journal_a={EW}, year={2025}, month={3}, keywords={Power system, reinforcement learning, data-driven, dynamic programming, Load frequency control}, doi={10.4108/ew.7500} }
- Yi Zhao
Year: 2025
Reinforcement Learning Data-Driven Optimal Load-Frequency Control for Power Systems
EW
EAI
DOI: 10.4108/ew.7500
Abstract
INTRODUCTION: Power systems are complex due to their time-varying and uncertain parameters, challenging conventional control methods. OBJECTIVES: This study proposes an adaptive dynamic programming (ADP) controller to address this limitation. The ADP controller eliminates the need for pre-existing knowledge of the system dynamics, a significant advantage in real-world applications. METHODS: By iteratively solving the Riccati equation using only system state and input data, the controller learns an approximate optimal control strategy. In this study, we use an iterative computational approach with an online adaptive optimal controller designed for unknown power system dynamics. RESULTS: Utilizing real-time collected system states and input information, even in the absence of knowledge about the power system matrix, we achieve iterative solutions for the algebraic Riccati equation, enabling the computation of an optimal controller. Simulation results demonstrate the ease of implementation of this approach in power system load frequency control (LFC). CONCLUSION: The proposed ADP controller exhibits good control performance of grid stability, making it a valuable reference for LFC, especially in scenarios with unknown system parameters.
Copyright © 2025 Y. Zhao et al., licensed to EAI. This is an open access article distributed under the terms of the CC BY-NC-SA 4.0, which permits copying, redistributing, remixing, transformation, and building upon the material in any medium so long as the original work is properly cited.