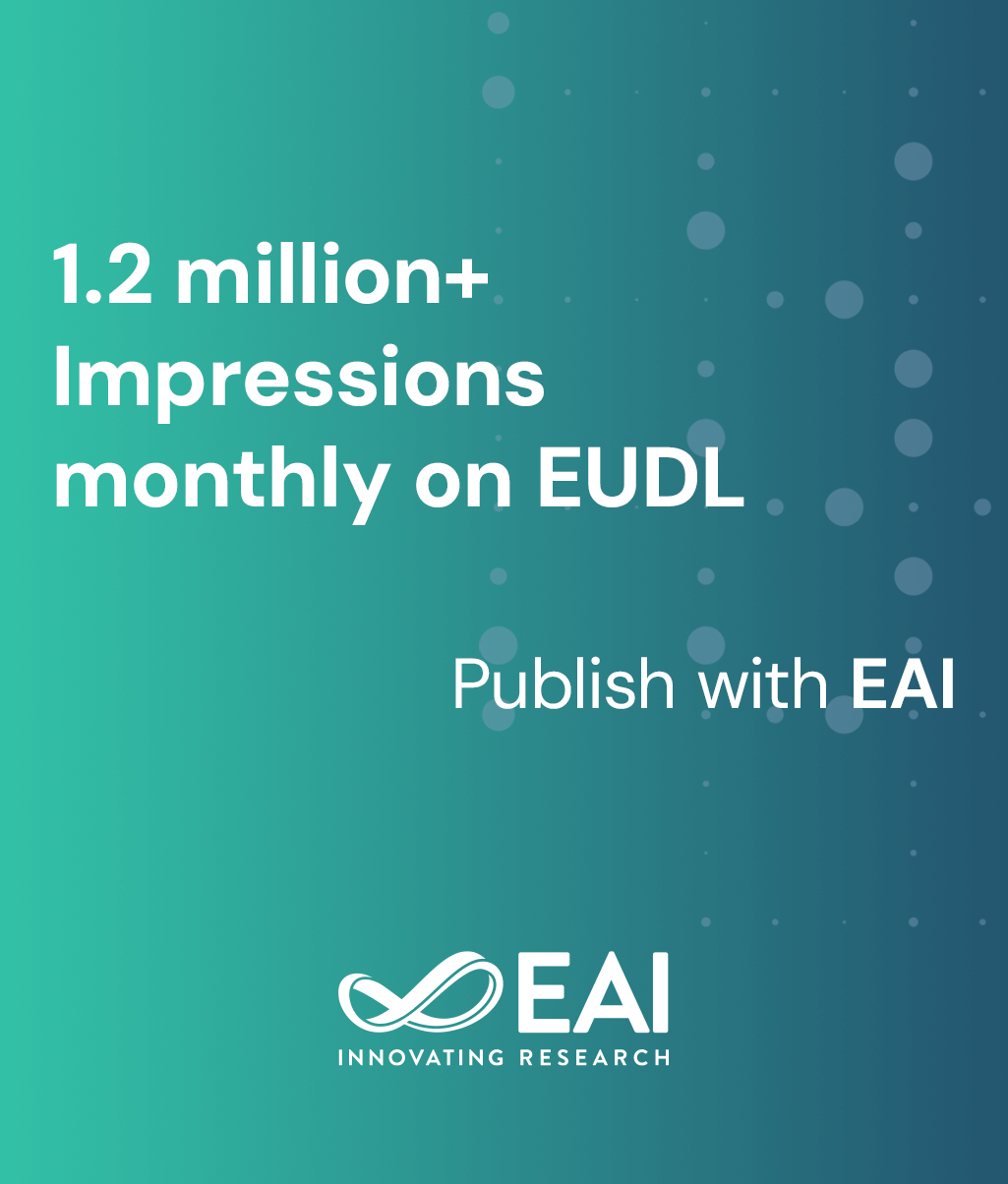
Editorial
Construction and Application Analysis of an Intelligent Distribution Network Identification System Based on Deep Neural Networks
@ARTICLE{10.4108/ew.5547, author={Yu Ma}, title={Construction and Application Analysis of an Intelligent Distribution Network Identification System Based on Deep Neural Networks}, journal={EAI Endorsed Transactions on Energy Web}, volume={12}, number={1}, publisher={EAI}, journal_a={EW}, year={2024}, month={12}, keywords={Deep neural network, Online topology identification, Topological labels, Intelligent distribution network, Light GBM algorithm}, doi={10.4108/ew.5547} }
- Yu Ma
Year: 2024
Construction and Application Analysis of an Intelligent Distribution Network Identification System Based on Deep Neural Networks
EW
EAI
DOI: 10.4108/ew.5547
Abstract
INTRODUCTION: At present, the communication between measuring data and network topology in the distribution system cannot be accurately established. Therefore, deep neural networks were utilized to learn the mapping relationship between the measurement data and network topology, achieving topology structure discrimination under different working conditions. OBJECTIVES: This study aims to establish a machine learning-based Intelligent Distribution Network (IDN) online topology recognition model to address the limited measurement equipment in distribution networks and improve the accuracy and efficiency of network topology recognition. METHODS: First, light GBM was used for feature selection to reduce computational complexity and improve learning efficiency. Then, a DNN model was constructed for topological identification and enhances the model scalability through incremental and transfer learning mechanisms. In addition, the Cross-Validation Grid Search Algorithm (GSA) was used to optimize the hyperparameters to ensure that the model can achieve the optimal performance on different data sets. Finally, a new intelligent distribution network identification model (Intelligent Distribution Electricity Network Identification System, IDENIS) was constructed. RESULTS: The study was experimentally verified on the distribution system of IEEE 33 and PG&E 69. The experimental results showed that the accuracy of the DNN-based model reached 0.9817 on the test set, while the accuracy after feature selection only decreased by 1.3%, and the features decreased by 81.8%. In the PG&E 69 node system, the features were reduced by 85.5%, while the identification accuracy was decreased by only 0.51%. These results demonstrated that the proposed method maintained high identification accuracy while reducing the computational resource consumption. CONCLUSION: Its efficient computing speed fully meets the real-time requirements in practical applications. This paper provides new ideas and methods for achieving intelligent distribution network topology recognition of high proportion distributed power sources.
Copyright © 2024 Yu Ma, licensed to EAI. This is an open access article distributed under the terms of the CC BY-NC-SA 4.0, which permits copying, redistributing, remixing, transformation, and building upon the material in any medium so long as the original work is properly cited.