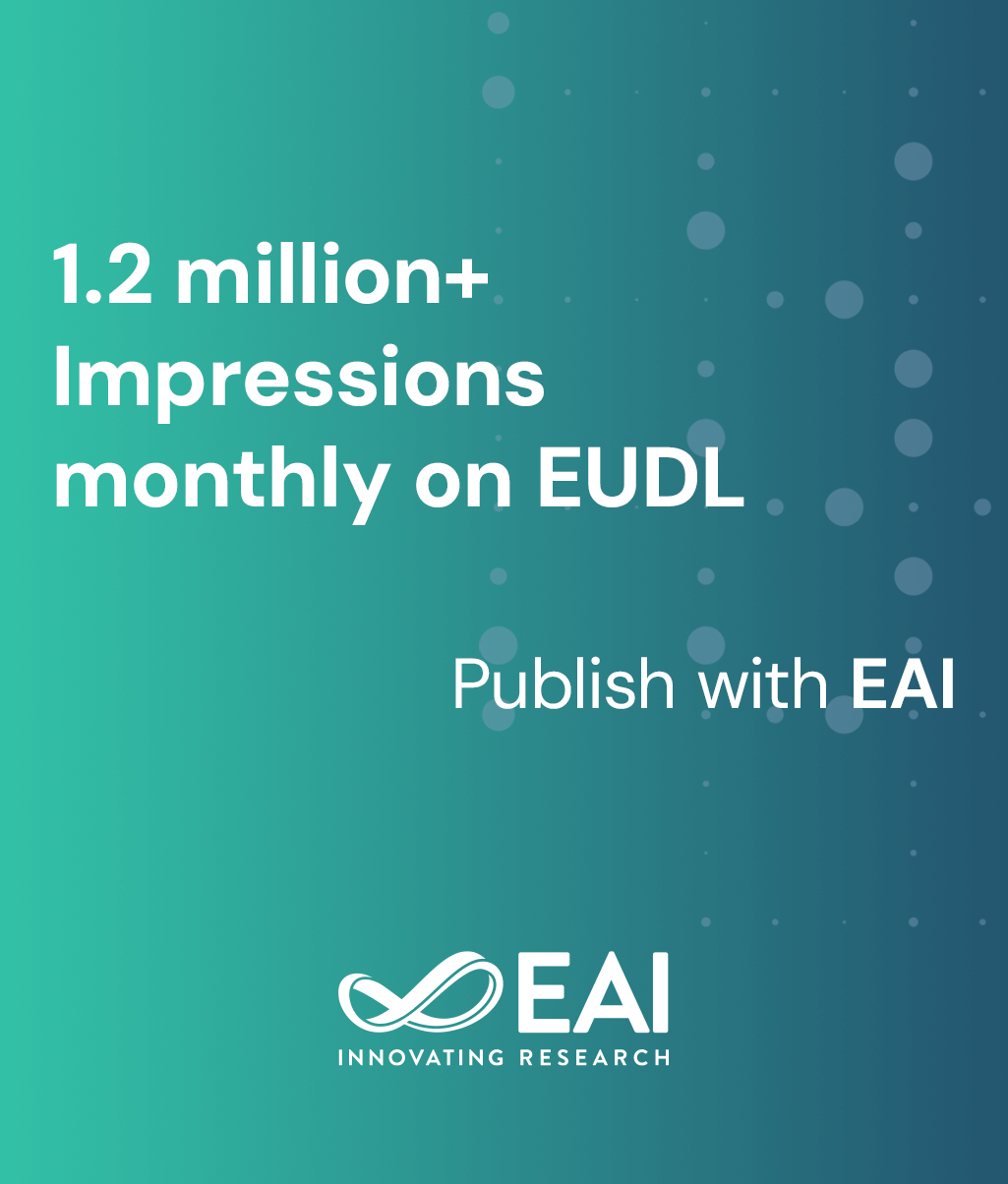
Research Article
Combining Lexical, Host, and Content-based features for Phishing Websites detection using Machine Learning Models
@ARTICLE{10.4108/eetsis.4421, author={Samiya Hamadouche and Ouadjih Boudraa and Mohamed Gasmi}, title={Combining Lexical, Host, and Content-based features for Phishing Websites detection using Machine Learning Models}, journal={EAI Endorsed Transactions on Scalable Information Systems}, volume={11}, number={6}, publisher={EAI}, journal_a={SIS}, year={2024}, month={4}, keywords={Phishing URLs detection, Machine learning algorithms, Classification, Lexical-based features, Host-based features, content-based features, Feature selection}, doi={10.4108/eetsis.4421} }
- Samiya Hamadouche
Ouadjih Boudraa
Mohamed Gasmi
Year: 2024
Combining Lexical, Host, and Content-based features for Phishing Websites detection using Machine Learning Models
SIS
EAI
DOI: 10.4108/eetsis.4421
Abstract
In cybersecurity field, identifying and dealing with threats from malicious websites (phishing, spam, and drive-by downloads, for example) is a major concern for the community. Consequently, the need for effective detection methods has become a necessity. Recent advances in Machine Learning (ML) have renewed interest in its application to a variety of cybersecurity challenges. When it comes to detecting phishing URLs, machine learning relies on specific attributes, such as lexical, host, and content based features. The main objective of our work is to propose, implement and evaluate a solution for identifying phishing URLs based on a combination of these feature sets. This paper focuses on using a new balanced dataset, extracting useful features from it, and selecting the optimal features using different feature selection techniques to build and conduct a comparative performance evaluation of four ML models (SVM, Decision Tree, Random Forest, and XGBoost). Results showed that the XGBoost model outperformed the others models, with an accuracy of 95.70% and a false negatives rate of 1.94%.
Copyright © 2024 S. Hamadouche et al., licensed to EAI. This is an open access article distributed under the terms of the Creative Commons Attribution license (http://creativecommons.org/licenses/by/3.0/), which permits unlimited use, distribution and reproduction in any medium so long as the original work is properly cited.