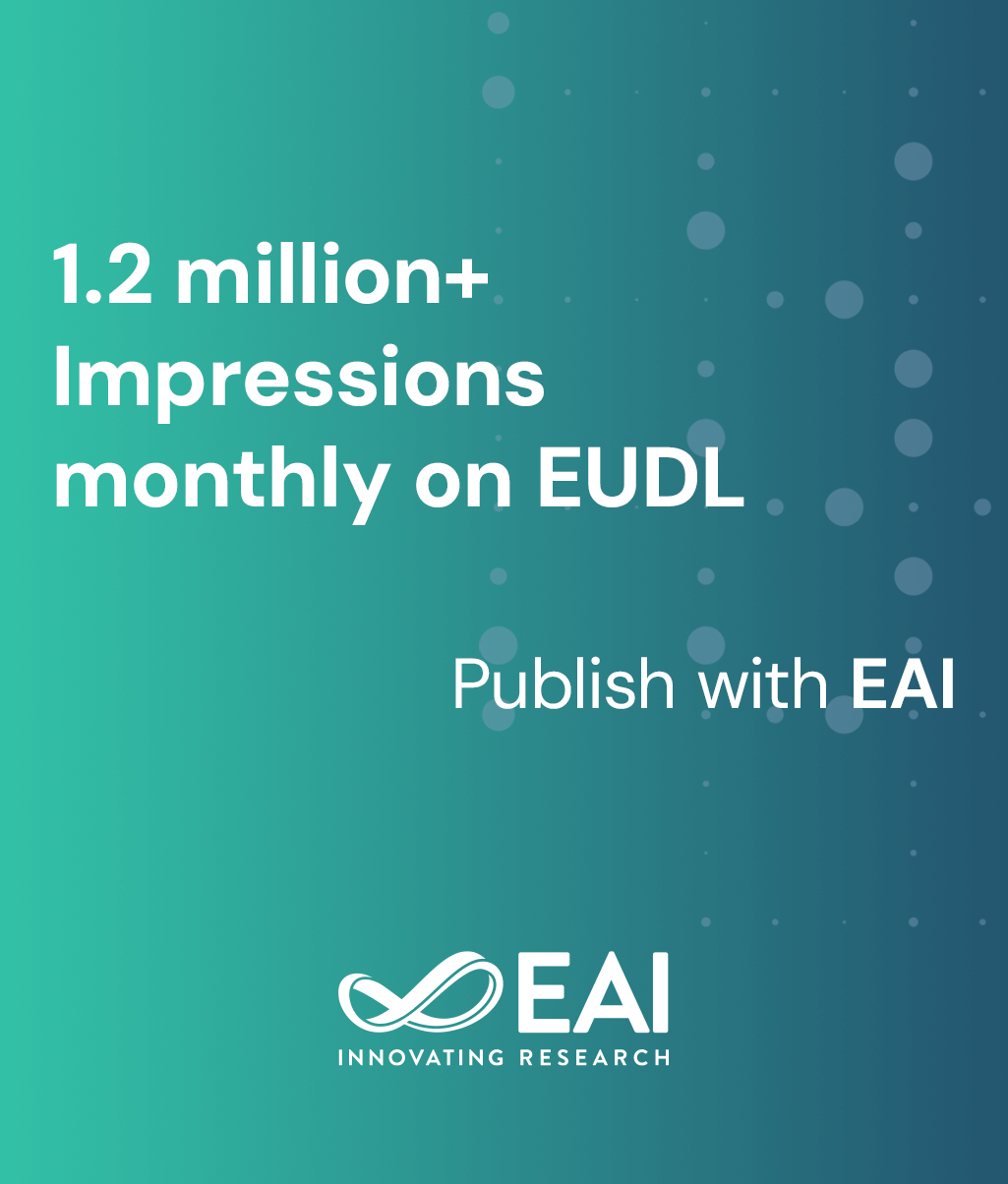
Research Article
Deep Model Training and Deployment in Heterogeneous IoT Networks
@ARTICLE{10.4108/eetmca.v7i3.2899, author={Bowen Lu and Shiwei Lai and Yajuan Tang and Tao Cui and Chengyuan Fan and Jianghong Ou and Dahua Fan}, title={Deep Model Training and Deployment in Heterogeneous IoT Networks}, journal={EAI Endorsed Transactions on Mobile Communications and Applications}, volume={7}, number={3}, publisher={EAI}, journal_a={MCA}, year={2023}, month={1}, keywords={Deep learning, deep model training, deep model deployment}, doi={10.4108/eetmca.v7i3.2899} }
- Bowen Lu
Shiwei Lai
Yajuan Tang
Tao Cui
Chengyuan Fan
Jianghong Ou
Dahua Fan
Year: 2023
Deep Model Training and Deployment in Heterogeneous IoT Networks
MCA
EAI
DOI: 10.4108/eetmca.v7i3.2899
Abstract
As a typical form of machines learning, deep learning has attracted much attention from researchers. It can independently construct (train) basic rules according to the sample data in the learning process. Especially in the field of machine vision, neural networks are usually trained by supervised learning, that is, by example data and predefined results of example data. In this paper, we firstly overview the current research progress on the deep model training and deployment on the heterogeneous Internet of Things (IoT) networks, by taking into account both the latency and energy consumption from various devices in the system. We then summarize the existing challenges on the model training and model deployment on the heterogeneous IoT devices. We further give some feasible solutions to solve the challenges on the model training and model deployment on the heterogeneous IoT devices. The study in this paper can serve as an important reference for the development of deep model training and model deployment for heterogeneous IoT networks.
Copyright © 2023 Bowen Lu et al., licensed to EAI. This is an open access article distributed under the terms of the CC BY-NC-SA 4.0, which permits copying, redistributing, remixing, transformation, and building upon the material in any medium so long as the original work is properly cited.