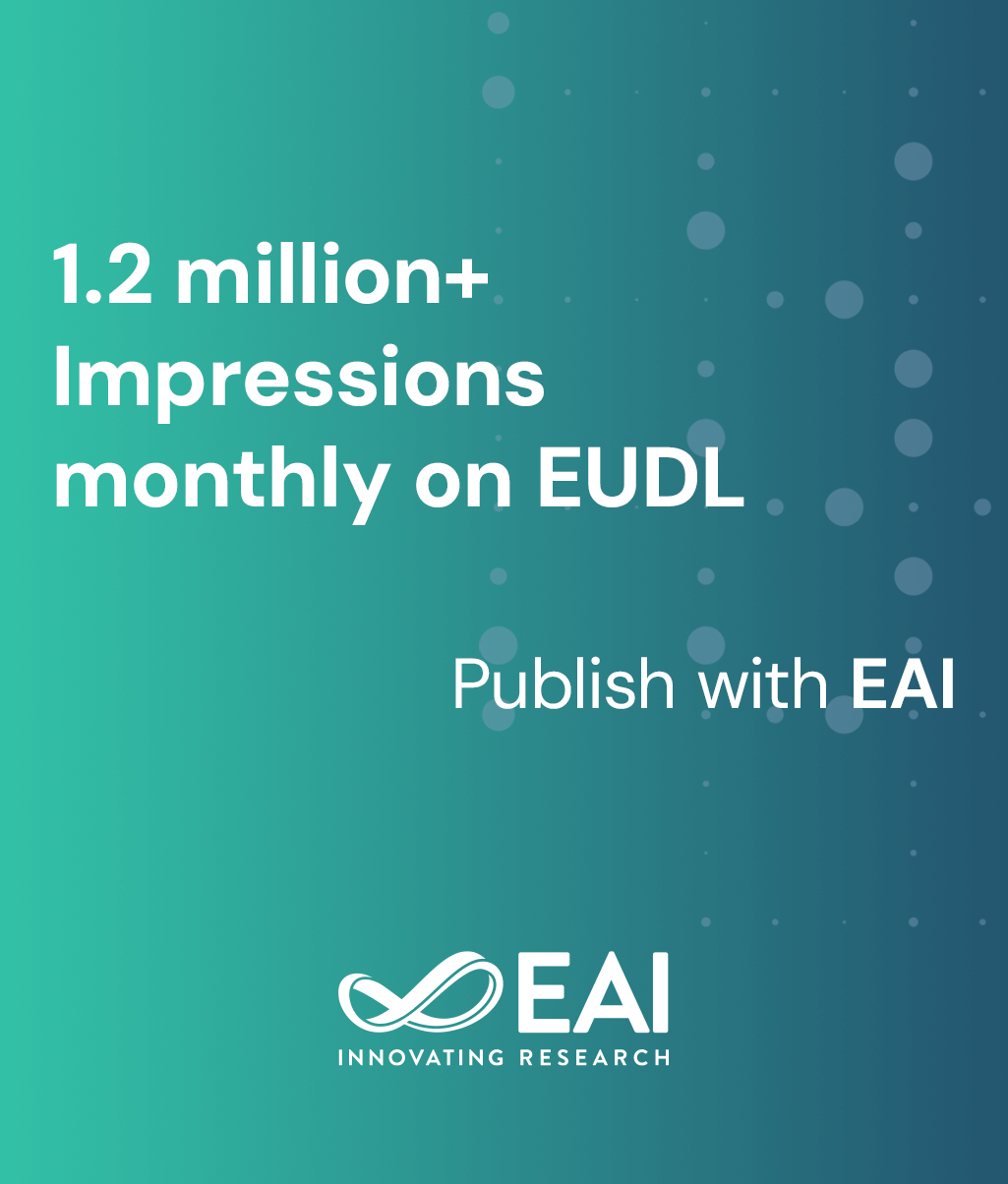
Research Article
Weed detection with Improved Yolov 7
@ARTICLE{10.4108/eetiot.v9i3.3468, author={Mingkang Peng and Wuping Zhang and Fuzhong Li and Qiyuan Xue and Jialiang Yuan and Peipu An}, title={Weed detection with Improved Yolov 7}, journal={EAI Endorsed Transactions on Internet of Things}, volume={9}, number={3}, publisher={EAI}, journal_a={IOT}, year={2023}, month={7}, keywords={weed identification, Deep Learning, attention mechanism, yolov7}, doi={10.4108/eetiot.v9i3.3468} }
- Mingkang Peng
Wuping Zhang
Fuzhong Li
Qiyuan Xue
Jialiang Yuan
Peipu An
Year: 2023
Weed detection with Improved Yolov 7
IOT
EAI
DOI: 10.4108/eetiot.v9i3.3468
Abstract
INTRODUCTION: An improved Yolo v7 model. OBJECTIVES: To solve the weed detection and identification in complex field background. METHODS: The dataset was enhanced by online data enhancement, in which the feature extraction, feature fusion and feature point judgment of weed image were carried out by Yolov7 to predict the weed situation corresponding to the prior box. In the enhanced feature extraction part of Yolov7, CBAM, an attention mechanism combining channel and space, is introduced to improve the attention of the algorithm to weeds and strengthen the characteristics of weeds. RESULTS: The mean average precision (mAP ) of the improved algorithm reached 91.15%, which was 2.06% higher than that of the original Yolov7 algorithm. Compared with the current mainstream target detection algorithms Yolox, Yolov5l, Fster RCNN, Yolov4-tiny and Yolov3, the mAP value of the improved algorithm increased by 4.35, 4.51, 5.41, 19.77 and 20.65 percentage points. Weed species can be accurately identified when multiple weeds are adjacent. CONCLUSION: This paper provides a detection model based on Yolov7 for weed detection in the field, which has a good detection effect on weed detection, and lays a research foundation for intelligent weeding robot and spraying robot.
Copyright © 2023 Peng et al., licensed to EAI. This is an open access article distributed under the terms of the CC BY-NC-SA 4.0, which permits copying, redistributing, remixing, transformation, and building upon the material in any medium so long as the original work is properly cited.