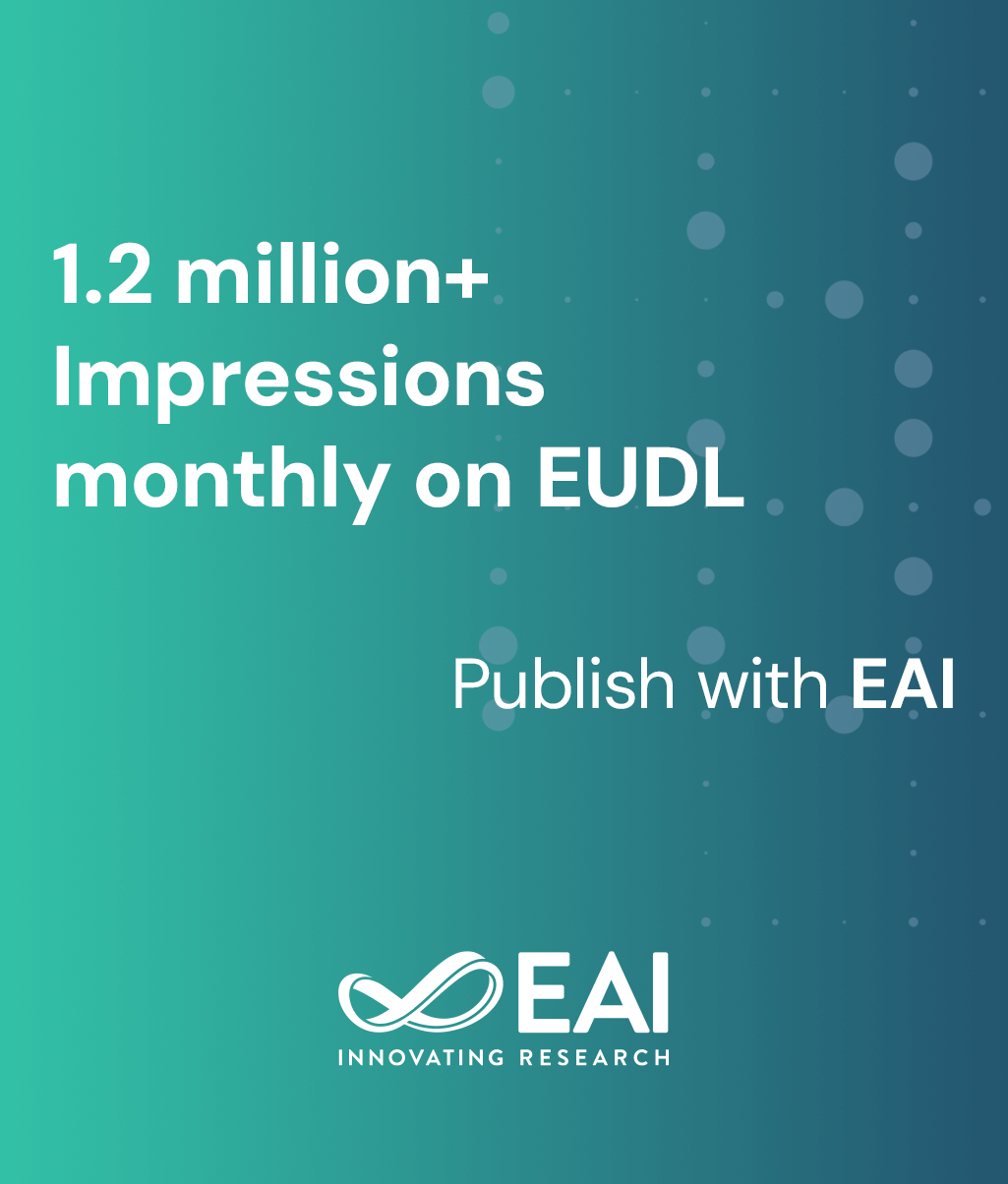
Research Article
Synergistic Integration of Quantum and Classical Machine Learning Models for High-Fidelity Asteroid Hazard Detection
@ARTICLE{10.4108/eetiot.8170, author={Kuldeep Vayadande and Yogesh Bodhe and Amol A. Bhosle and Kavita Sultanpure and Geetanjali Yadav and Ajit R. Patil and Jyoti Jayesh Chavhan and Amolkumar N. Jadhav and Preeti Bailke}, title={Synergistic Integration of Quantum and Classical Machine Learning Models for High-Fidelity Asteroid Hazard Detection}, journal={EAI Endorsed Transactions on Internet of Things}, volume={11}, number={1}, publisher={EAI}, journal_a={IOT}, year={2025}, month={4}, keywords={Quantum machine learning, Quantum computing, Potentially Hazardous Asteroids (PHAs), Quantum Convolutional Neural Networks (QCNN), Space Hazard Assessment, quantum algorithm optimization, Recursive Feature Elimination(RFE)}, doi={10.4108/eetiot.8170} }
- Kuldeep Vayadande
Yogesh Bodhe
Amol A. Bhosle
Kavita Sultanpure
Geetanjali Yadav
Ajit R. Patil
Jyoti Jayesh Chavhan
Amolkumar N. Jadhav
Preeti Bailke
Year: 2025
Synergistic Integration of Quantum and Classical Machine Learning Models for High-Fidelity Asteroid Hazard Detection
IOT
EAI
DOI: 10.4108/eetiot.8170
Abstract
This research investigates the use of quantum machine learning (QML) to classify asteroids into non-hazardous and hazardous groups, which yields successful results in detecting the hazard. In addition to the complexity involved in analyzing orbits and physical objects, QML performs better than traditional machine learning in modeling the relationship between data. This method involves data-intensive preprocessing steps, such as feature selection by removing unnecessary features and correlation analysis to find predictors. Quantum circuits are used for specification and classification, and the standard evaluation is based on accuracy, recall, F1 score, and precision. A strong and weak method is provided by cross-validation and hyperparameter tuning. The best classical model here is the decision tree, which is a good model for high-resolution and low-budget social benefit with 0.883 accuracy, 0.955 recovery rate, 0.981 F1 score, and 0.883 sensitivity. However, the quantum model has made great leaps. AMSGRAD QCNN (Adaptive Moment Estimation with Gradient Thresholding Quantum Convolutional Neural Networks) achieves a non-uniform accuracy of 0.997, which is 13% higher than the decision tree with 0.984 accuracy, 0.955 recovery rate, and 0.981 F1 score. The accuracy of SPSA QCNN(Simultaneous Perturbation Stochastic Approximation) is 0.993, the recall is 0.974, and the F1 score is 0.977. This improvement shows the excellent ability of the quantum model to better resolve correlation data and, more importantly, to reduce false negatives in detecting stars. These results demonstrate the ability of quantum computing to analyze complex data and provide the best results. Our future work will focus on identifying and repairing quantum circuits, as well as exploring hybrid quantum classical models to improve model accuracy and interpretation. The findings open the door to new ways to predict the simplest solutions and the most powerful and accurate way to date of estimating the danger zone.
Copyright © 2025 K. Vayadande et al., licensed to EAI. This is an open access article distributed under the terms of the CC BY-NC-SA 4.0, which permits copying, redistributing, remixing, transformation, and building upon the material in any medium so long as the original work is properly cited.