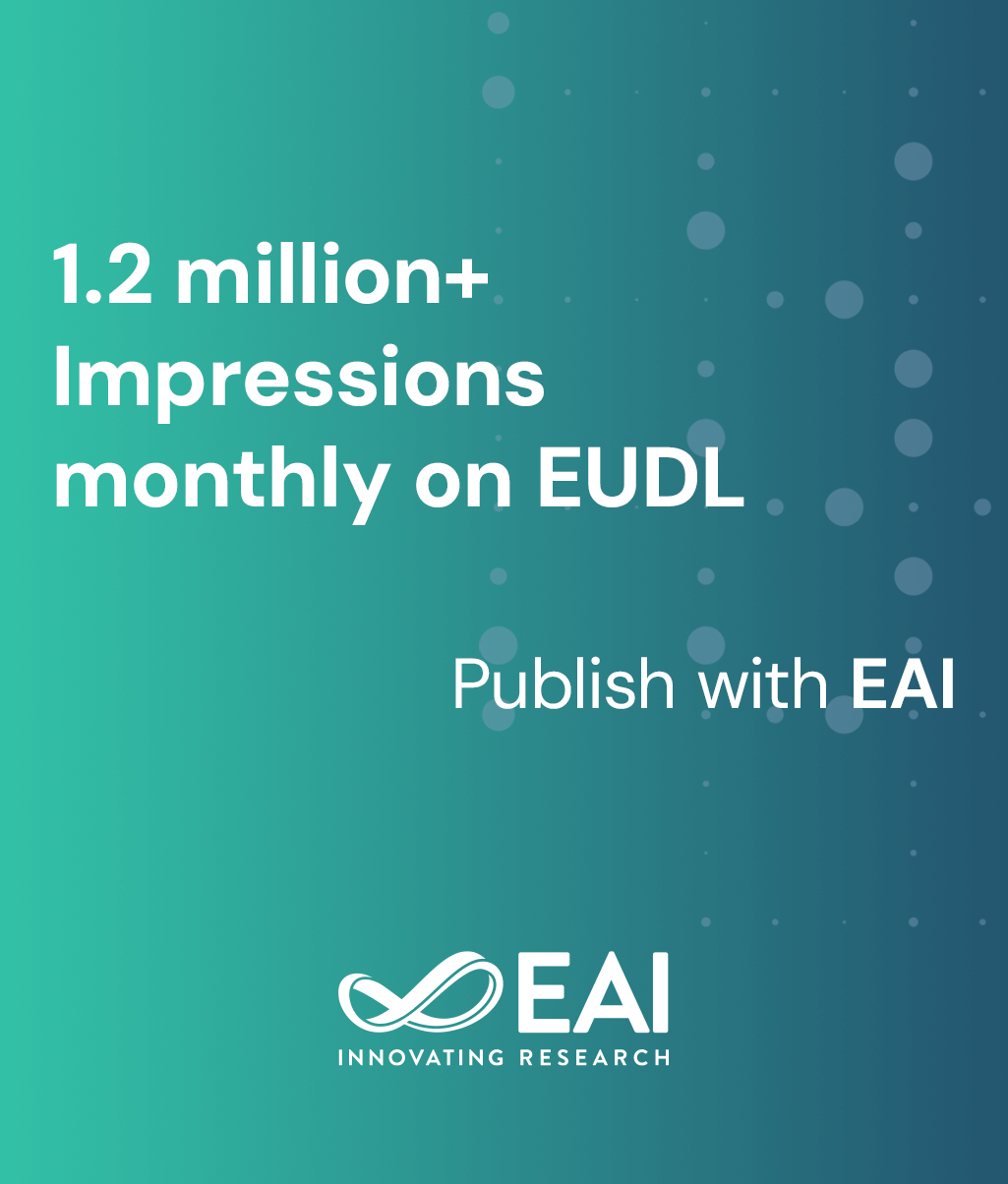
Research Article
Design Of Intelligent Road Eye Using AI And Machine Learning For Automobiles
@ARTICLE{10.4108/eetiot.7638, author={Chandrasekharan Nataraj and Lawrence Wong Ming Wei and Mukil Alagirisamy and Sathish Kumar Selvaperumal}, title={Design Of Intelligent Road Eye Using AI And Machine Learning For Automobiles}, journal={EAI Endorsed Transactions on Internet of Things}, volume={11}, number={1}, publisher={EAI}, journal_a={IOT}, year={2025}, month={4}, keywords={Artificial Intelligence, Image processing, object detection, Machine Learning, Road safety, Vehicle safety}, doi={10.4108/eetiot.7638} }
- Chandrasekharan Nataraj
Lawrence Wong Ming Wei
Mukil Alagirisamy
Sathish Kumar Selvaperumal
Year: 2025
Design Of Intelligent Road Eye Using AI And Machine Learning For Automobiles
IOT
EAI
DOI: 10.4108/eetiot.7638
Abstract
The project aims to design an intelligent road eye by using AI and a machine learning approach to detect speedbumps and potholes on the road. The system design utilizes a YOLOv5 custom-trained model and COCO dataset in detecting the objects on the road. The system is integrated with lane detection algorithms to achieve active steering feedback and pothole avoidance. Based on the detection results, feedback will be given in the form of visual, audio, and steering angles, allowing the driver to have sufficient response time to perform braking or steering adjustments where applicable. The trained model can achieve a mean average precision value (mAP) of up to 0.995 for all classes, and a maximum detection range of 5.77m and 34.8m for potholes and speedbump respectively. The future works of the project include integrating the algorithm into the vehicle to achieve autonomous braking and active pothole avoidance with the help of sensors and cameras on the vehicle, as well as adopting augmented reality (AR) to project the visual feedback on the vehicle windscreen.
Copyright © 2025 Ch. Nataraj et al., licensed to EAI. This is an open access article distributed under the terms of the CC BY-NC-SA 4.0, which permits copying, redistributing, remixing, transformation, and building upon the material in any medium so long as the original work is properly cited.