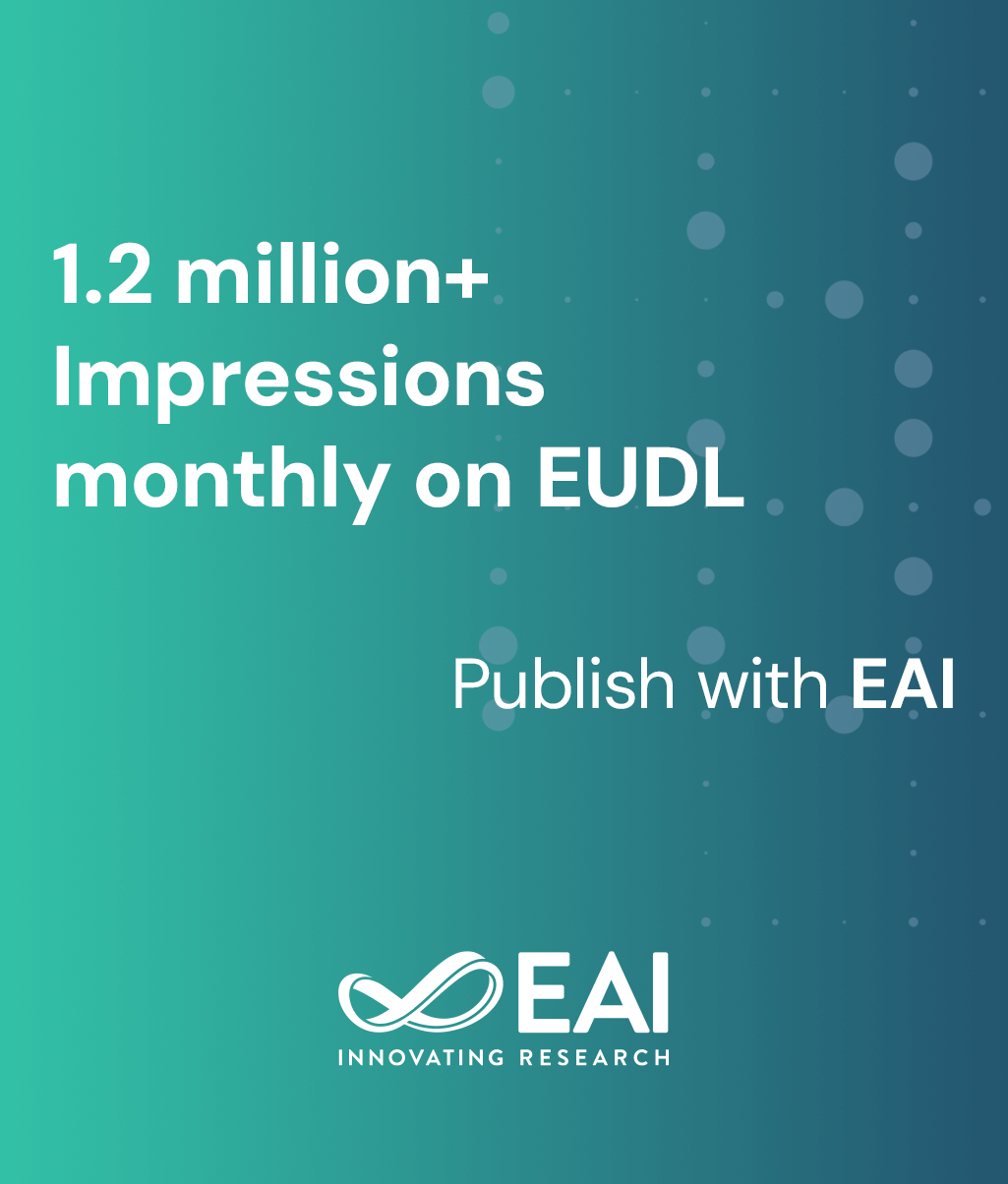
Research Article
Analysis of Current Advancement in 3D Point Cloud Semantic Segmentation
@ARTICLE{10.4108/eetiot.4495, author={Koneru Pranav Sai and Sagar Dhanraj Pande}, title={Analysis of Current Advancement in 3D Point Cloud Semantic Segmentation}, journal={EAI Endorsed Transactions on Internet of Things}, volume={10}, number={1}, publisher={EAI}, journal_a={IOT}, year={2023}, month={11}, keywords={Point cloud, Semantic segmentation, Datasets, Deep learning}, doi={10.4108/eetiot.4495} }
- Koneru Pranav Sai
Sagar Dhanraj Pande
Year: 2023
Analysis of Current Advancement in 3D Point Cloud Semantic Segmentation
IOT
EAI
DOI: 10.4108/eetiot.4495
Abstract
INTRODUCTION: The division of a 3D point cloud into various meaningful regions or objects is known as point cloud segmentation. OBJECTIVES: The paper discusses the challenges faced in 3D point cloud segmentation, such as the high dimensionality of point cloud data, noise, and varying point densities. METHODS: The paper compares several commonly used datasets in the field, including the ModelNet, ScanNet, S3DIS, and Semantic 3D datasets, ApploloCar3D, and provides an analysis of the strengths and weaknesses of each dataset. Also provides an overview of the papers that uses Traditional clustering techniques, deep learning-based methods, and hybrid approaches in point cloud semantic segmentation. The report also discusses the benefits and drawbacks of each approach. CONCLUSION: This study sheds light on the state of the art in semantic segmentation of 3D point clouds.
Copyright © 2023K. P. Sai et al., licensed to EAI. This is an open access article distributed under the terms of the CC BY-NC-SA 4.0, which permits copying, redistributing, remixing, transformation, and building upon the material in any medium so long as the original work is properly cited.