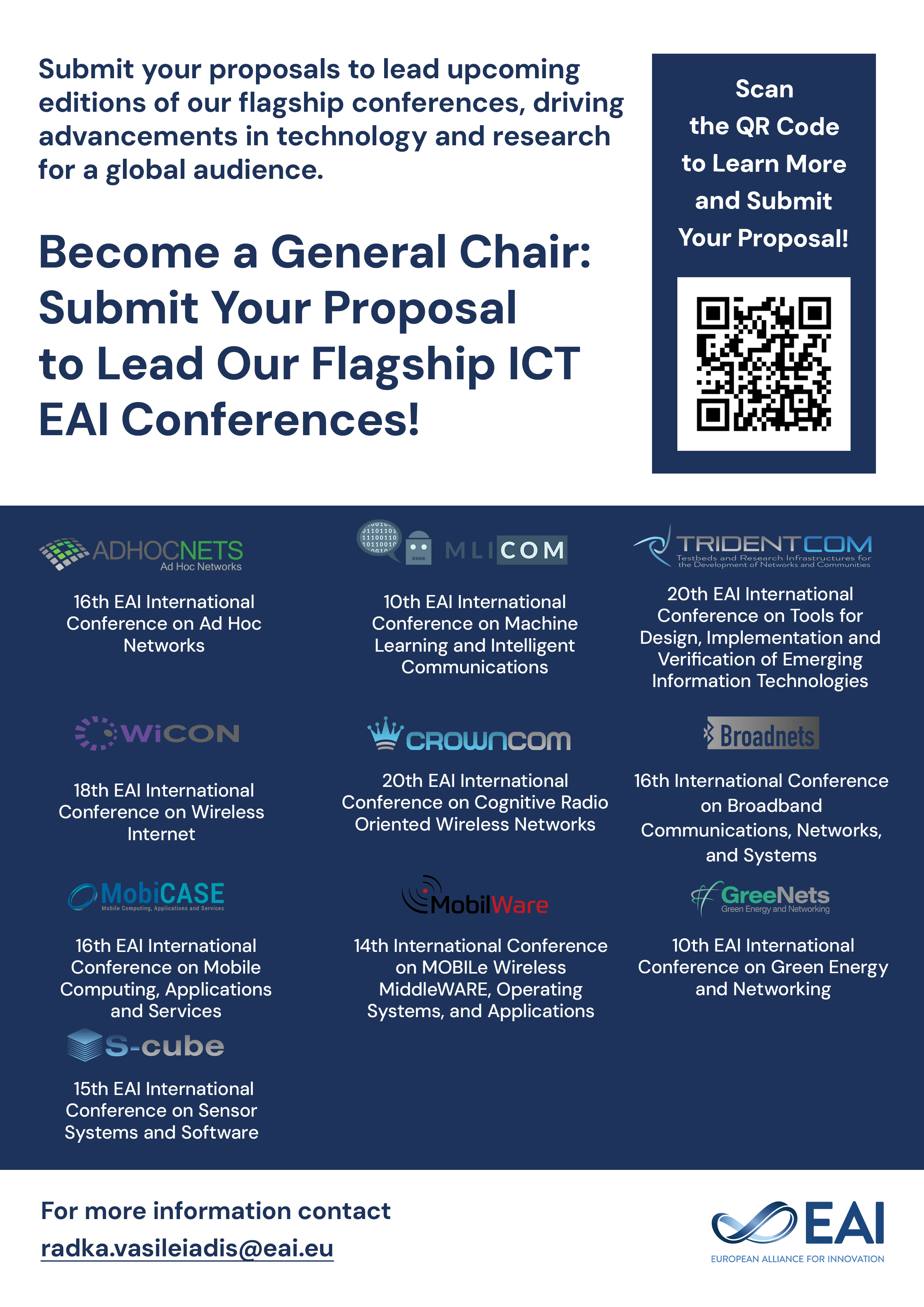
Research Article
Evaluation Study of the Chi-Square Method for Feature Selection in Stroke Prediction with Random Forest Regression
@INPROCEEDINGS{10.4108/eai.30-10-2023.2343096, author={Nurliana Nasution and Feldiansyah Bakri Nasution and Erlin Erlin and Mhd Arief Hasan}, title={Evaluation Study of the Chi-Square Method for Feature Selection in Stroke Prediction with Random Forest Regression}, proceedings={Proceedings of the 2nd International Conference on Environmental, Energy, and Earth Science, ICEEES 2023, 30 October 2023, Pekanbaru, Indonesia}, publisher={EAI}, proceedings_a={ICEEES}, year={2024}, month={4}, keywords={stroke chi-square random forest regression classification early detection}, doi={10.4108/eai.30-10-2023.2343096} }
- Nurliana Nasution
Feldiansyah Bakri Nasution
Erlin Erlin
Mhd Arief Hasan
Year: 2024
Evaluation Study of the Chi-Square Method for Feature Selection in Stroke Prediction with Random Forest Regression
ICEEES
EAI
DOI: 10.4108/eai.30-10-2023.2343096
Abstract
This study aims to develop a more accurate classification model for diagnosing Stroke cases based on various clinical features. Stroke is a serious global health issue, and early detection has a positive impact on prognosis and the prevention of complications. In this research, we combine two main approaches, feature selection using the Chi-Square statistical test and the implementation of Random Forest Regression, to enhance the accuracy of Stroke diagnosis.First, we use the Chi-Square test to evaluate the relationship between categorical variables (such as gender, smoking history, marital status, and others) and Stroke status. The results of this test are used to select variables that have a significant association with Stroke. In addition to accuracy, we also observe improvements in precision, recall, and F1-Score, which indicate the model's ability to identify Stroke cases and avoid misdiagnoses.The findings of this research hold significant potential in clinical practice, particularly in the early detection and management of Stroke. Early Stroke detection can lead to faster intervention, ultimately reducing the negative impact of Stroke on patients. We hope that this study will serve as a foundation for the development of more advanced and accurate models for Stroke diagnosis, contributing to overall public healthcare improvement.