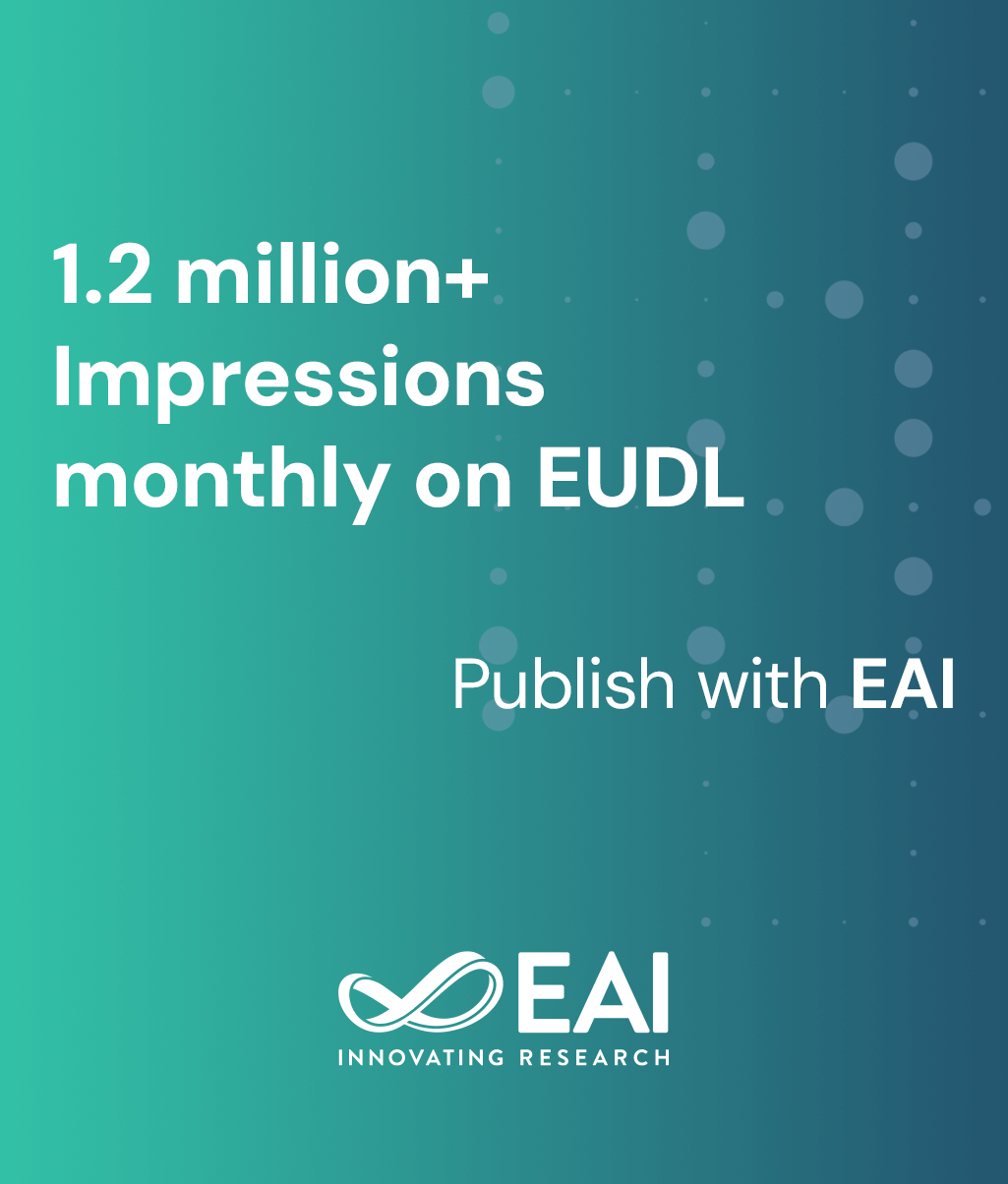
Research Article
Transmission Policies for Energy Harvesting Sensors Based on Markov Chain Energy Supply
@ARTICLE{10.4108/eai.28-9-2015.2261406, author={Wenxiang Zhu and Pingping Xu and Maozong Zheng and Guilu Wu and Honglei Wang}, title={Transmission Policies for Energy Harvesting Sensors Based on Markov Chain Energy Supply}, journal={EAI Endorsed Transactions on Energy Web}, volume={3}, number={8}, publisher={ACM}, journal_a={EW}, year={2015}, month={12}, keywords={energy harvesting, wireless sensor networks, energy management, markov decision process}, doi={10.4108/eai.28-9-2015.2261406} }
- Wenxiang Zhu
Pingping Xu
Maozong Zheng
Guilu Wu
Honglei Wang
Year: 2015
Transmission Policies for Energy Harvesting Sensors Based on Markov Chain Energy Supply
EW
EAI
DOI: 10.4108/eai.28-9-2015.2261406
Abstract
Due to the small energy harvesting rates and stochastic energy harvesting processes, energy management of energy harvesting senor is still crucial for body network. Transmission polices for energy harvesting sensors with Markov chain energy supply over time varying channels is formulated as an infinite discounted reward Markov Decision Problem under the assumption of geometric distribution of sensors’ lifetime. In this paper, we firstly propose a low-storage transmission policy based on probability of successful transmission for body network. Then we narrow the feasible region of parameters in our policies from the real domain to a discrete set with limited number, which makes the method of combing optimal equations and enumeration algorithm to obtain optimal parameters workable. Finally, numerical results show that our presented transmission policies can achieve a good approximated performance of optimal policies, which can be derived by policy iteration algorithm. Compared with the optimal policies, our presented policies has the advantage of low storage.
Copyright © 2015 W. Zhu et al., licensed to EAI. This is an open access article distributed under the terms of the Creative Commons Attribution licence (http://creativecommons.org/licenses/by/3.0/), which permits unlimited use, distribution and reproduction in any medium so long as the original work is properly cited.