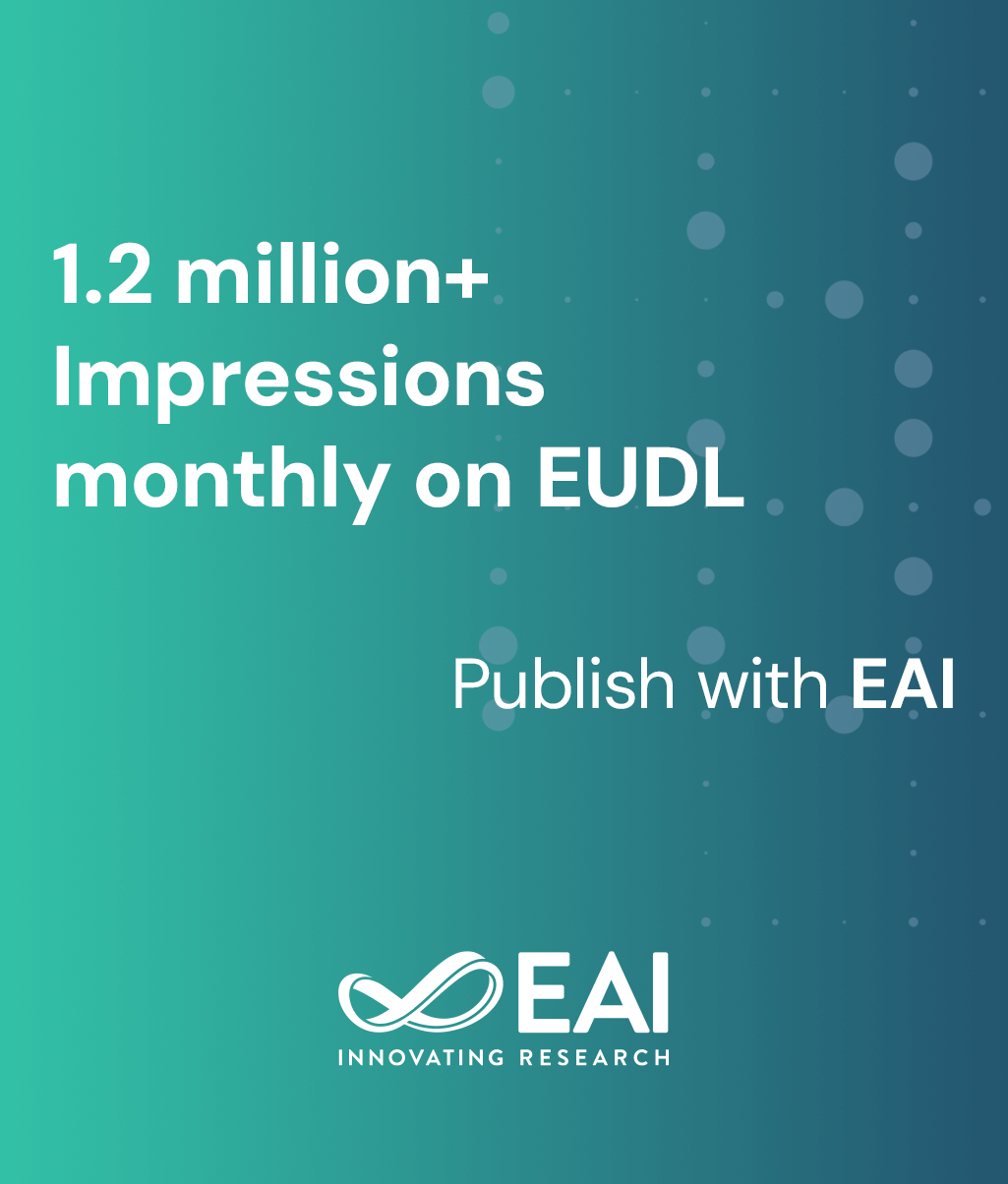
Research Article
Mobeacon: An iBeacon-Assisted Smartphone-Based Real Time Activity Recognition Framework
@ARTICLE{10.4108/eai.22-7-2015.2260073, author={Mohammad Arif Ul Alam and Nilavra Pathak and Nirmalya Roy}, title={Mobeacon: An iBeacon-Assisted Smartphone-Based Real Time Activity Recognition Framework}, journal={EAI Endorsed Transactions on Ubiquitous Environments}, volume={2}, number={7}, publisher={EAI}, journal_a={UE}, year={2015}, month={8}, keywords={activity recognition, adaptive lightweight classification, semantic knowledge, multi-modal sensing system}, doi={10.4108/eai.22-7-2015.2260073} }
- Mohammad Arif Ul Alam
Nilavra Pathak
Nirmalya Roy
Year: 2015
Mobeacon: An iBeacon-Assisted Smartphone-Based Real Time Activity Recognition Framework
UE
EAI
DOI: 10.4108/eai.22-7-2015.2260073
Abstract
Human activity recognition using multi-modal sensing technologies to automatically collect and classify daily activities has become an active field of research. Given the proliferation of smart and wearable devices and their greater acceptance in human lives, the need for developing real time lightweight activity recognition algorithms become a viable and urgent avenue. Although variants of online and offline lightweight activity recognition algorithms have been developed, realizing them on real time to recognize people's activities is still a challenging research problem due to the computational complexity of building, training, learning and storing activity models in resource constrained smart and wearable devices. To navigate the above challenges, we build Mobeacon: a mobile phone and iBeacon sensor-based smart home activity recognition system. We investigated the viability of extending Bagging Ensemble Learning (BEL) and Packaged Naive Bayes (PNB) classification algorithms for high-level activity recognition on smartphone. We incorporated the semantic knowledge of the testing environment and used that with the built-in adaptive learning models on smartphone to ease the ground truth data annotation. We demonstrated that Mobeacon outperforms existing lightweight activity recognition techniques in terms of accuracy (max. 94%) in a low resource setting and proves itself substantially efficient to reside on smartphones for recognizing ADLs in real time.
Copyright © 2015 M. Arif Ul Alam et al., licensed to EAI. This is an open access article distributed under the terms of the Creative Commons Attribution licence (http://creativecommons.org/licenses/by/3.0/), which permits unlimited use, distribution and reproduction in any medium so long as the original work is properly cited.