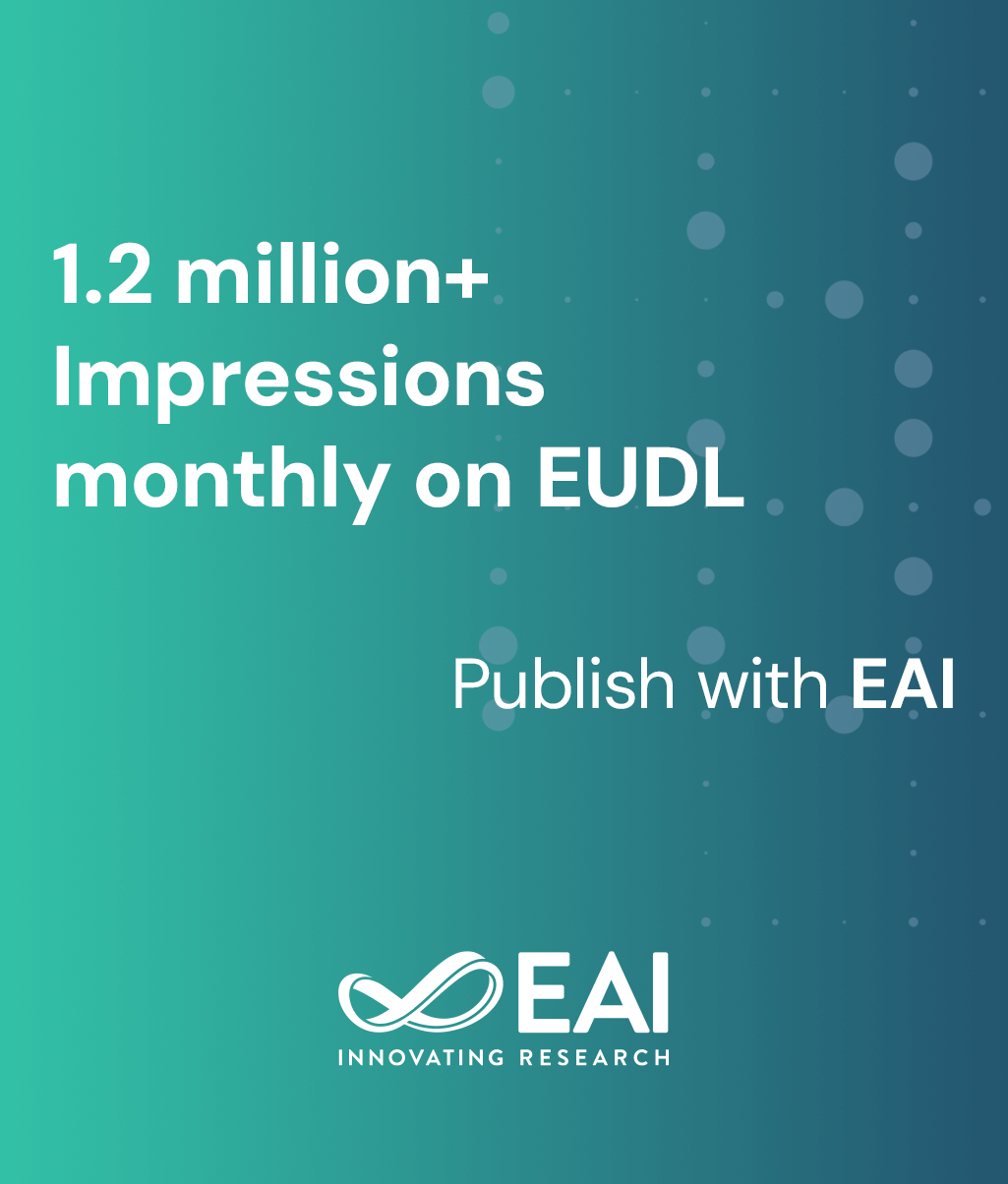
Research Article
Application of FMCW Radar for Dynamic Continuous Hand Gesture Recognition
@INPROCEEDINGS{10.4108/eai.21-6-2018.2276537, author={Zhenyuan Zhang and Zengshan Tian and Zhou Mu and Yi Liu}, title={Application of FMCW Radar for Dynamic Continuous Hand Gesture Recognition}, proceedings={International Workshop on Millimeter-wave Radar Technology and Application}, publisher={EAI}, proceedings_a={IWMRTA}, year={2018}, month={9}, keywords={hand gesture recognition fmcw radar recurrent three-dimensional convolutional neural network connectionist temporal classification}, doi={10.4108/eai.21-6-2018.2276537} }
- Zhenyuan Zhang
Zengshan Tian
Zhou Mu
Yi Liu
Year: 2018
Application of FMCW Radar for Dynamic Continuous Hand Gesture Recognition
IWMRTA
EAI
DOI: 10.4108/eai.21-6-2018.2276537
Abstract
Recently, hand gesture recognition systems have become increasingly interesting to researchers in the field of human-computer interfaces. Real-world systems for human dynamic hand gesture recognition is challenging as: 1) the system must be robustness to various conditions; 2) there is a rich diversity in how people perform hand gestures, making hand gesture recognition difficult; 3) the system must detect and recognize hand gestures continuously using unsegmented input streams in order to avoid noticeable lag between performing a gesture and its classification. In this paper, to address these challenges, we present a novel system for dynamic continuous hand gesture recognition based on Frequency Modulated Continuous Wave (FMCW) radar sensor. The radar system does not depend on lighting, noise or atmospheric conditions. We employ a recurrent three-dimensional convolutional neural network to perform classification of dynamic hand gestures. To enhance the processing performance, Connectionist Temporal Classification (CTC) algorithm is used to train the network to predict class labels from inprogress gestures in unsegmented input streams. The experimental results show that this system is able to achieve high recognition rates of 96%, which is higher than state-of-the-art hand gesture recognition systems. In addition, the conclusion in this work can be used for real-time hand gesture recognition system design.