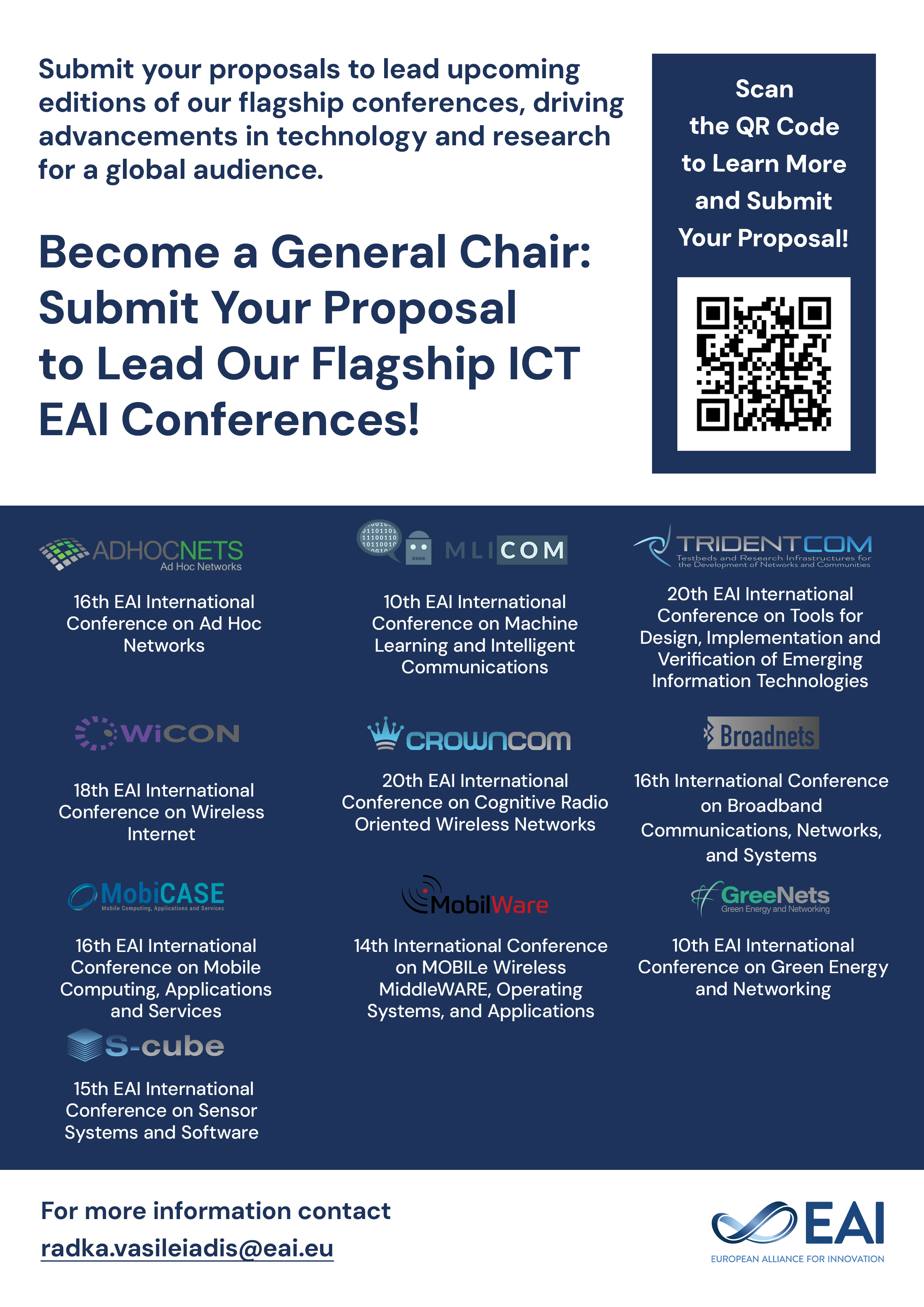
Research Article
The Artificial Neural Networks (ANN) for Batik Detection Based on Textural Features
@INPROCEEDINGS{10.4108/eai.12-10-2019.2296538, author={Anita Ahmad Kasim and Muhammad Bakri and Anindita Septiarini}, title={The Artificial Neural Networks (ANN) for Batik Detection Based on Textural Features}, proceedings={Proceedings of the 7th Mathematics, Science, and Computer Science Education International Seminar, MSCEIS 2019, 12 October 2019, Bandung, West Java, Indonesia}, publisher={EAI}, proceedings_a={MSCEIS}, year={2020}, month={7}, keywords={artificial neural networks (ann) batik detection textural features}, doi={10.4108/eai.12-10-2019.2296538} }
- Anita Ahmad Kasim
Muhammad Bakri
Anindita Septiarini
Year: 2020
The Artificial Neural Networks (ANN) for Batik Detection Based on Textural Features
MSCEIS
EAI
DOI: 10.4108/eai.12-10-2019.2296538
Abstract
This study aims to utilize artificial neural networks to distinguish batik motifs and non-batik fabric motifs. Several important steps are needed, namely the process of acquiring batik and non-batik images, pre-transforming batik and non-batik images to gray scale forms, texture feature extraction in gray scale images and detection of motifs using networks artificial nerve. Image acquisition is done by collecting batik and not batik images from several different motifs. Processing data sets is divided into 70% as training data and 30% as testing data. Artificial neural network models used in this research use the Backpropagation learning algorithm by comparing the Scaled conjugate gradient algorithm (trainscg) training method and the Levenberg-Marquardt algorithm (trainlm) training method. The results obtained for the accuracy using the Scaled conjugate gradient algorithm (trainscg) training method were higher with an accuracy value of 84.12%, compared to the Levenberg-Marquardt algorithm (trainlm) method by 86.11%.