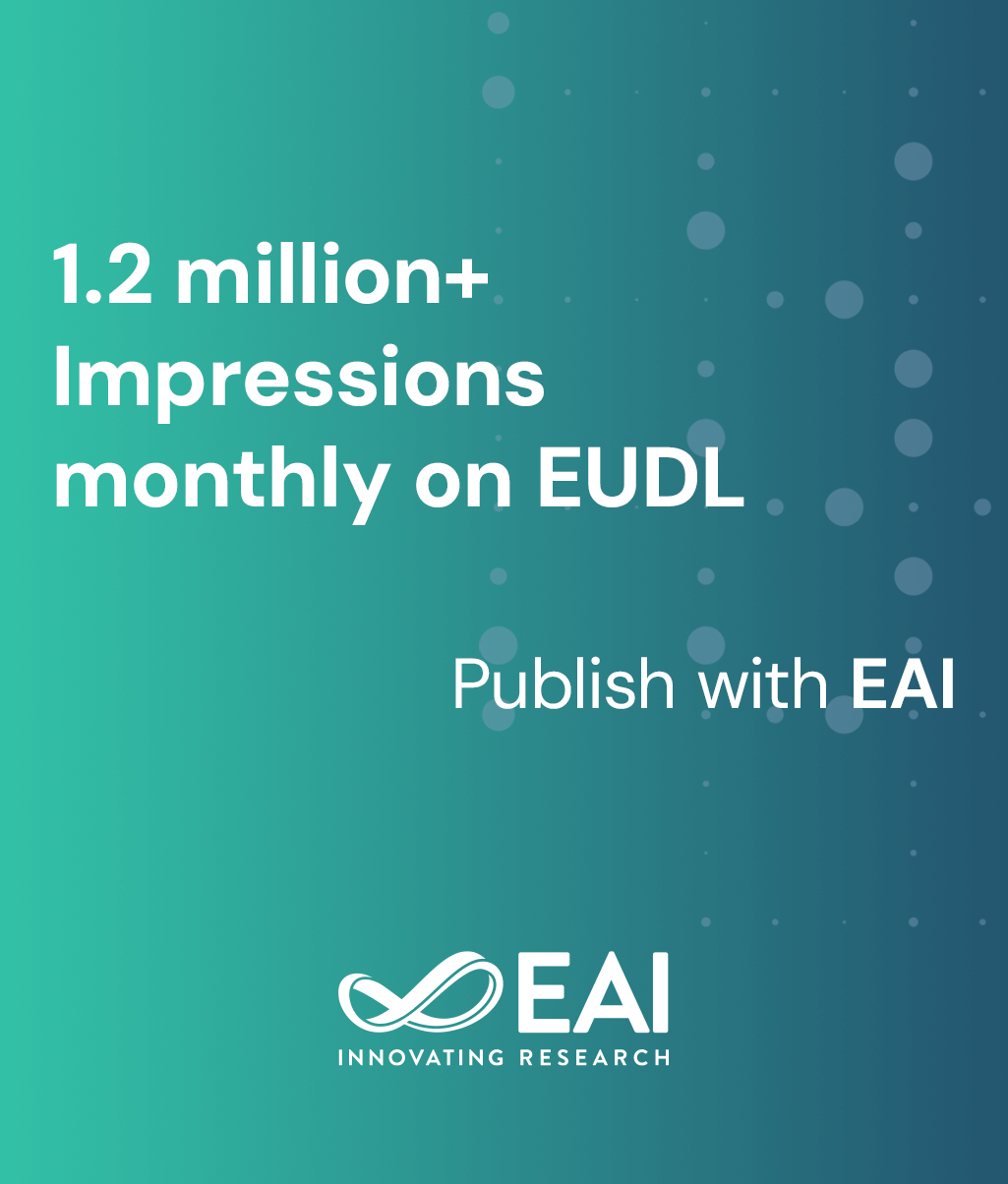
Research Article
Evaluating Unsupervised Fault Detection in Self-Healing Systems Using Stochastic Primitives
@ARTICLE{10.4108/sas.1.1.e3, author={Chris Schneider and Adam Barker and Simon Dobson}, title={Evaluating Unsupervised Fault Detection in Self-Healing Systems Using Stochastic Primitives}, journal={EAI Endorsed Transactions on Self-Adaptive Systems}, volume={1}, number={1}, publisher={ICST}, journal_a={SAS}, year={2015}, month={1}, keywords={Self-healing; Systems; Fault; Anomaly; Detection; Machine Learning; Computational Intelligence; Autonomic Computing; Artificial Neural Networks; Self-Organising Maps; Hidden Markov Models; Restricted Boltzmann Machines; Recurrent Neural Networks.}, doi={10.4108/sas.1.1.e3} }
- Chris Schneider
Adam Barker
Simon Dobson
Year: 2015
Evaluating Unsupervised Fault Detection in Self-Healing Systems Using Stochastic Primitives
SAS
ICST
DOI: 10.4108/sas.1.1.e3
Abstract
Autonomous fault detection represents one approach for reducing operational costs in large-scale computing environments. However, little empirical evidence exists regarding the implementation or comparison of such methodologies, or offers proof that such approaches reduce costs. This paper compares the effectiveness of several types of stochastic primitives using unsupervised learning to heuristically determine the root causes of faults. The results suggest that self-healing systems frameworks leveraging these techniques can reliably and autonomously determine the source of an anomaly within as little as five minutes. This finding lays the foundation for determining the potential these approaches have for reducing operational costs and ultimately concludes with new avenues for exploring anomaly prediction.
Copyright © 2015 C. Schneider et al., licensed to ICST. This is an open access article distributed under the terms of the Creative Commons Attribution licence (http://creativecommons.org/licenses/by/3.0/), which permits unlimited use, distribution and reproduction in any medium so long as the original work is properly cited.