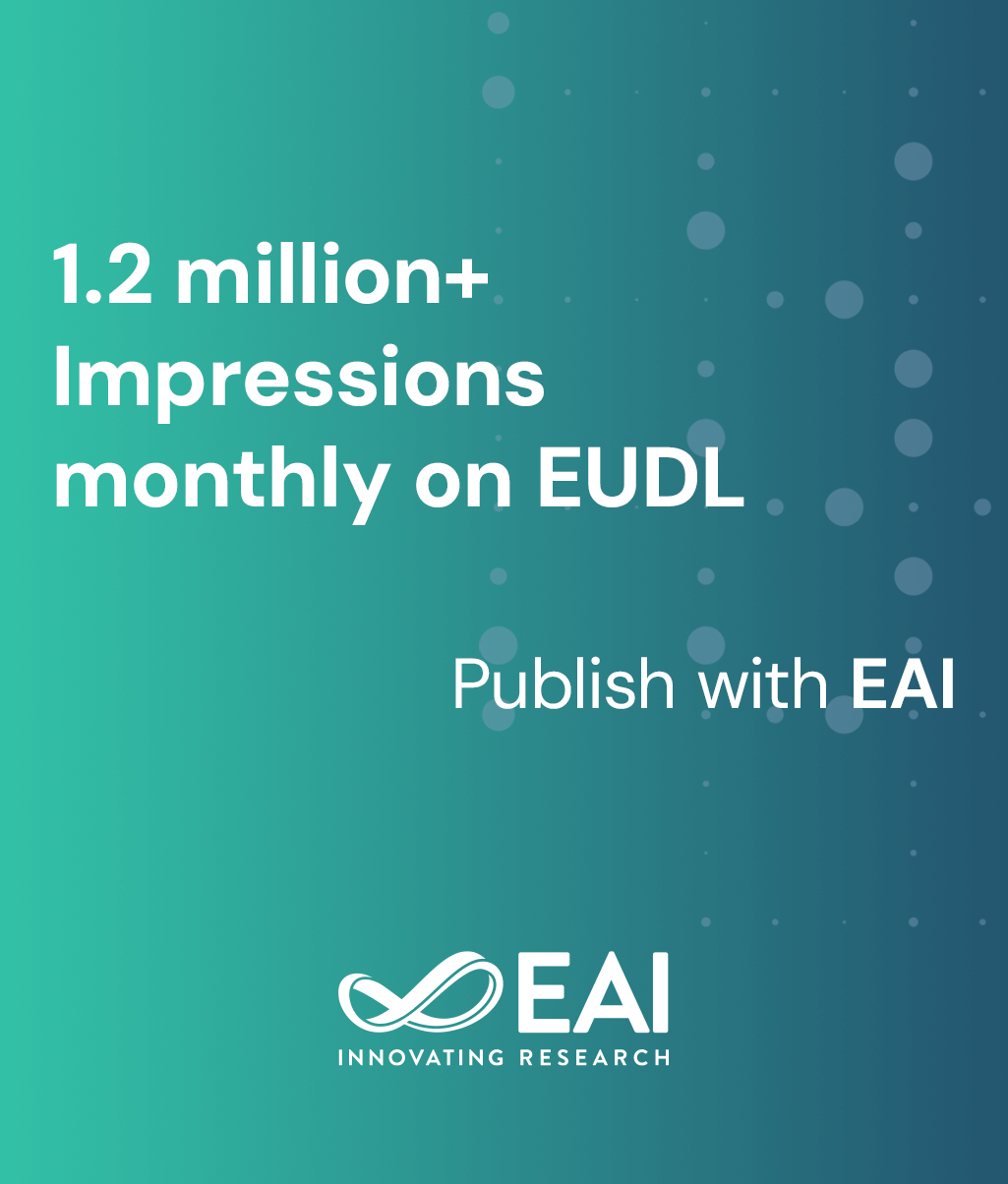
Research Article
An Investigation of Performance Analysis of Anomaly Detection Techniques for Big Data in SCADA Systems
@ARTICLE{10.4108/inis.2.3.e5, author={Mohiuddin Ahmed and Adnan Anwar and Abdun Naser Mahmood and Zubair Shah and Michael J. Maher}, title={An Investigation of Performance Analysis of Anomaly Detection Techniques for Big Data in SCADA Systems}, journal={EAI Endorsed Transactions on Industrial Networks and Intelligent Systems}, volume={2}, number={3}, publisher={ICST}, journal_a={INIS}, year={2015}, month={5}, keywords={Anomaly detection, SCADA systems, big data}, doi={10.4108/inis.2.3.e5} }
- Mohiuddin Ahmed
Adnan Anwar
Abdun Naser Mahmood
Zubair Shah
Michael J. Maher
Year: 2015
An Investigation of Performance Analysis of Anomaly Detection Techniques for Big Data in SCADA Systems
INIS
ICST
DOI: 10.4108/inis.2.3.e5
Abstract
Anomaly detection is an important aspect of data mining, where the main objective is to identify anomalous or unusual data from a given dataset. However, there is no formal categorization of application-specific anomaly detection techniques for big data and this ignites a confusion for the data miners. In this paper, we categorise anomaly detection techniques based on nearest neighbours, clustering and statistical approaches and investigate the performance analysis of these techniques in critical infrastructure applications such as SCADA systems. Extensive experimental analysis is conducted to compare representative algorithms from each of the categories using seven benchmark datasets (both real and simulated) in SCADA systems. The effectiveness of the representative algorithms is measured through a number of metrics. We highlighted the set of algorithms that are the best performing for SCADA systems.
Copyright © 2015 Mohiuddin Ahmed et al., licensed to ICST. This is an open access article distributed under the terms of the Creative Commons Attribution licence (http://creativecommons.org/licenses/by/3.0/), which permits unlimited use, distribution and reproduction in any medium so long as the original work is properly cited.