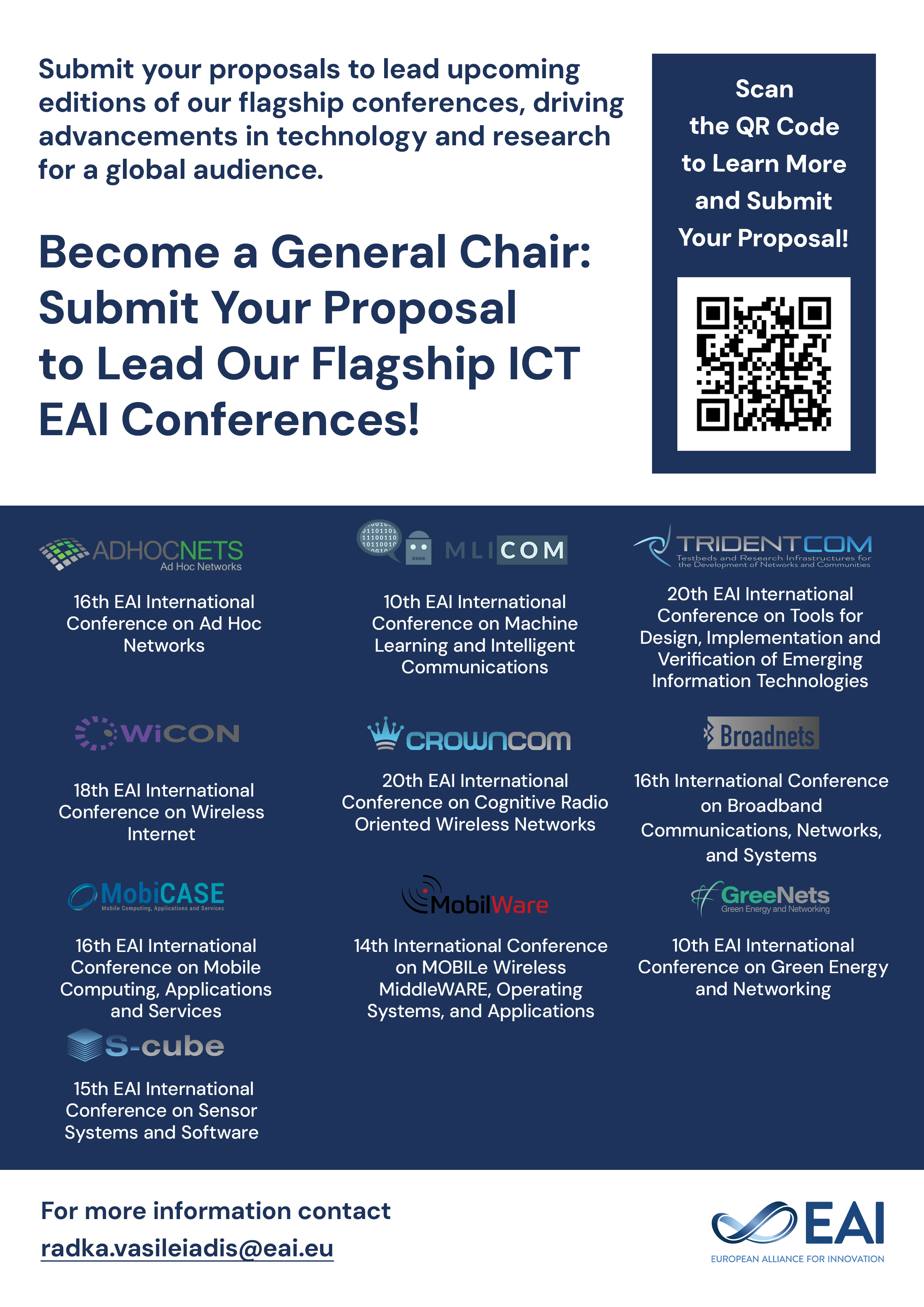
Research Article
Privacy-aware Location Privacy Preference Recommendations
@INPROCEEDINGS{10.4108/icst.mobiquitous.2014.258017, author={Yuchen Zhao and Juan Ye and Tristan Henderson}, title={Privacy-aware Location Privacy Preference Recommendations}, proceedings={11th International Conference on Mobile and Ubiquitous Systems: Computing, Networking and Services}, publisher={ICST}, proceedings_a={MOBIQUITOUS}, year={2014}, month={11}, keywords={location-based services privacy protection recommender systems prediction}, doi={10.4108/icst.mobiquitous.2014.258017} }
- Yuchen Zhao
Juan Ye
Tristan Henderson
Year: 2014
Privacy-aware Location Privacy Preference Recommendations
MOBIQUITOUS
ICST
DOI: 10.4108/icst.mobiquitous.2014.258017
Abstract
Location-Based Services have become increasingly popular due to the prevalence of smart devices and location-sharing applications such as Facebook and Foursquare. The protection of people's sensitive location data in such applications is an important requirement. Conventional location privacy protection methods, however, such as manually defining privacy rules or asking users to make decisions each time they enter a new location may be overly complex, intrusive or unwieldy. An alternative is to use machine learning to predict people's privacy preferences and automatically configure settings. Model-based machine learning classifiers may be too computationally complex to be used in real-world applications, or suffer from poor performance when training data are insufficient. In this paper we propose a location-privacy recommender that can provide people with recommendations of appropriate location privacy settings through user-user collaborative filtering. Using a real-world location-sharing dataset, we show that the prediction accuracy of our scheme (73.08%) is similar to the best performance of model-based classifiers (75.30%), and at the same time causes fewer privacy leaks (11.75% vs 12.70%). Our scheme further outperforms model-based classifiers when there are insufficient training data. Since privacy preferences are innately private, we make our recommender privacy-aware by obfuscating people's preferences. Our results show that obfuscation leads to a minimal loss of prediction accuracy (0.76%).