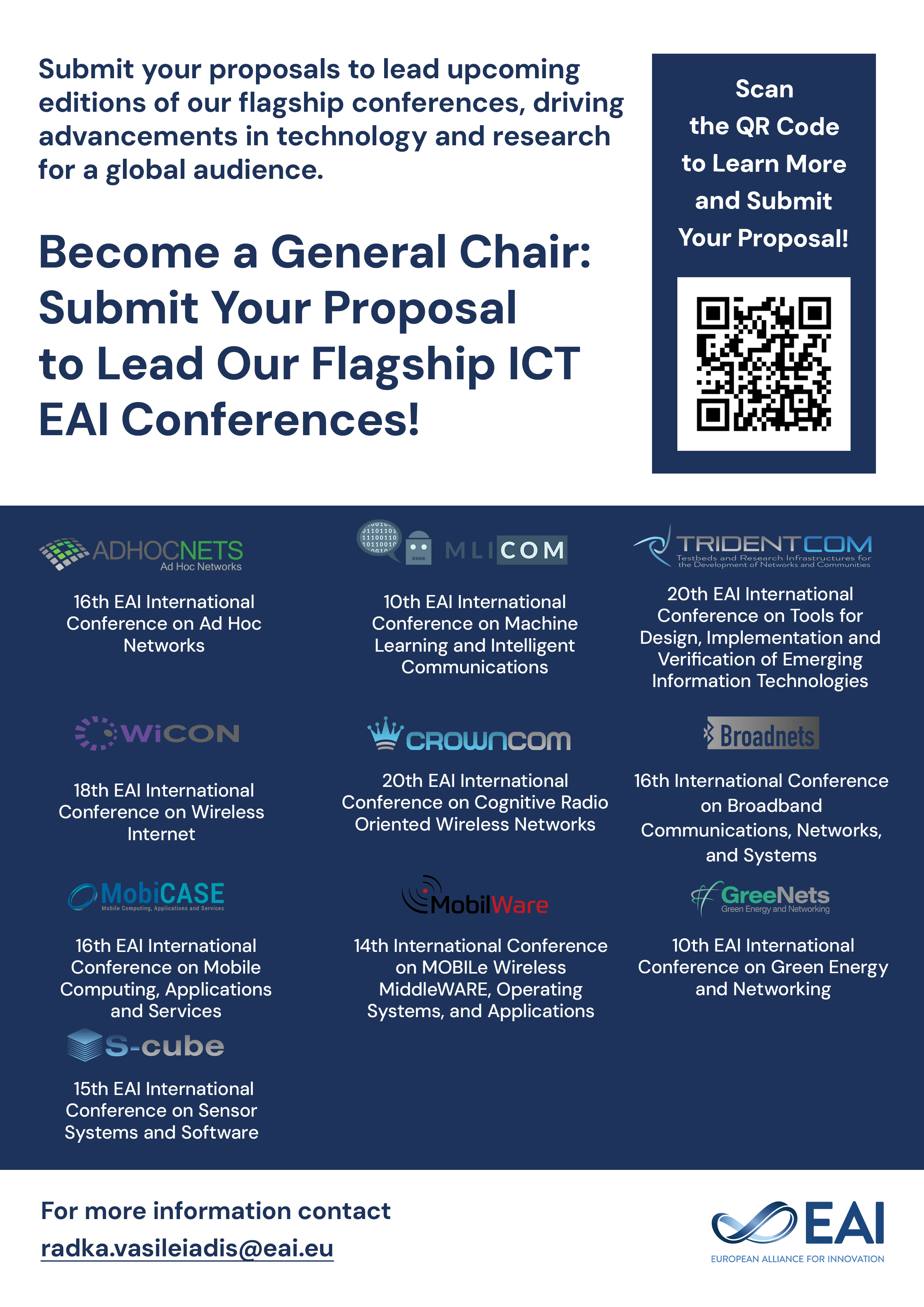
Research Article
TagTrack: Device-Free Localization and Tracking Using Passive RFID Tags
@INPROCEEDINGS{10.4108/icst.mobiquitous.2014.258004, author={Wenjie Ruan and Lina Yao and Quan Z. Sheng and Nickolas Falkner and Xue Li}, title={TagTrack: Device-Free Localization and Tracking Using Passive RFID Tags}, proceedings={11th International Conference on Mobile and Ubiquitous Systems: Computing, Networking and Services}, publisher={ICST}, proceedings_a={MOBIQUITOUS}, year={2014}, month={11}, keywords={localization rfid hidden markov model gaussian mix- ture model kernel-based nearest neighbor}, doi={10.4108/icst.mobiquitous.2014.258004} }
- Wenjie Ruan
Lina Yao
Quan Z. Sheng
Nickolas Falkner
Xue Li
Year: 2014
TagTrack: Device-Free Localization and Tracking Using Passive RFID Tags
MOBIQUITOUS
ICST
DOI: 10.4108/icst.mobiquitous.2014.258004
Abstract
Device-free passive localization aims to localize or track targets without requiring them to carry any devices or to be actively involved with the localization process. This technique has received much attention recently in a wide range of applications including elderly people surveillance, intruder detection, and indoor navigation. In this paper, we propose a novel localization and tracking system based on the Received Signal Strength field formed by a set of cost-efficient passive RFID tags. We firstly formulate localization as a classification task, where we compare several state-of-the-art learning-based classification methods including k Nearest Neighbor (kNN), Multivariate Gaussian Mixture Model (GMM) and Support Vector Machine (SVM). To track a moving subject, we propose two Hidden Markov Model (HMM)-based methods, namely GMM-based HMM and kNN-based HMM. kNN-based HMM extends kNN into a probabilistic style to approximate the Emission Probability Matrix in HMM. The proposed methods can be easily applied into other fingerprint-based tracking systems regardless of their hardware platforms. We conduct extensive experiments and the results demonstrate the effectiveness and accuracy of our approaches with up to 98% localization accuracy and an average of 0.7m tracking error.