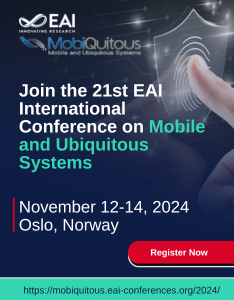
Research Article
Detection of Plugin Misuse Drive-By Download Attacks using Kernel Machines
@INPROCEEDINGS{10.4108/icst.collaboratecom.2014.257749, author={Manoj Cherukuri and Srinivas Mukkamala and Dongwan Shin}, title={Detection of Plugin Misuse Drive-By Download Attacks using Kernel Machines}, proceedings={The 9th IEEE International Workshop on Trusted Collaboration}, publisher={IEEE}, proceedings_a={TRUSTCOL}, year={2014}, month={11}, keywords={plugin exploits drive-by download web malware}, doi={10.4108/icst.collaboratecom.2014.257749} }
- Manoj Cherukuri
Srinivas Mukkamala
Dongwan Shin
Year: 2014
Detection of Plugin Misuse Drive-By Download Attacks using Kernel Machines
TRUSTCOL
ICST
DOI: 10.4108/icst.collaboratecom.2014.257749
Abstract
Malware distribution using drive-by download attacks has become the most prominent threat for organizations and individuals. Compromised web services and web applications hosted on the cloud act as the delivery medium for the exploits. The exploits included often target the vulnerabilities within the plugins of the web browsers. Implementing security controls to counter the exploits within the browsers for ensuring end point security has become a challenge. In this paper, a set of features is proposed and is extracted by monitoring the communications between the browser and the plugins during the rendering of webpages. The Support Vector Machines are trained using the defined features and the performance of the trained classifier is evaluated using a dataset with both malicious and benign use cases of the plugins. The dataset included 10,239 malicious use cases and 37,369 benign use cases. To compensate the imbalance in the distribution of the dataset, experiments were performed using weighted costs and oversampling. Our analysis shows that the Support Vector Machines trained by using the proposed set of features classified with an average accuracy of about 99.4%. On integrating the proposed approach as an inline defense, an average performance overhead of 5.14% was observed.