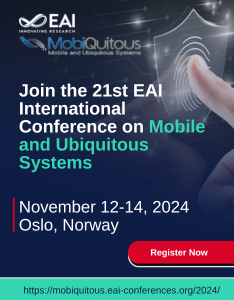
Research Article
Optimizing Heterogeneous Cognitive Radio Networks by Rigorous Algorithms and Neural Networks
@INPROCEEDINGS{10.4108/icst.bict.2014.257894, author={Mikio Hasegawa}, title={Optimizing Heterogeneous Cognitive Radio Networks by Rigorous Algorithms and Neural Networks}, proceedings={Brain-inspired Information Communication Technologies}, publisher={ICST}, proceedings_a={BIICT}, year={2015}, month={2}, keywords={cognitive radio optimization minimum cost flow problem radio resource management neural networks}, doi={10.4108/icst.bict.2014.257894} }
- Mikio Hasegawa
Year: 2015
Optimizing Heterogeneous Cognitive Radio Networks by Rigorous Algorithms and Neural Networks
BIICT
ICST
DOI: 10.4108/icst.bict.2014.257894
Abstract
Cognitive radio technology improves efficiency of radio resource usage and quality of services in congested mobile networks, by reconfiguring the wireless connection settings according to the optimum decisions, which are made based on the collected context information. This paper focuses on the optimization algorithms for the decision-making to optimize radio resource usage in heterogeneous cognitive wireless networks. For the networks with centralized management, we can apply the algorithms to obtain rigorous solutions, because all of the network context information can be collected at the central manager. In order to avoid exponential increase of computational complexity in large-scale wireless networks, we model the target optimization problem as a minimum cost flow problem, and make it possible to solve the problem in polynomial time. In the networks with distributed management, the central network manager is not necessary, but the algorithms have to be run in a distributed manner. In order to optimize the defined objective function, we introduce the distributed energy minimization of the Hopfield-Tank neural network. We derive the decision-making rule for each terminal to optimize the entire network. We show validity of the proposed algorithms by numerical simulations.