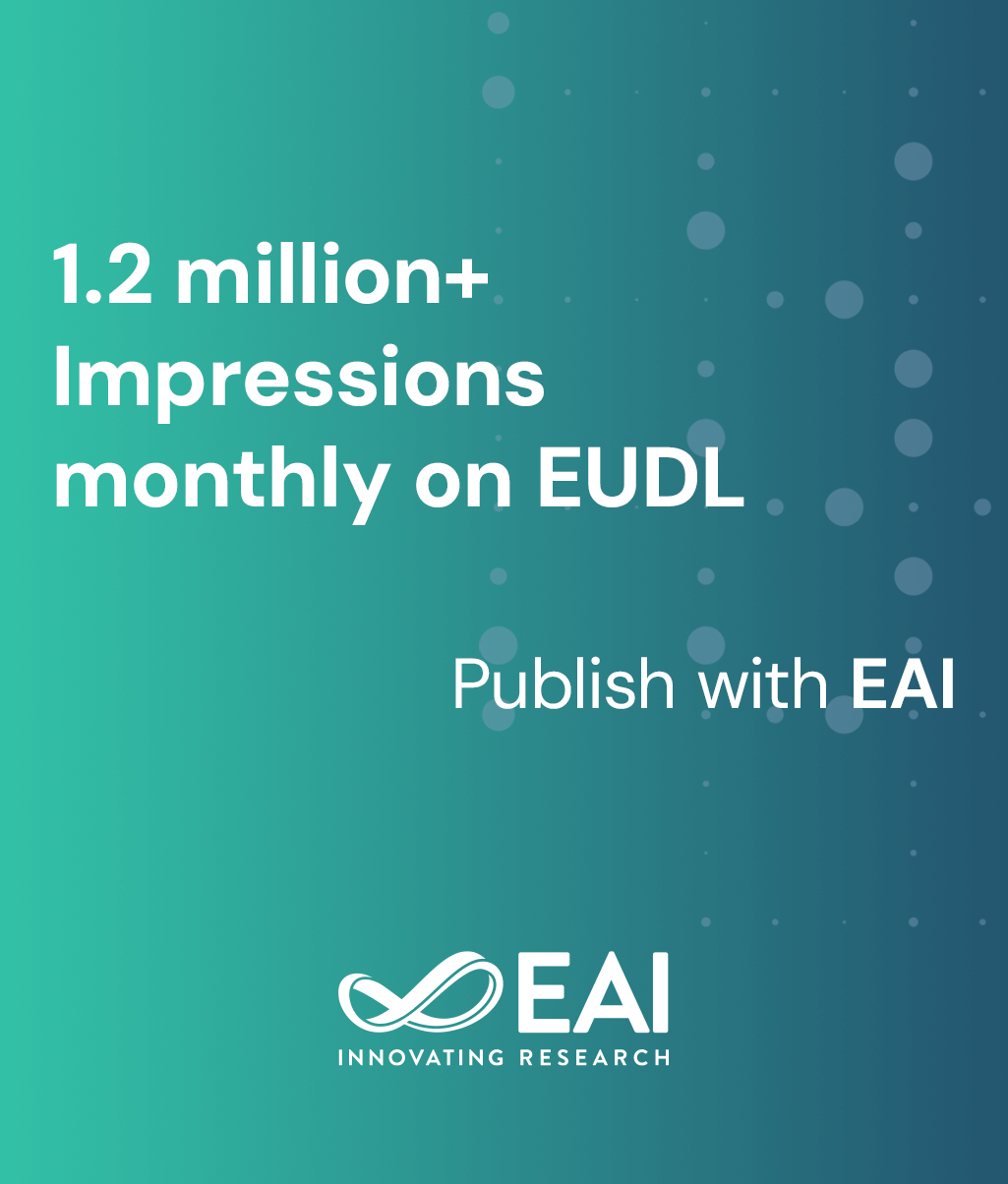
Editorial
Research on short-term power load forecasting based on deep reinforcement learning with multiple intelligences
@ARTICLE{10.4108/ew.9086, author={Tianyun Luo and Dunlin Zhu and Jinming Liu and Sheng Yang and Jinglong He and Yuan Fu}, title={Research on short-term power load forecasting based on deep reinforcement learning with multiple intelligences}, journal={EAI Endorsed Transactions on Energy Web}, volume={12}, number={1}, publisher={EAI}, journal_a={EW}, year={2025}, month={4}, keywords={Multi-intelligent body systems, deep reinforcement learning, Markov decision making, short-term power load forecasting}, doi={10.4108/ew.9086} }
- Tianyun Luo
Dunlin Zhu
Jinming Liu
Sheng Yang
Jinglong He
Yuan Fu
Year: 2025
Research on short-term power load forecasting based on deep reinforcement learning with multiple intelligences
EW
EAI
DOI: 10.4108/ew.9086
Abstract
A reliable supply of power systems is critical for industry, commerce, and residential life. Improving the accuracy and reliability of short-term electricity load forecasting plays a crucial role in ensuring the satisfaction of electricity demand and the stable operation of the power system. Therefore, to realize accurate and efficient prediction of short-term power loads, a short-term power load prediction method based on multi-intelligence deep reinforcement learning is proposed to address the complex nonlinear characteristics of load data. In this paper, we analyze the multi-intelligence application architecture in power load forecasting, and analyze the function of each intelligent unit applied to short-term power load forecasting; based on clarifying the interaction relationship of each intelligent unit in short-term power load forecasting, we model short-term power load forecasting as a distributed and partially observable Markov decision-making process, which is suitable for multi-intelligence deep reinforcement learning; based on the MATD3 algorithm, a centralized training-distributed execution framework is used to train multiple intelligences within the model to achieve short-term power load forecasting. The experimental results show that in the August short-term electricity load forecasting using the design method, the obtained MAE value is 35.94 kW, MAPE value is 4.05%, and RMSE value is 32.71 kW. In the short-term power load forecasting evaluation conducted for December, the average absolute error (MAE) value obtained was 36.75 kilowatts, the average absolute percentage error (MAPE) value was 4.51%, and the root mean square error (RMSE) value was 34.82 kilowatts. These evaluation results fully demonstrate that the design method adopted has high prediction accuracy and forecast precision. This method has demonstrated good practical value and broad application prospects in practical applications due to its high-precision prediction performance and strong prediction stability.
Copyright © 2025 Tianyun Luo et al., licensed to EAI. This is an open access article distributed under the terms of the CC BY-NCSA 4.0, which permits copying, redistributing, remixing, transformation, and building upon the material in any medium so long as the original work is properly cited.