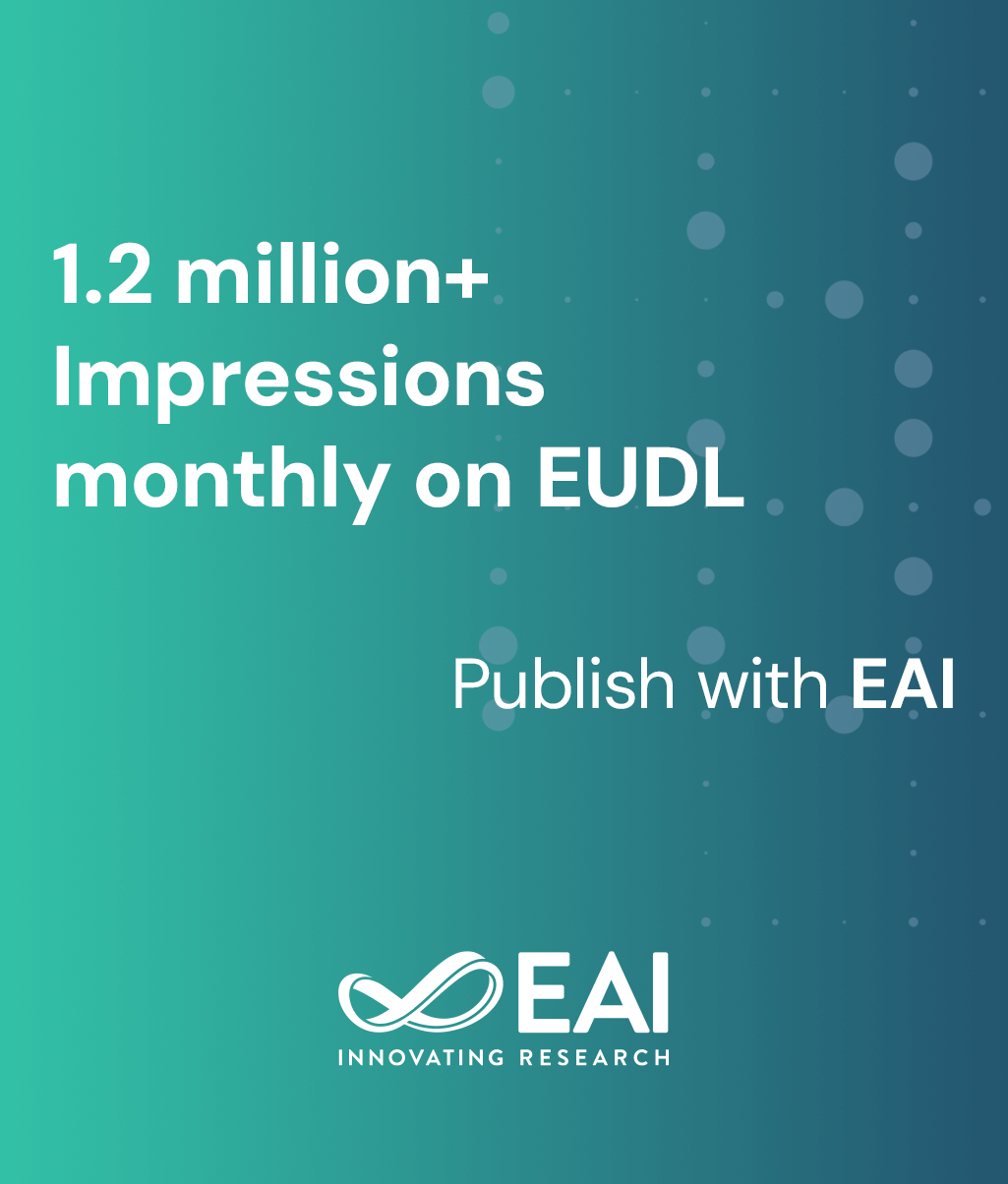
Editorial
Power System Operation Stability Assessment Method Based on Deep Convolutional Neural Network
@ARTICLE{10.4108/ew.7572, author={Jinman Luo and Yuqing Li and Qile Wang and Liyuan Liu}, title={ Power System Operation Stability Assessment Method Based on Deep Convolutional Neural Network}, journal={EAI Endorsed Transactions on Energy Web}, volume={12}, number={1}, publisher={EAI}, journal_a={EW}, year={2025}, month={5}, keywords={Convolutional neural network, power system, stability, Deep learning}, doi={10.4108/ew.7572} }
- Jinman Luo
Yuqing Li
Qile Wang
Liyuan Liu
Year: 2025
Power System Operation Stability Assessment Method Based on Deep Convolutional Neural Network
EW
EAI
DOI: 10.4108/ew.7572
Abstract
INTRODUCTION: For the assessment of power system stability, a power system assessment method based on a deep convolutional neural network is studied. OBJECTIVES: Through the improvement of the integrated convolutional neural network (CNN) network model, the impact of insufficient transient stability assessment caused by sample misjudgment and sample omission is effectively reduced. METHODS: We adopt the hierarchical real-time prediction model to evaluate the stability and instability of the determined stable samples and unstable samples, thereby improving the timeliness and accuracy of transient evaluation. RESULTS: Through experimental comparison, the integrated CNN network model in this study has obvious advantages in accuracy compared with the single CNN network. Compared with other algorithm reference models, this model has a higher evaluation accuracy of 98.39%, far exceeding other comparison models. CONCLUSION: By further evaluating the model’s accuracy, it is proved that the model can provide an effective reference for the follow-up power system stability prevention and has important application value.
Copyright © 2025 J. Luo et al., licensed to EAI. This is an open access article distributed under the terms of the CC BY-NC-SA 4.0, which permits copying, redistributing, remixing, transformation, and building upon the material in any medium so long as the original work is properly cited.