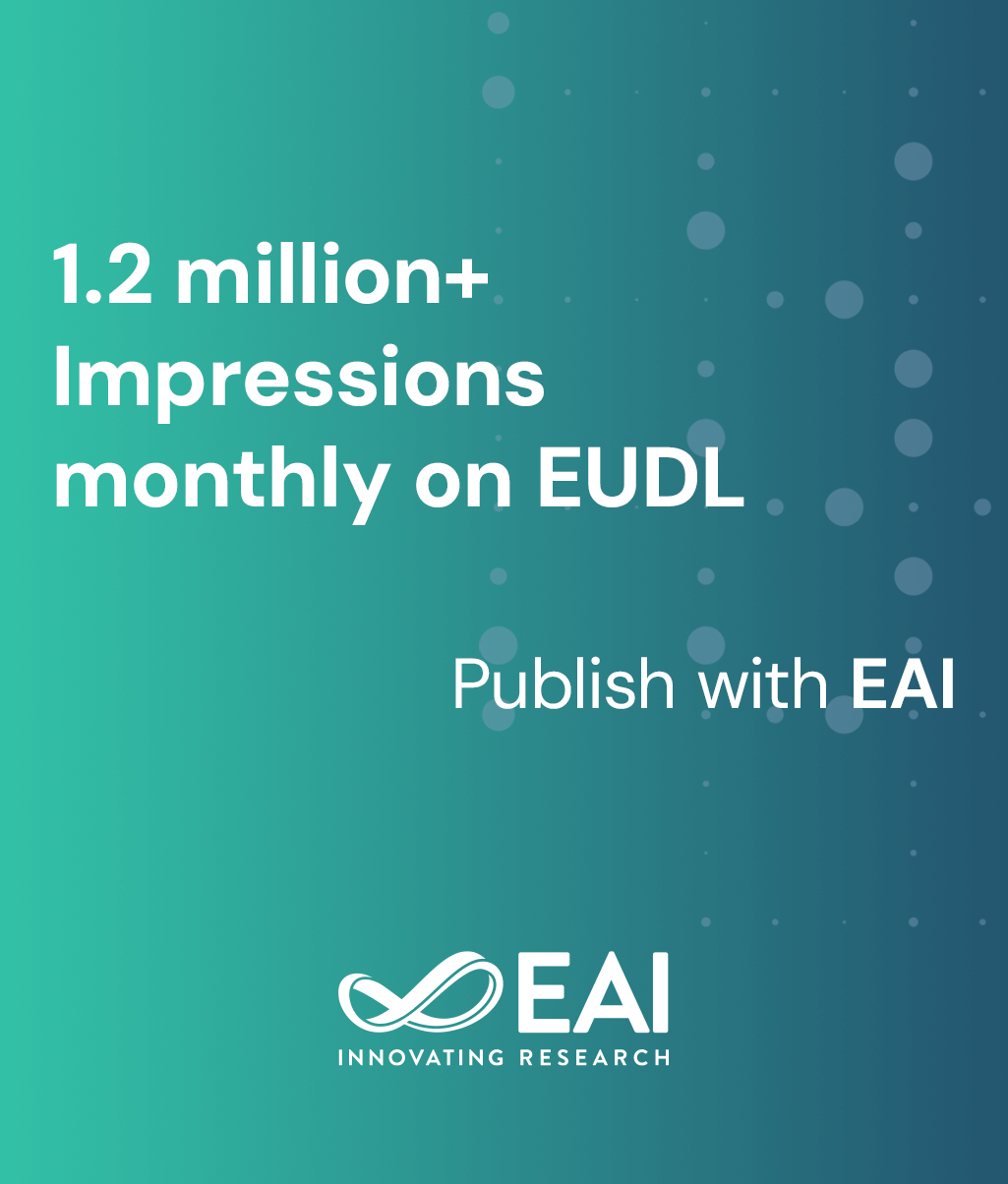
Editorial
Risk prediction method for power Internet of Things operation based on ensemble learning
@ARTICLE{10.4108/ew.6045, author={Chao Hong and Xiaoyun Kuang and Yiwei Yang and Yixin Jiang and Yunan Zhang}, title={Risk prediction method for power Internet of Things operation based on ensemble learning}, journal={EAI Endorsed Transactions on Energy Web}, volume={12}, number={1}, publisher={EAI}, journal_a={EW}, year={2025}, month={4}, keywords={adaptive synthetic oversampling, ensemble learning, power internet of things, prediction, risk}, doi={10.4108/ew.6045} }
- Chao Hong
Xiaoyun Kuang
Yiwei Yang
Yixin Jiang
Yunan Zhang
Year: 2025
Risk prediction method for power Internet of Things operation based on ensemble learning
EW
EAI
DOI: 10.4108/ew.6045
Abstract
INTRODUCTION: The power Internet of Things is an important strategic support for the State Grid Corporation of China to build an international leading energy internet enterprise. However, the operating environment of the power Internet of Things is complex and varied, which has serious implications for the safe operation of the power Internet of Things. OBJECTIVES: To timely predict the various risk. METHODS: A data set is fused based on time series. The training set is over-sampled using an adaptive synthetic oversampling method. Then, by jointly considering the contribution of features to classification and the correlation between features, a risk prediction method ground on ensemble learning is established. RESULTS: From the results, the accuracy of predicting 5 risk categories increased by 7.00%, 1.10%, 2.20%, 2.30%, and 0.60%, respectively, reducing the features from the original 118 columns to 60 columns and reducing the data dimension by 49.00%. Compared with traditional models, the accuracy was 98.61%, and the overall accuracy was improved by 0.60%. CONCLUSION: This risk prediction scheme can quickly and accurately predict the risk categories that affect its operation. It has high prediction accuracy and fast speed than other algorithms. This research can provide strong assistance for security decision-making in the power Internet of Things.
Copyright © 2025 Ch. Hong et al., licensed to EAI. This is an open access article distributed under the terms of the CC BY-NC-SA 4.0, which permits copying, redistributing, remixing, transformation, and building upon the material in any medium so long as the original work is properly cited.