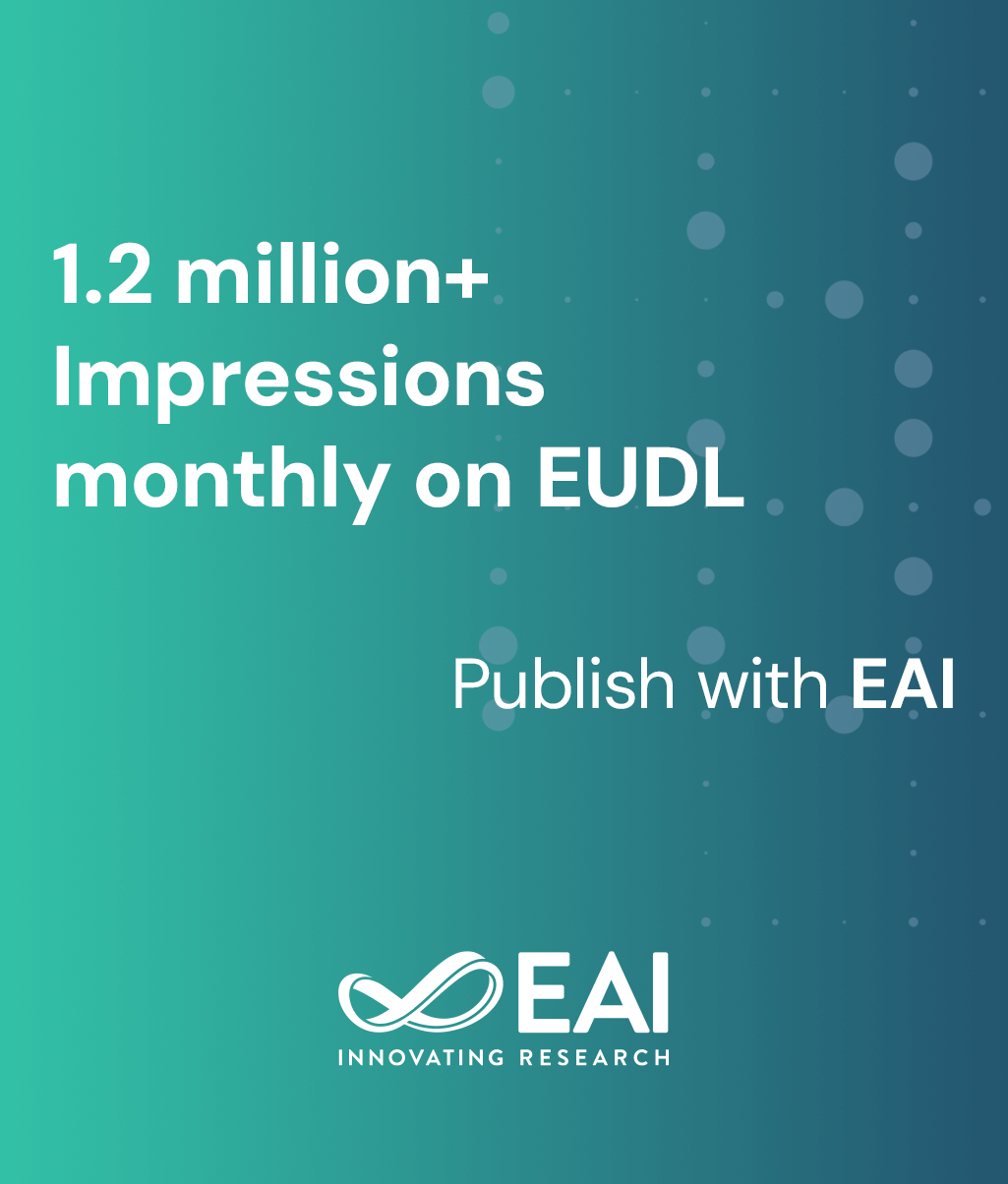
Editorial
A Short term Electricity Load Forecasting for Community Residents Based on Federated Learning and Considering Privacy Protection
@ARTICLE{10.4108/ew.6007, author={Bo Peng and Jing Zuo and Xianfu Gong and Yaodong Li and Weijie Wu and Yixin Li}, title={A Short term Electricity Load Forecasting for Community Residents Based on Federated Learning and Considering Privacy Protection}, journal={EAI Endorsed Transactions on Energy Web}, volume={11}, number={1}, publisher={EAI}, journal_a={EW}, year={2024}, month={10}, keywords={Neural Network, Privacy, Federated Learning, Aggregation, Short-term Load Forecasting}, doi={10.4108/ew.6007} }
- Bo Peng
Jing Zuo
Xianfu Gong
Yaodong Li
Weijie Wu
Yixin Li
Year: 2024
A Short term Electricity Load Forecasting for Community Residents Based on Federated Learning and Considering Privacy Protection
EW
EAI
DOI: 10.4108/ew.6007
Abstract
INTRODUCTION: As the penetration rate of renewable energy increases and patterns of energy demand evolve, fluctuations on both the supply and demand sides of electricity are becoming more pronounced. Consequently, accurate forecasting of community residential electrical loads has become crucial. OBJECTIVES: Although the widespread adoption of smart meters among residents provides abundant data for model training, strict challenges arise during the training process due to the need for privacy protection and data security. METHODS: This paper proposes a privacy-preserving community residential short-term electric load forecasting method based on federated learning. Initially, the method applies shared random masking encryption to the sensitive data of community residents, ensuring data privacy while maintaining consistency with the original data after preprocessing. Subsequently, a private data aggregation scheme is established to perform dynamic clustering of the community’s electrical load. RESULTS: The clustered model then serves as the basis for establishing individual load forecasting models for each category of community residents to predict short-term electrical loads. Finally, an empirical analysis is performed using the electrical load data from 120 households across 6 communities in a city in Southern China. CONCLUSION: The analysis demonstrates that the proposed method can achieve the prediction of community residential electrical loads without sharing residents’ data, thus verifying the effectiveness of this approach.
Copyright © 2024 B. Peng et al., licensed to EAI. This is an open access article distributed under the terms of the CC BY-NC-SA 4.0, which permits copying, redistributing, remixing, transformation, and building upon the material in any medium so long as the original work is properly cited.