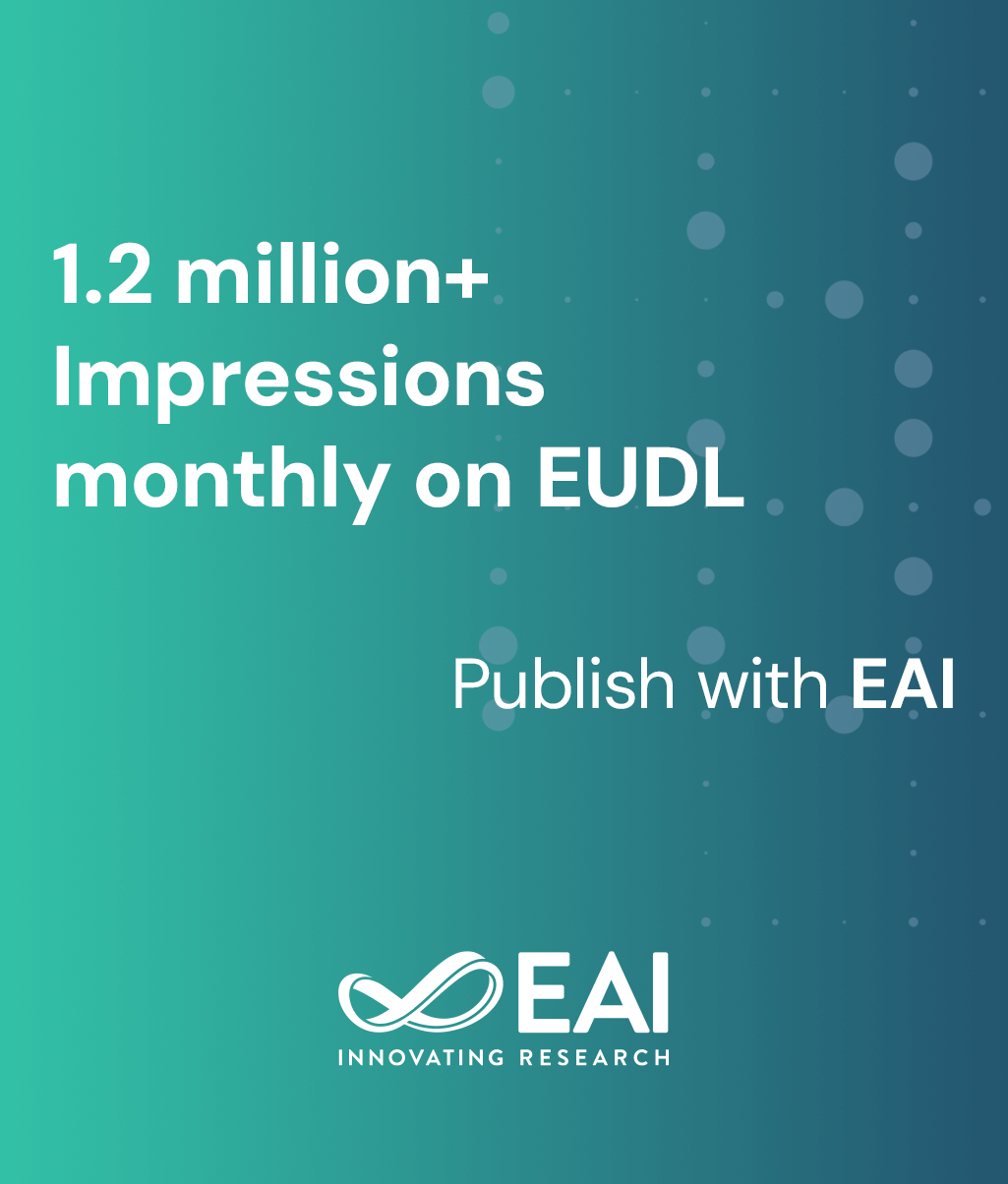
Research Article
Construction of a Fast Monitoring System for Electric Energy Equipment Status Based on Data Mining
@ARTICLE{10.4108/ew.5869, author={Fusheng Wei and Xue Li and Weiwen Chen and Zhaokai Liang and Zhaopeng Huang}, title={Construction of a Fast Monitoring System for Electric Energy Equipment Status Based on Data Mining}, journal={EAI Endorsed Transactions on Energy Web}, volume={12}, number={1}, publisher={EAI}, journal_a={EW}, year={2025}, month={4}, keywords={Data mining, Electric energy equipment, Status monitoring, Edge perception, Generative adversarial network}, doi={10.4108/ew.5869} }
- Fusheng Wei
Xue Li
Weiwen Chen
Zhaokai Liang
Zhaopeng Huang
Year: 2025
Construction of a Fast Monitoring System for Electric Energy Equipment Status Based on Data Mining
EW
EAI
DOI: 10.4108/ew.5869
Abstract
In modern power system operation, it is crucial to achieve fast and accurate monitoring of the electrical equipment status. To achieve this fast and accurate detection, this study proposes a generative adversarial network that combines edge features to amplify and recognize infrared images of devices, aiming to improve the model’s training effect. This model extracted edge features from infrared images to eliminate background noise in infrared images to achieve the goal of improving the accurate monitoring of the status of electrical equipment. The results showed that on the balanced dataset, the recognition accuracy of the model could reach about 96%, and the recognition effect of the model was relatively stable. On imbalanced datasets, the highest model recognition accuracy was around 89%, and the model recognition accuracy fluctuated greatly. The constructed model effectively improves the accuracy of monitoring the operating status of electric energy equipment, achieving fast and accurate monitoring of this state. This study can achieve rapid monitoring of the operating status of electric energy equipment, effectively reducing the operation and maintenance costs of the power system.
Copyright © 2024 F. Wei et al., licensed to EAI. This is an open access article distributed under the terms of the CC BY-NC-SA 4.0, which permits copying, redistributing, remixing, transformation, and building upon the material in any medium so long as the original work is properly cited.