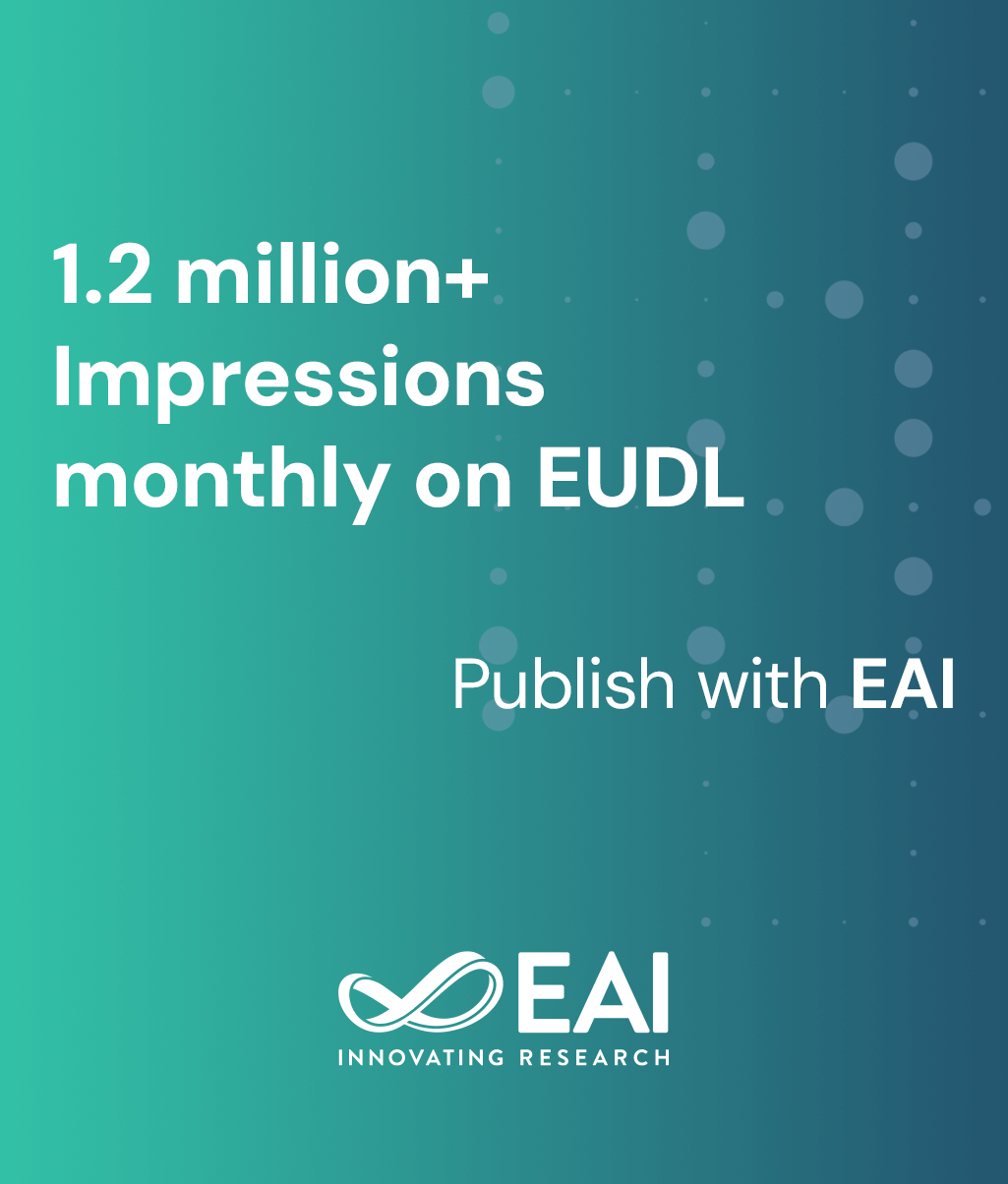
Research Article
Multi-temporal Scale Wind Power Forecasting Based on Lasso-CNN-LSTM-LightGBM
@ARTICLE{10.4108/ew.5792, author={Qingzhong Gao}, title={Multi-temporal Scale Wind Power Forecasting Based on Lasso-CNN-LSTM-LightGBM}, journal={EAI Endorsed Transactions on Energy Web}, volume={11}, number={1}, publisher={EAI}, journal_a={EW}, year={2024}, month={12}, keywords={wind power prediction, ICEEMDAN, CNN network, LSTM, LightGBM model, multi-time scale}, doi={10.4108/ew.5792} }
- Qingzhong Gao
Year: 2024
Multi-temporal Scale Wind Power Forecasting Based on Lasso-CNN-LSTM-LightGBM
EW
EAI
DOI: 10.4108/ew.5792
Abstract
Due to the increasingly severe climate problems, wind energy has received widespread attention as the most abundant energy on Earth. However, due to the uncertainty of wind energy, a large amount of wind energy is wasted, so accurate wind power prediction can greatly improve the utilization of wind energy. To increase the forecast for wind energy accuracy across a range of time scales, this paper presents a multi-time scale wind power prediction by constructing an ICEEMDAN-CNN-LSTM-LightGBM model. Initially, feature selection is performed using Lasso regression to identify the most significant variables affecting the forecast for wind energy across distinct time intervals. Subsequently, the ICEEMDAN is utilized to break down the wind power data into various scales to capture its nonlinear and non-stationary characteristics. Following this, a deep learning model based on CNN and LSTM networks is developed, with the CNN responsible for extracting spatial features from the time series data, and the LSTM designed to capture the temporal relationships. Finally, the outputs of the deep learning model are fed into the LightGBM model to leverage its superior learning capabilities for the ultimate prediction of wind power. Simulation experiments demonstrate that the proposed ICEEMDAN-CNN-LSTM-LightGBM model achieves higher accuracy in multi-time scale wind power prediction, providing more reliable decision assistance with the management and operation of wind farms.
Copyright © Q. Gao Author et al., licensed to EAI. This is an open access article distributed under the terms of the CC BY-NC-SA 4.0, which permits copying, redistributing, remixing, transformation, and building upon the material in any medium so long as the original work is properly cited.