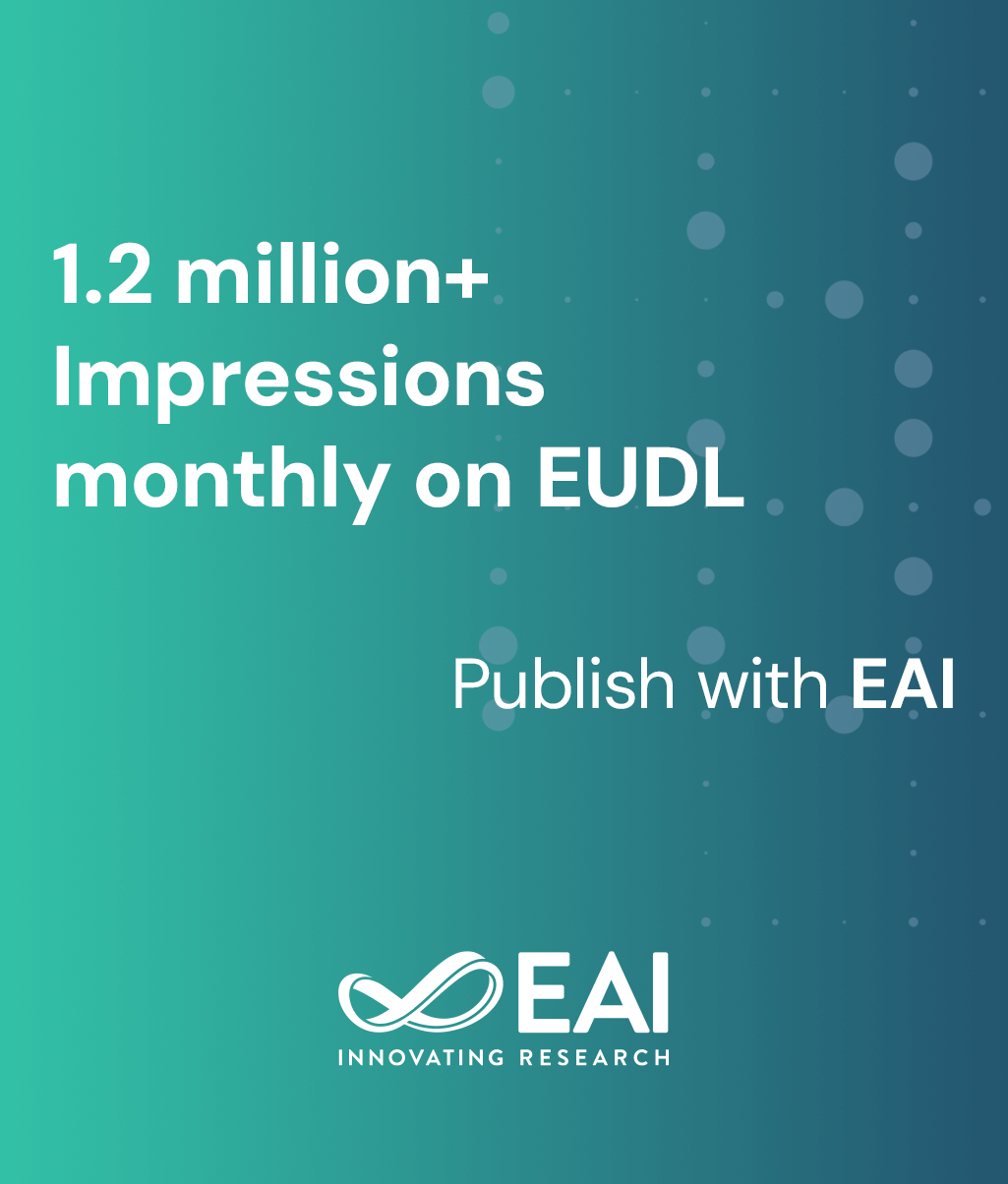
Research Article
An Ultra-Short-Term Wind Power Prediction Method Based on Quadratic Decomposition and Multi-Objective Optimization
@ARTICLE{10.4108/ew.5787, author={Hayou Chen and Zhenglong Zhang and Shaokai Tong and Peiyuan Chen and Zhiguo Wang and Hai Huang}, title={An Ultra-Short-Term Wind Power Prediction Method Based on Quadratic Decomposition and Multi-Objective Optimization}, journal={EAI Endorsed Transactions on Energy Web}, volume={11}, number={1}, publisher={EAI}, journal_a={EW}, year={2024}, month={4}, keywords={wind power prediction, quadratic decomposition, multi-objective optimization, deep bidirectional long short-term memory, sand cat swarm optimization algorithm}, doi={10.4108/ew.5787} }
- Hayou Chen
Zhenglong Zhang
Shaokai Tong
Peiyuan Chen
Zhiguo Wang
Hai Huang
Year: 2024
An Ultra-Short-Term Wind Power Prediction Method Based on Quadratic Decomposition and Multi-Objective Optimization
EW
EAI
DOI: 10.4108/ew.5787
Abstract
To augment the accuracy, stability, and qualification rate of wind power prediction, thereby fostering the secure and economical operation of wind farms, a method predicated on quadratic decomposition and multi-objective optimization for ultra-short-term wind power prediction is proposed. Initially, the original wind power signal is decomposed using a quadratic decomposition method constituted by the Complete Ensemble Empirical Mode Decomposition with Adaptive Noise (CEEMDAN), Fuzzy Entropy (FE), and Symplectic Geometry Mode Decomposition (SGMD), thereby mitigating the randomness and volatility of the original signal. Subsequently, the decomposed signal components are introduced into the Deep Bidirectional Long Short-Term Memory (DBiLSTM) neural network for time series modeling, and the Sand Cat Swarm Optimization Algorithm (SCSO) is employed to optimize the network hyperparameters, thereby enhancing the network’s predictive performance. Ultimately, a multi-objective optimization loss that accommodates accuracy, stability, and grid compliance is proposed to guide network training. Experimental results reveal that the employed quadratic decomposition method and the proposed multi-objective optimization loss can effectively bolster the model’s predictive performance. Compared to other classical methods, the proposed method achieves optimal results across different seasons, thereby demonstrating robust practicality.
Copyright © 2024 H. Chen et al., licensed to EAI. This is an open access article distributed under the terms of the CC BY-NC-SA 4.0, which permits copying, redistributing, remixing, transformation, and building upon the material in any medium so long as the original work is properly cited.