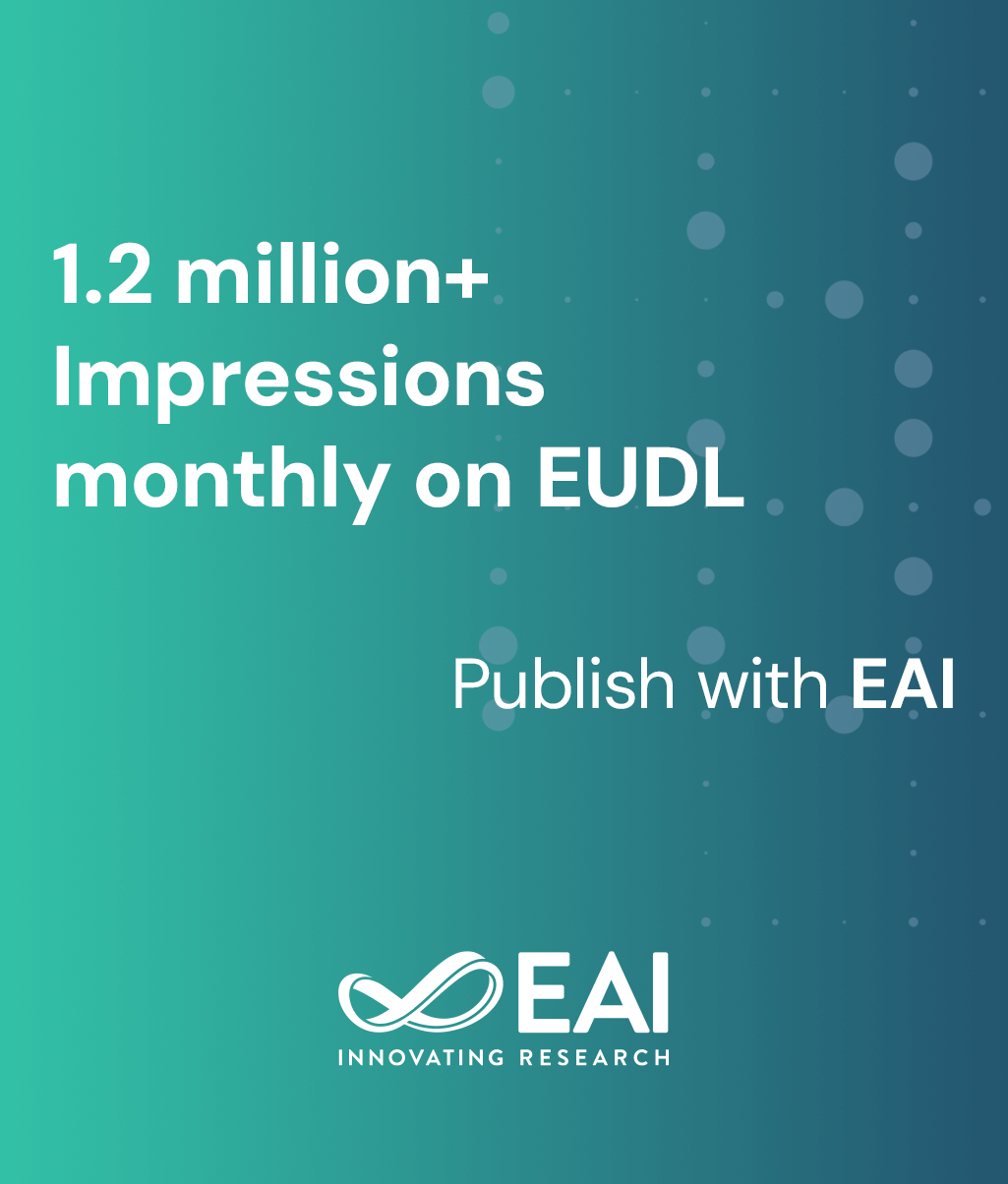
Research Article
Research on Surface Defect Detection Method of Photovoltaic Power Generation Panels——Comparative Analysis of Detecting Model Accuracy
@ARTICLE{10.4108/ew.5741, author={Yunxin Wang and Zhi Zhang and Jialiang Zhang and Jiangning Han and Jianguo Lian and Yifeng Qi and Xiaowei Liu and Jiangyang Guo and Xiaoju Yin}, title={Research on Surface Defect Detection Method of Photovoltaic Power Generation Panels------Comparative Analysis of Detecting Model Accuracy}, journal={EAI Endorsed Transactions on Energy Web}, volume={11}, number={1}, publisher={EAI}, journal_a={EW}, year={2024}, month={12}, keywords={Computer vision, Deep learning, Solar panels, Photovoltaic defects}, doi={10.4108/ew.5741} }
- Yunxin Wang
Zhi Zhang
Jialiang Zhang
Jiangning Han
Jianguo Lian
Yifeng Qi
Xiaowei Liu
Jiangyang Guo
Xiaoju Yin
Year: 2024
Research on Surface Defect Detection Method of Photovoltaic Power Generation Panels——Comparative Analysis of Detecting Model Accuracy
EW
EAI
DOI: 10.4108/ew.5741
Abstract
INTRODUCTION: Research on intelligent defect detection technology using machine vision was conducted to address the challenging problem of detecting and localizing PV defects in photovoltaic power generation system operation and maintenance. OBJECTIVES: The aim is to improve the accuracy of PV defect detection and enhance the operation and maintenance efficiency of PV power plants. METHODS: In this paper, three detection methods such as image processing based detection, traditional machine learning based detection, and deep learning algorithm based detection are discussed and compared, and analyzed respectively. It is finally concluded that the deep learning based detection is more efficient in comparison. Then further analysis and simulation experiments are done through several detection algorithms based on deep learning. RESULTS: The experiment yields a high accuracy of the detection model based on the Faster-RCNN algorithm. Its mAP value reaches 92.6%. The detection model based on the YOLOv5 algorithm reaches a mAP value of 91.4%. But its speed is as much as 7 times faster than the model based on the Faster-RCNN algorithm. CONCLUSION: Comprehensive speed and accuracy index. Combining the needs of PV defect detection in the operation and maintenance of PV power generation systems with the results of simulation experiments. It is concluded that the detection model based on the YOLOv5 algorithm can provide better detection capability. Modeling with this algorithm is more suitable for PV defect detection.
Copyright © 2024 Y. Yang et al., licensed to EAI. This is an open access article distributed under the terms of the CC BY-NC-SA 4.0, which permits copying, redistributing, remixing, transformation, and building upon the material in any medium so long as the original work is properly cited.