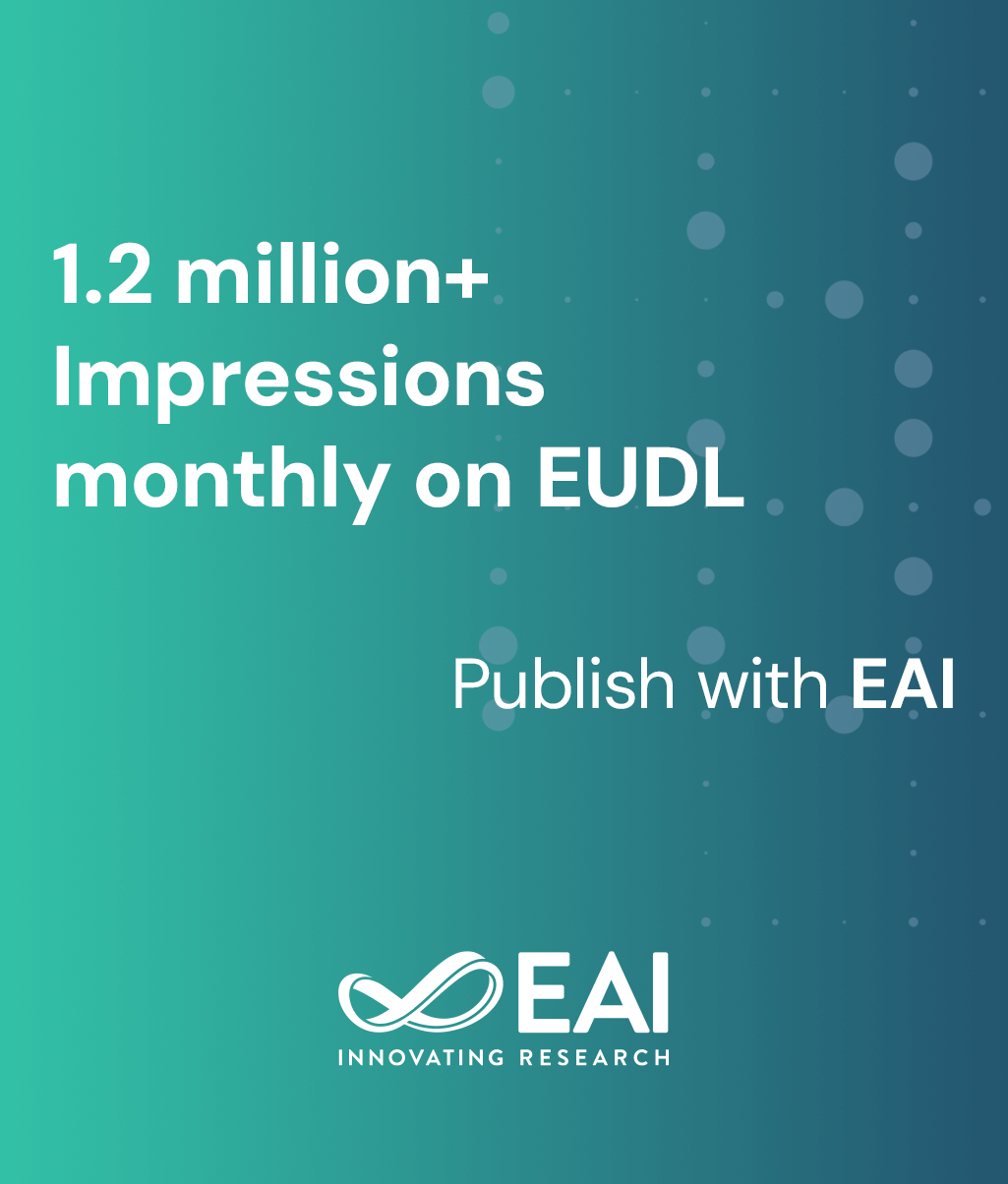
Research Article
Fault diagnosis of gearboxin wind turbine based on EMD-DCGAN
@ARTICLE{10.4108/ew.5652, author={Guangyi Meng and Yuxing An and Dong Zhang and Xudong Li}, title={Fault diagnosis of gearboxin wind turbine based on EMD-DCGAN}, journal={EAI Endorsed Transactions on Energy Web}, volume={11}, number={1}, publisher={EAI}, journal_a={EW}, year={2024}, month={4}, keywords={Empirical mode decomposition method, Data expansion, Generative adversarial neural network, Fault diagnosis of wind turbine gearbox}, doi={10.4108/ew.5652} }
- Guangyi Meng
Yuxing An
Dong Zhang
Xudong Li
Year: 2024
Fault diagnosis of gearboxin wind turbine based on EMD-DCGAN
EW
EAI
DOI: 10.4108/ew.5652
Abstract
INTRODUCTION: Wind turbine gearbox fault diagnosis is of great significance for the safe and stable operation of wind turbines. The accuracy of wind turbine gearbox fault diagnosis can be effectively improved by using complete wind turbine gearbox fault data and efficient fault diagnosis algorithms.A wind turbine gearbox fault diagnosis method based on EMD-DCGAN method is proposed in this paper. OBJECTIVES: It can solve the problem when the sensor fails or the data transmission fails, it will lead to errors in the wind turbine gearbox fault data, which in turn will lead to a decrease in the wind turbine gearbox fault diagnosis accuracy. METHODS: Firstly, the outliers in the sample data need to be detected and removed. In this paper, the EMD method is used to eliminate outliers in the wind turbine gearbox fault data samples with the aim of enhancing the true continuity of the samples; secondly, in order to make up for the lack of missing samples, a data enhancement algorithm based on a GAN network is proposed in the paper, which is able to effectively perfect the missing items of the sample data; lastly, in order to improve the accuracy of wind turbine gearbox faults, a DCGAN neural network-based fault diagnosis method is proposed, which effectively combines the data dimensionality reduction feature of deep learning method and the data enhancement feature of generative adversarial network, and can improve the accuracy and speed of fault diagnosis. RESULTS and CONCLUSIONS: The experimental results show that the proposed method can effectively identify wind turbine gearbox fault conditions, and verify the effectiveness of the algorithm under different sample data conditions.
Copyright © 2024 G. Meng et al., licensed to EAI. This is an open access article distributed under the terms of the CC BY-NC-SA 4.0, which permits copying, redistributing, remixing, transformation, and building upon the material in any medium so long as the original work is properly cited.