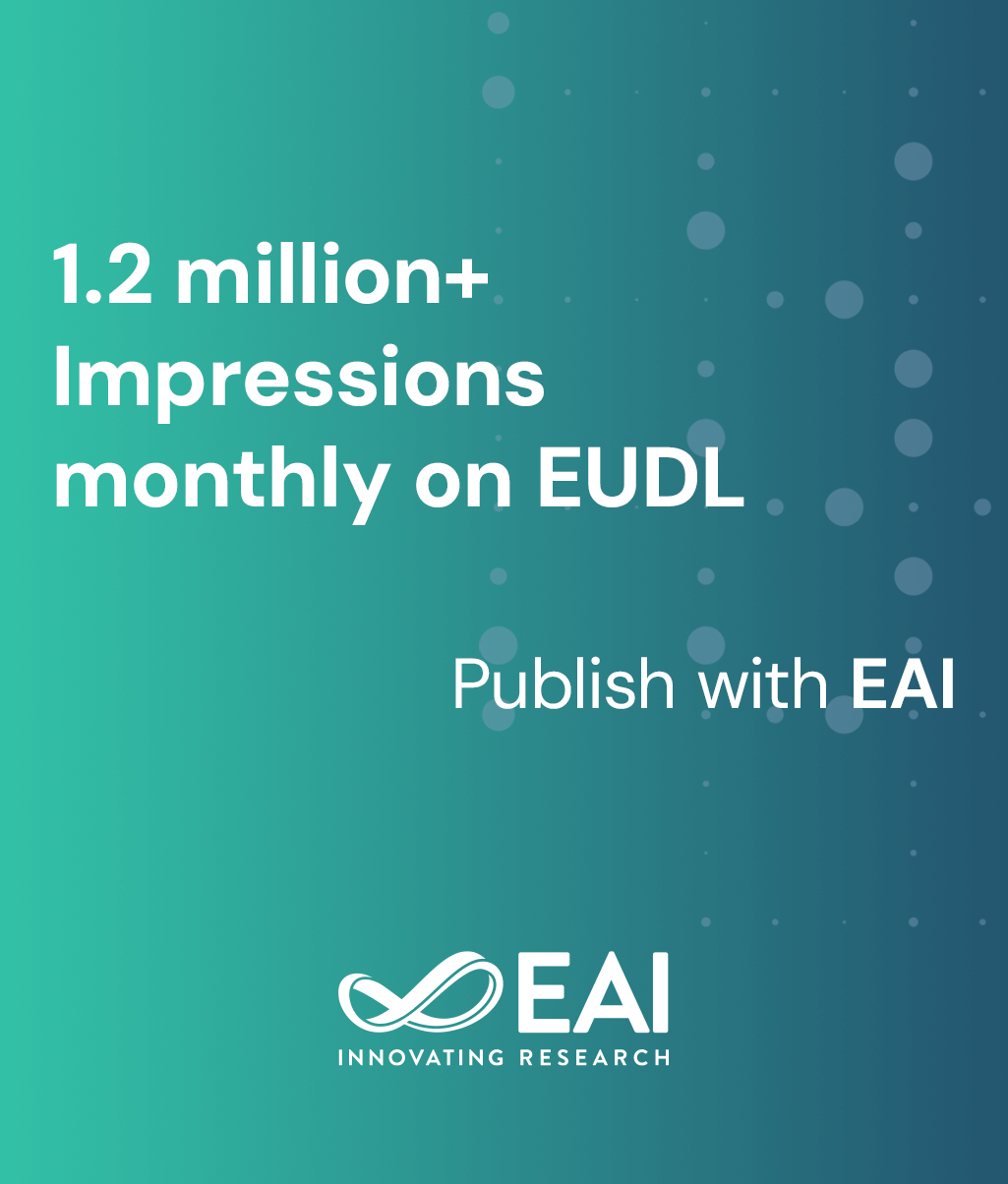
Research Article
Prognostication of Weather Patterns using Meteorological Data and ML Techniques
@ARTICLE{10.4108/ew.5648, author={Saksham Mathur and Sanjeev Kumar and Tanupriya Choudhury}, title={Prognostication of Weather Patterns using Meteorological Data and ML Techniques}, journal={EAI Endorsed Transactions on Energy Web}, volume={11}, number={1}, publisher={EAI}, journal_a={EW}, year={2024}, month={4}, keywords={Meteorological Data, Precipitation Prediction, Radial Basis Function Neural Network, Classifier Random Forest, Gradient Boosting}, doi={10.4108/ew.5648} }
- Saksham Mathur
Sanjeev Kumar
Tanupriya Choudhury
Year: 2024
Prognostication of Weather Patterns using Meteorological Data and ML Techniques
EW
EAI
DOI: 10.4108/ew.5648
Abstract
In the field of modern weather prediction, the accurate classification is essential, impacting critical sectors such as agriculture, aviation, and water resource management. This research presents a weather forecasting model employing two influential classifiers random forest and technique based on gradient boosting, both implemented using the Scikit-learn library. Evaluation is based on key metrics including F1 score, accuracy, recall, and precision, with Gradient Boosting emerging as the superior choice for precipitation prediction. The study examines the performance of Random Forest Regression, Gradient Boosting Regression, and Radial Basis Function Neural Network in forecasting precipitation, drawing on prior research that demonstrated the superiority of the Random Forest algorithm in terms of accuracy and speed. Ensemble methods, particularly the Voting Classifier, a fusion of Random Forest and Gradient Boosting, outperform individual models, offering a promising avenue for advancing weather classification.
Copyright © 2024 S. Mathur et al., licensed to EAI. This is an open access article distributed under the terms of the CC BY-NC-SA 4.0, which permits copying, redistributing, remixing, transformation, and building upon the material in any medium so long as the original work is properly cited.