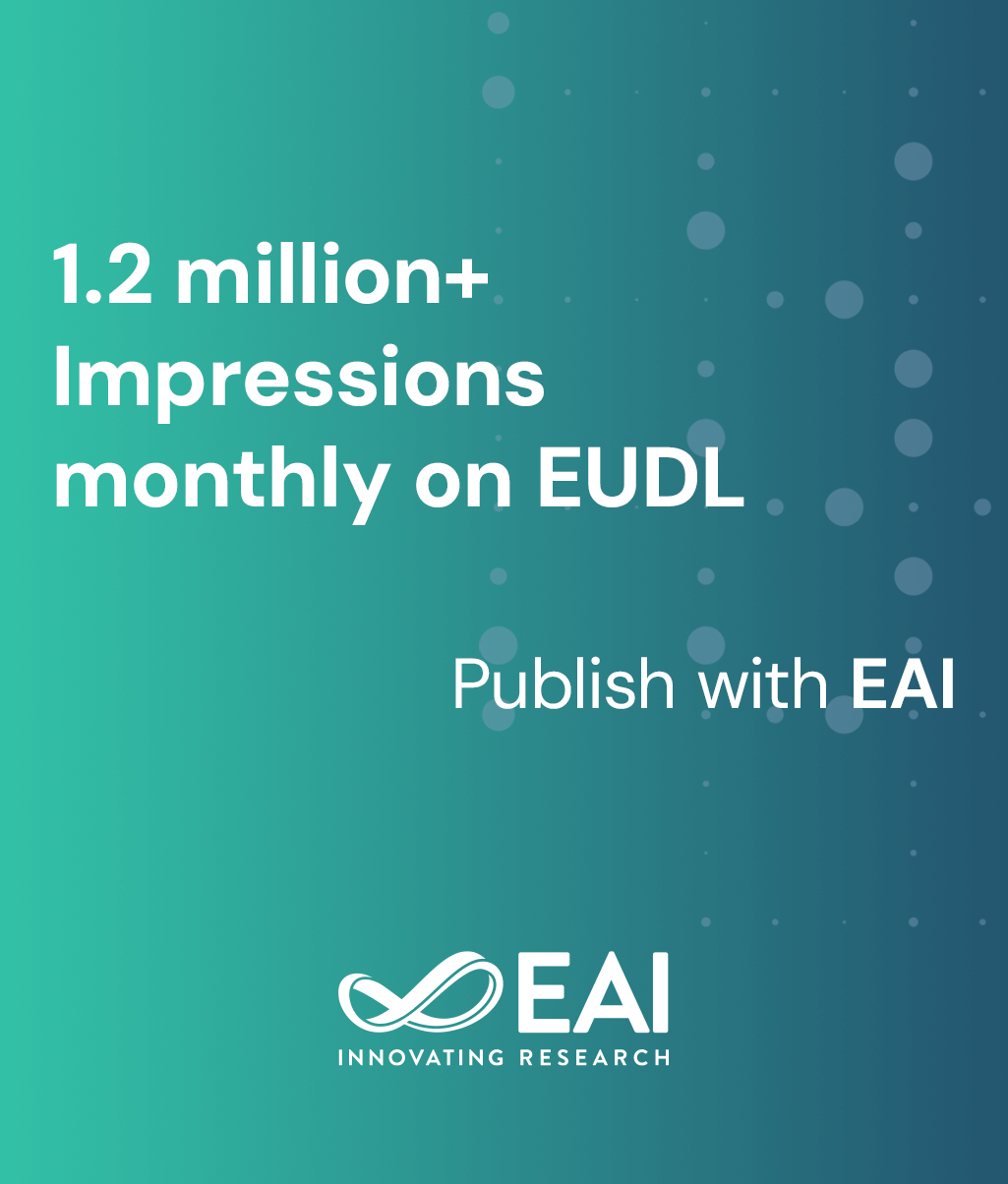
Research Article
An Architecture and Review of Intelligence Based Traffic Control System for Smart Cities
@ARTICLE{10.4108/ew.4964, author={Manasa Kommineni and K. K. Baseer}, title={An Architecture and Review of Intelligence Based Traffic Control System for Smart Cities}, journal={EAI Endorsed Transactions on Energy Web}, volume={11}, number={1}, publisher={EAI}, journal_a={EW}, year={2024}, month={1}, keywords={Smart Cities, QoS, Traffic flow, Offloading Decisions, Signal Timing, Energy Saving}, doi={10.4108/ew.4964} }
- Manasa Kommineni
K. K. Baseer
Year: 2024
An Architecture and Review of Intelligence Based Traffic Control System for Smart Cities
EW
EAI
DOI: 10.4108/ew.4964
Abstract
City traffic congestion can be reduced with the help of adaptable traffic signal control system. The technique improves the efficiency of traffic operations on urban road networks by quickly adjusting the timing of signal values to account for seasonal variations and brief turns in traffic demand. This study looks into how adaptive signal control systems have evolved over time, their technical features, the state of adaptive control research today, and Control solutions for diverse traffic flows composed of linked and autonomous vehicles. This paper finally came to the conclusion that the ability of smart cities to generate vast volumes of information, Artificial Intelligence (AI) approaches that have recently been developed are of interest because they have the power to transform unstructured data into meaningful information to support decision-making (For instance, using current traffic information to adjust traffic lights based on actual traffic circumstances). It will demand a lot of processing power and is not easy to construct these AI applications. Unique computer hardware/technologies are required since some smart city applications require quick responses. In order to achieve the greatest energy savings and QoS, it focuses on the deployment of virtual machines in software-defined data centers. Review of the accuracy vs. latency trade-off for deep learning-based service decisions regarding offloading while providing the best QoS at the edge using compression techniques. During the past, computationally demanding tasks have been handled by cloud computing infrastructures. A promising computer infrastructure is already available and thanks to the new edge computing advancement, which is capable of meeting the needs of tomorrow's smart cities.
Copyright © 2024 M. Kommineni et al., licensed to EAI. This is an open access article distributed under the terms of the CC BYNC-SA 4.0, which permits copying, redistributing, remixing, transformation, and building upon the material in any medium so long as the original work is properly cited.