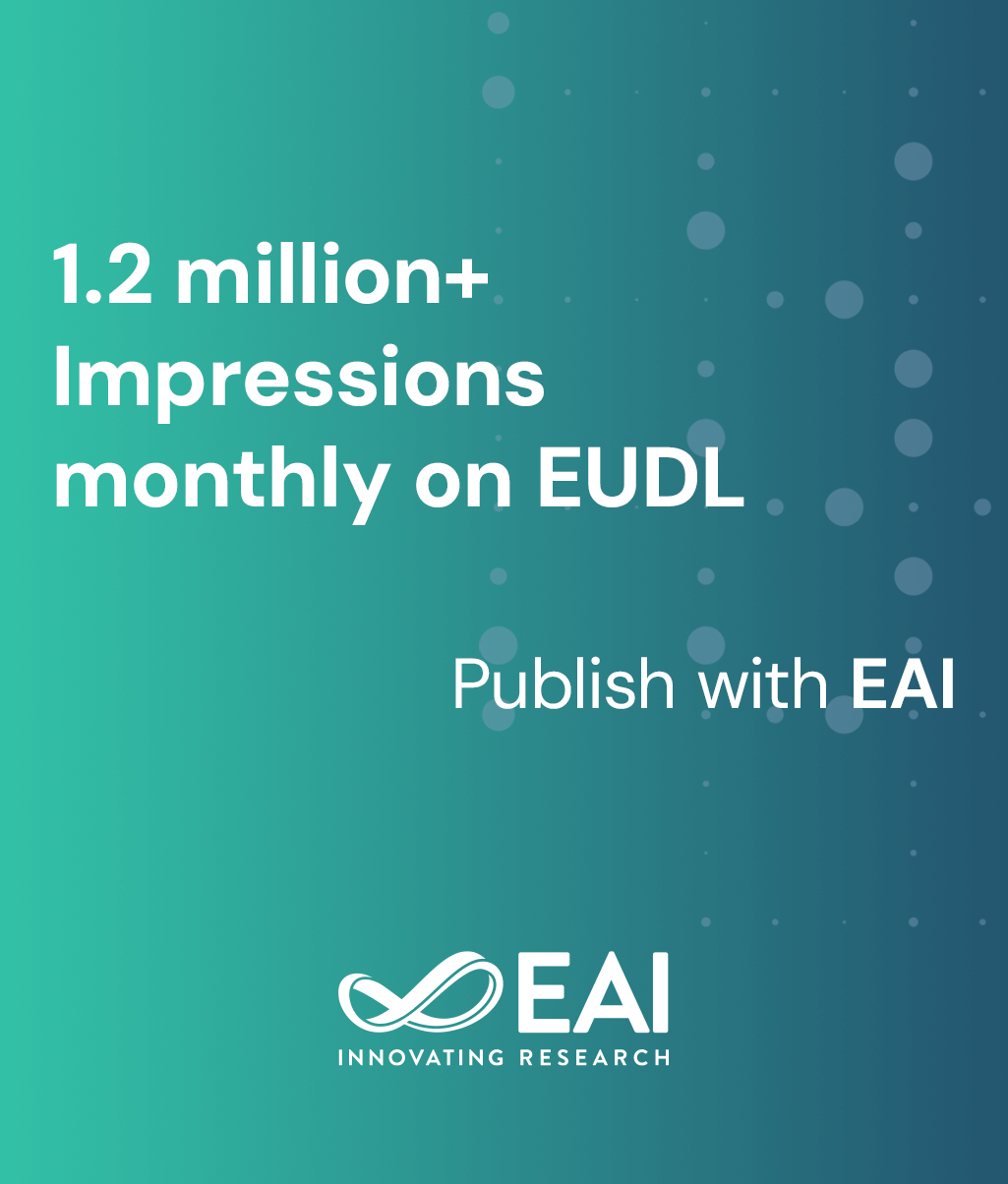
Research Article
The residual life prediction of power grid transformers based on GA-ELM computational model and digital twin data
@ARTICLE{10.4108/ew.4896, author={Xiangshang Wang and Chunlin Li and Jianguang Zhang}, title={The residual life prediction of power grid transformers based on GA-ELM computational model and digital twin data}, journal={EAI Endorsed Transactions on Energy Web}, volume={11}, number={1}, publisher={EAI}, journal_a={EW}, year={2024}, month={4}, keywords={Transformer, Power grid, Digital twin, Genetic algorithm, Extreme learning machine}, doi={10.4108/ew.4896} }
- Xiangshang Wang
Chunlin Li
Jianguang Zhang
Year: 2024
The residual life prediction of power grid transformers based on GA-ELM computational model and digital twin data
EW
EAI
DOI: 10.4108/ew.4896
Abstract
As one of the core equipment of the power grid, the operation status of transformers directly affects the stability and reliability of the power system. To accurately evaluate the remaining life of power grid transformers, a genetic algorithm is applied to optimize the Extreme Learning Machine based on digital twin technology. Then, considering changes in load rate, a residual life prediction model for power grid transformers is constructed. From the results, the error of the research method was within 2℃, with a maximum error of only 1.76℃. The research model converged with a fitness value of 0.04 at 150 iterations. It showed good predictive performance for hot spot temperatures under different load rates, with an average accuracy of 99.97%. Compared with backpropagation models and extreme learning machine models, the research method improved accuracy by 2.85% and 1.01%, respectively, with small and stable prediction errors. It verified the superiority of the research model, indicating that the research method can improve the accuracy of predicting the remaining life for power grid transformers. By monitoring the operation status of transformers in real-time, potential faults can be detected in a timely manner. The maintenance and replacement can be carried out in advance to avoid power outages caused by equipment damage. In addition, the research can provide reference for the planning and design of power systems, and support the stability and reliability of power systems.
Copyright © 2024 X. Wang et al., licensed to EAI. This is an open access article distributed under the terms of the CC BY-NC-SA 4.0, which permits copying, redistributing, remixing, transformation, and building upon the material in any medium so long as the original work is properly cited.