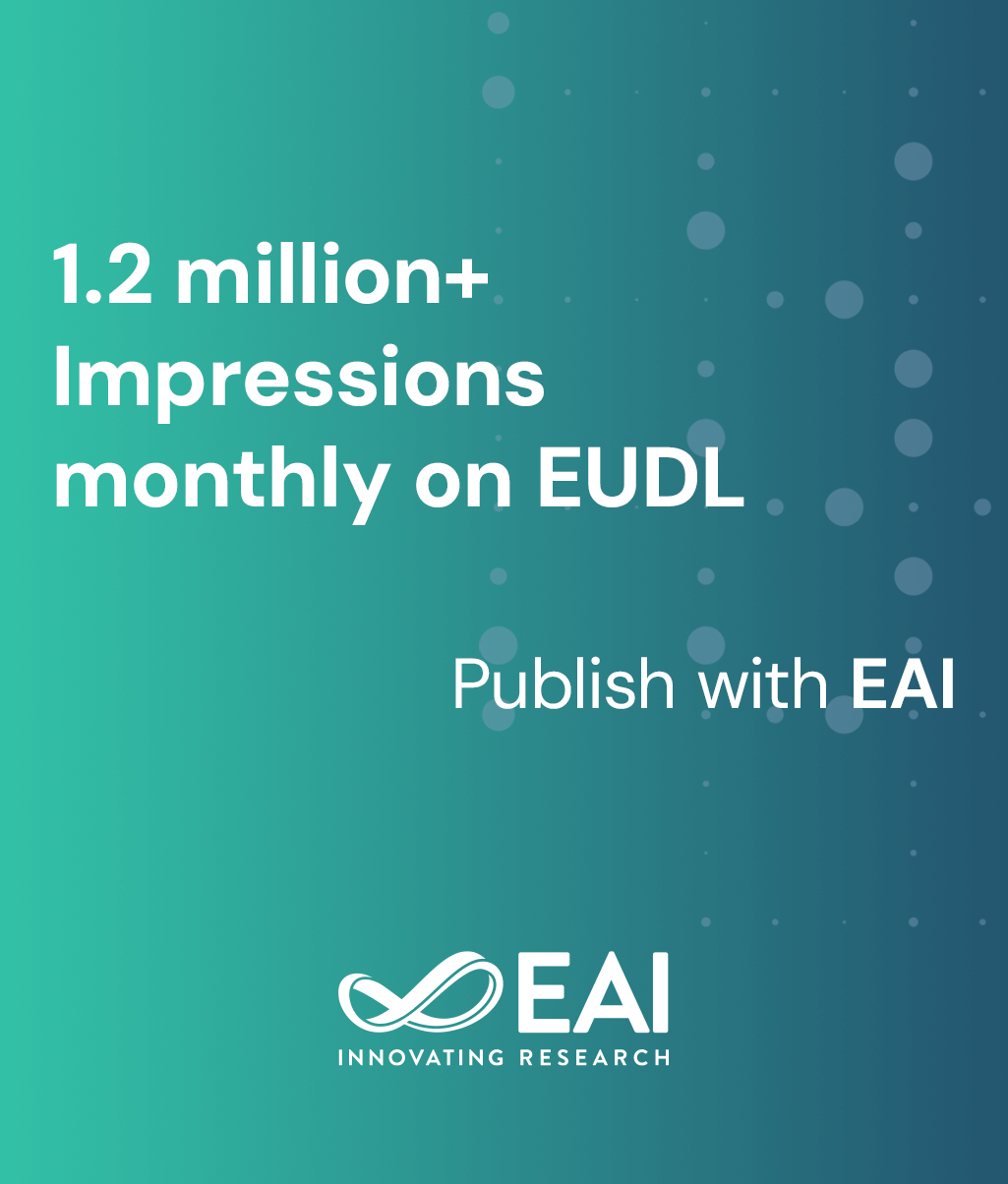
Research Article
Comparison between LightGBM and other ML algorithms in PV fault classification
@ARTICLE{10.4108/ew.4865, author={Paulo Monteiro and Jos\^{e} Lino and Rui Esteves Ara\^{u}jo and Louelson Costa}, title={Comparison between LightGBM and other ML algorithms in PV fault classification}, journal={EAI Endorsed Transactions on Energy Web}, volume={11}, number={1}, publisher={EAI}, journal_a={EW}, year={2024}, month={1}, keywords={Photovoltaic faults, Fault diagnostics, Fault classification, Data-driven, Machine Learning}, doi={10.4108/ew.4865} }
- Paulo Monteiro
José Lino
Rui Esteves Araújo
Louelson Costa
Year: 2024
Comparison between LightGBM and other ML algorithms in PV fault classification
EW
EAI
DOI: 10.4108/ew.4865
Abstract
In this paper, the performance analysis of Machine Learning (ML) algorithms for fault analysis in photovoltaic (PV) plants, is given for different algorithms. To make the comparison more relevant, this study is made based on a real dataset. The goal was to use electric and environmental data from a PV system to provide a framework for analysing, comparing, and discussing five ML algorithms, such as: Multilayer Perceptron (MLP), Decision Tree (DT), K-Nearest Neighbors (KNN), Support Vector Machine (SVM) and Light Gradient Boosting Machine (LightGBM). The research findings suggest that an algorithm from the Gradient Boosting family called LightGBM can offer comparable or better performance in fault diagnosis for PV system.
Copyright © 2024 P. Monteiro et al., licensed to EAI. This is an open access article distributed under the terms of the CC BY-NC-SA 4.0, which permits copying, redistributing, remixing, transformation, and building upon the material in any medium so long as the original work is properly cited.