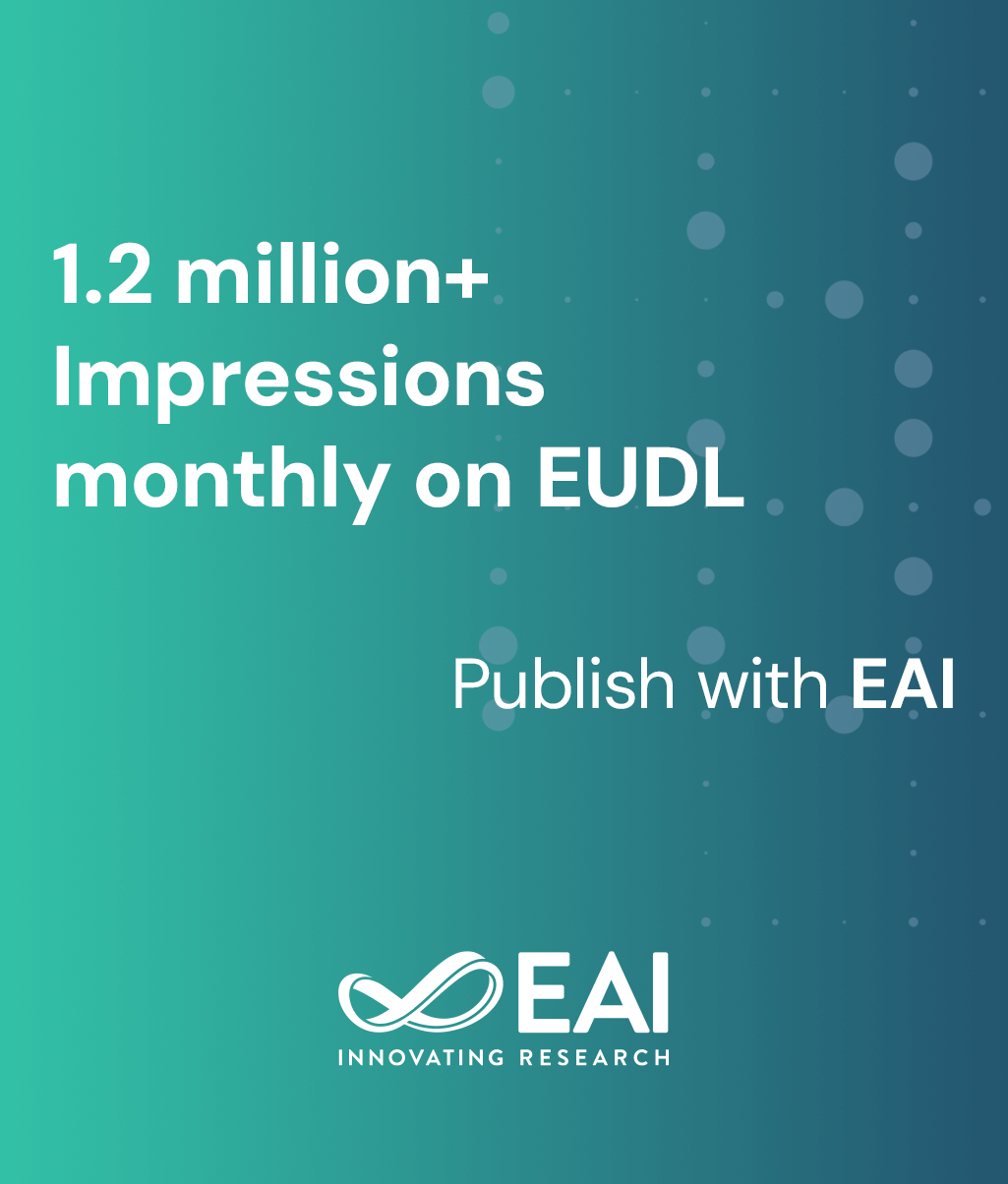
Research Article
Effect of a resampling method on the effectiveness of multi-layer neural network models in PV power forecasting
@ARTICLE{10.4108/ew.3705, author={Abderrahman Bensalem and Toual Belgacem and Abdellah Kouzou and Zakaria Belboul}, title={Effect of a resampling method on the effectiveness of multi-layer neural network models in PV power forecasting}, journal={EAI Endorsed Transactions on Energy Web}, volume={11}, number={1}, publisher={EAI}, journal_a={EW}, year={2024}, month={12}, keywords={Photovoltaic, MNN, Forecasting, Resampling Method}, doi={10.4108/ew.3705} }
- Abderrahman Bensalem
Toual Belgacem
Abdellah Kouzou
Zakaria Belboul
Year: 2024
Effect of a resampling method on the effectiveness of multi-layer neural network models in PV power forecasting
EW
EAI
DOI: 10.4108/ew.3705
Abstract
The primary aim of this study was to explore the impact of employing the K-fold Cross Validation resampling method in contrast to the hold-out set validation approach on the efficacy of forecasting models utilizing Multi-layer Neural Networks (MNN) for predicting photovoltaic (PV) output power. Real data sourced from southern Algeria was utilized for this purpose. The performance of various configurations of MNN models, with differing learning rate values, was evaluated using the coefficient of variation of Root Mean Square Error (CV(RMSE)). The findings consistently demonstrate that models developed using K-fold Cross Validation exhibited superior performance across most scenarios. These results underscore the potential advantages of leveraging such resampling techniques in terms of both generalization and robustness of forecasting models.
Copyright © 2024 Bensalem et al., licensed to EAI. This is an open access article distributed under the terms of the CC BY-NC-SA 4.0, which permits copying, redistributing, remixing, transformation, and building upon the material in any medium so long as the original work is properly cited.