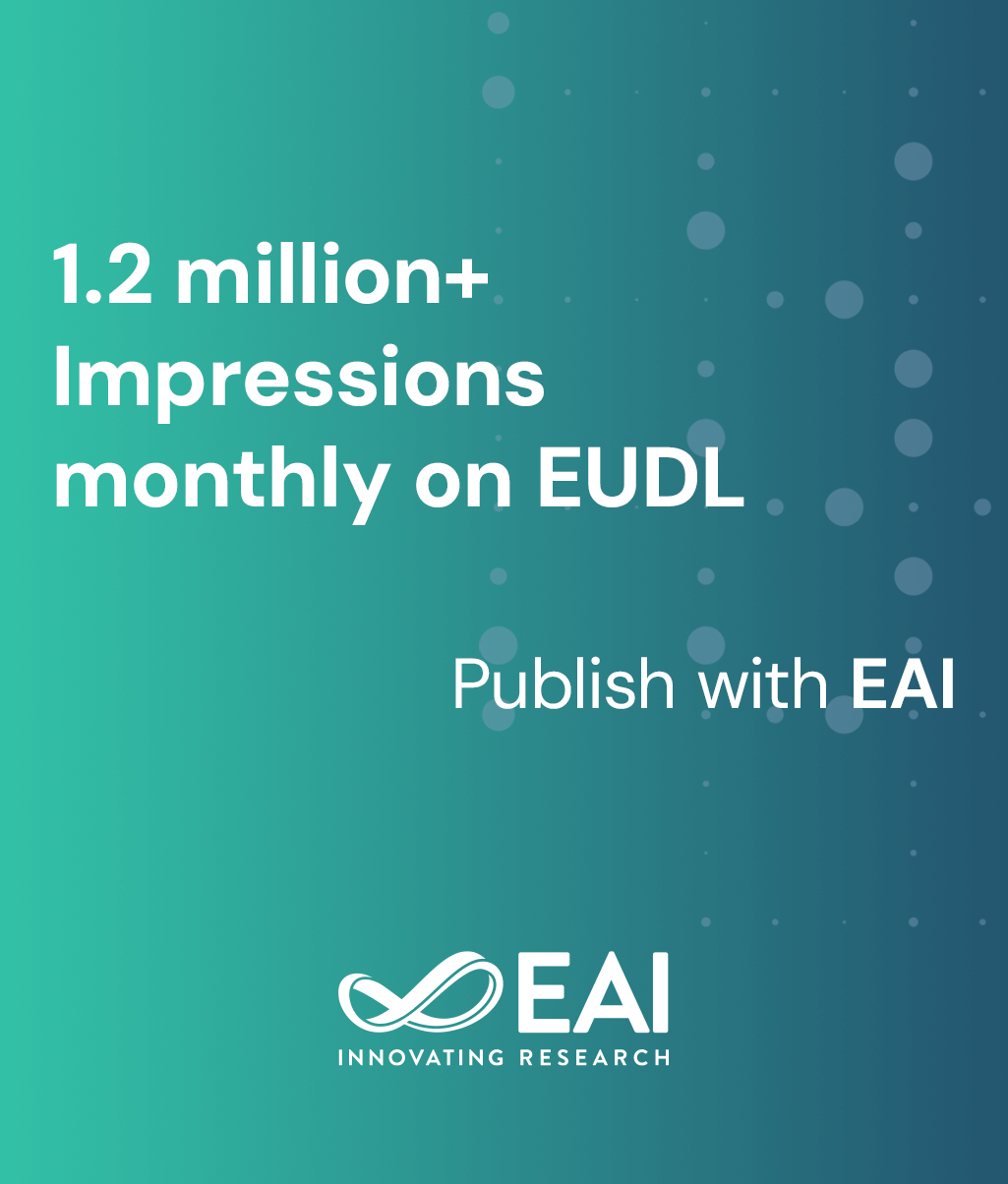
Research Article
Analysis and Design of Standard Knowledge Service System based on Deep Learning
@ARTICLE{10.4108/eetsis.v9i6.2637, author={Yuzhong Zhou and Zhengping Lin and Liang Tu and Junkai Huang and Zifeng Zhang}, title={Analysis and Design of Standard Knowledge Service System based on Deep Learning}, journal={EAI Endorsed Transactions on Scalable Information Systems}, volume={10}, number={2}, publisher={EAI}, journal_a={SIS}, year={2022}, month={10}, keywords={Deep learning, standard knowledge service system, knowledge classification, convolution neural network (CNN)}, doi={10.4108/eetsis.v9i6.2637} }
- Yuzhong Zhou
Zhengping Lin
Liang Tu
Junkai Huang
Zifeng Zhang
Year: 2022
Analysis and Design of Standard Knowledge Service System based on Deep Learning
SIS
EAI
DOI: 10.4108/eetsis.v9i6.2637
Abstract
The development of information technology has changed the mode of communication of social information, and this change has put forward new requirements on the contents, methods and even objects of information science research. Knowledge service in the information service process can extract knowledge and information content from various explicit and implicit knowledge resources according to people’s needs, build knowledge networks, and provide knowledge content or solutions for users’ problems. Hence, it is very important to investigate how to analyze and design the advanced standard knowledge service system based on deep learning. To this end, we firstly introduce the typical deep learning networks of convolutional neural network (CNN) for the knowledge service system, and then employ the CNN to implement the knowledge classification based on deep learning. Finally, some simulation results on the knowledge service system are presented to validate the proposed studies in this paper.
Copyright © 2022 Yuzhong Zhou et al., licensed to EAI. This is an open access article distributed under the terms of the CC BY-NC-SA 4.0, which permits copying, redistributing, remixing, transformation, and building upon the material in any medium so long as the original work is properly cited.