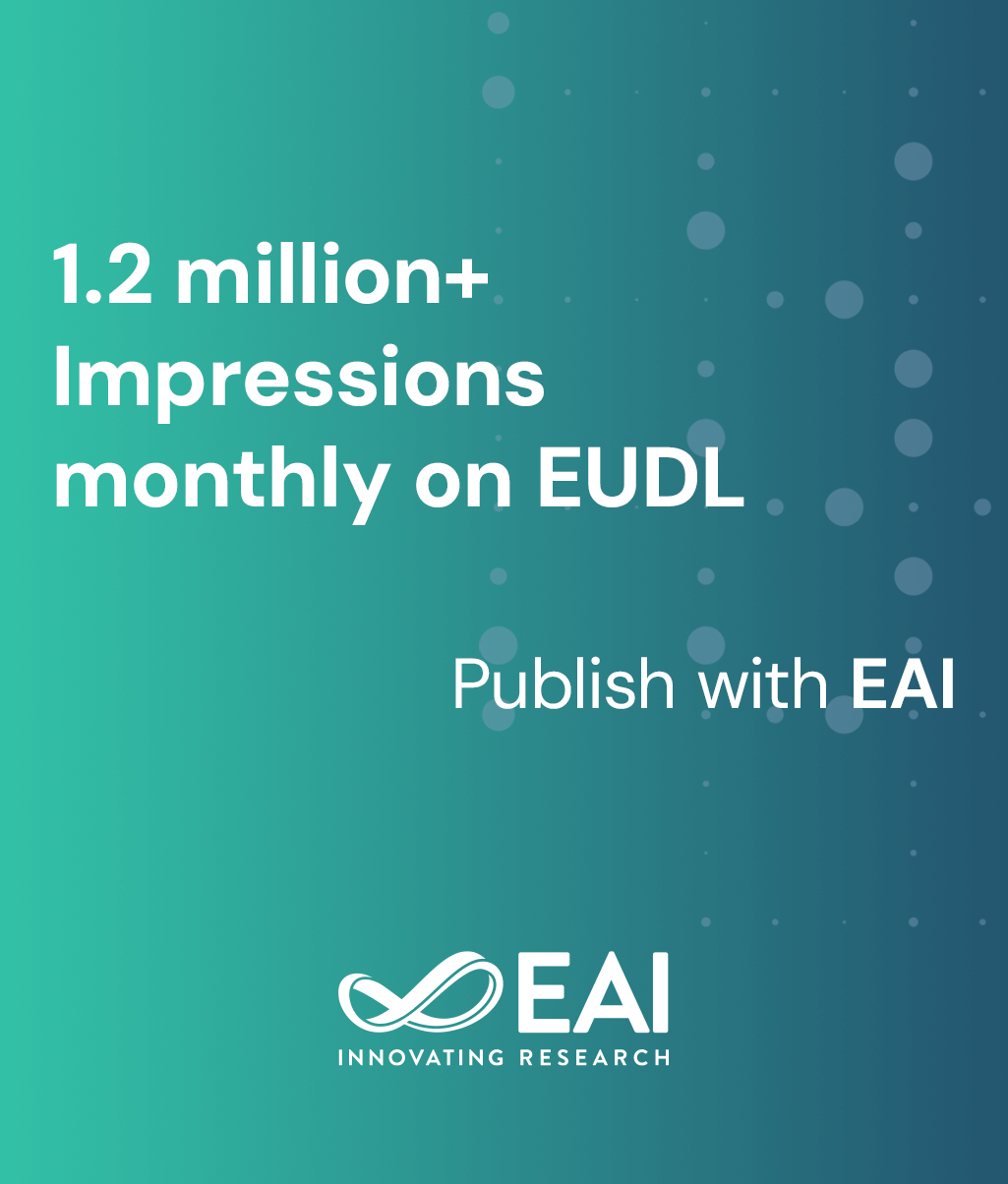
Research Article
Real-time Distributed Computing Model of Low-Voltage Flow Data in Digital Power Grid under Autonomous and Controllable Environments
@ARTICLE{10.4108/eetsis.v10i4.3166, author={Hancong Huangfu and Yongcai Wang and Zhenghao Qian and Yanning Shao}, title={Real-time Distributed Computing Model of Low-Voltage Flow Data in Digital Power Grid under Autonomous and Controllable Environments}, journal={EAI Endorsed Transactions on Scalable Information Systems}, volume={10}, number={5}, publisher={EAI}, journal_a={SIS}, year={2023}, month={6}, keywords={Distribute computing, wireless offloading, outage probability, performance analysis}, doi={10.4108/eetsis.v10i4.3166} }
- Hancong Huangfu
Yongcai Wang
Zhenghao Qian
Yanning Shao
Year: 2023
Real-time Distributed Computing Model of Low-Voltage Flow Data in Digital Power Grid under Autonomous and Controllable Environments
SIS
EAI
DOI: 10.4108/eetsis.v10i4.3166
Abstract
Motivated by the progress in artificial intelligence and edge computing, this paper proposes a real-time distributed computing model for low-voltage flow data in digital power grids under autonomous and controllable environments. The model utilizes edge computing through wireless offloading to efficiently process and analyze data generated by low-voltage devices in the power grid. Firstly, we evaluate the performance of the system under consideration by measuring its outage probability, utilizing both the received signal-to-noise ratio (SNR) and communication and computing latency. Subsequently, we analyze the system’s outage probability by deriving an analytical expression. To this end, we utilize the Gauss-Chebyshev approximation to provide an approximate closed-form expression. The results of our experimental evaluation demonstrate the effectiveness of the proposed model in achieving real-time processing of low-voltage flow data in digital power grids. Our model provides an efficient and practical solution for the processing of low-voltage flow data, making it a valuable contribution to the field of digital power grids.
Copyright © 2023 Hancong Huangfu et al., licensed to EAI. This is an open access article distributed under the terms of the CC BY-NC-SA 4.0, which permits copying, redistributing, remixing, transformation, and building upon the material in any medium so long as the original work is properly cited.