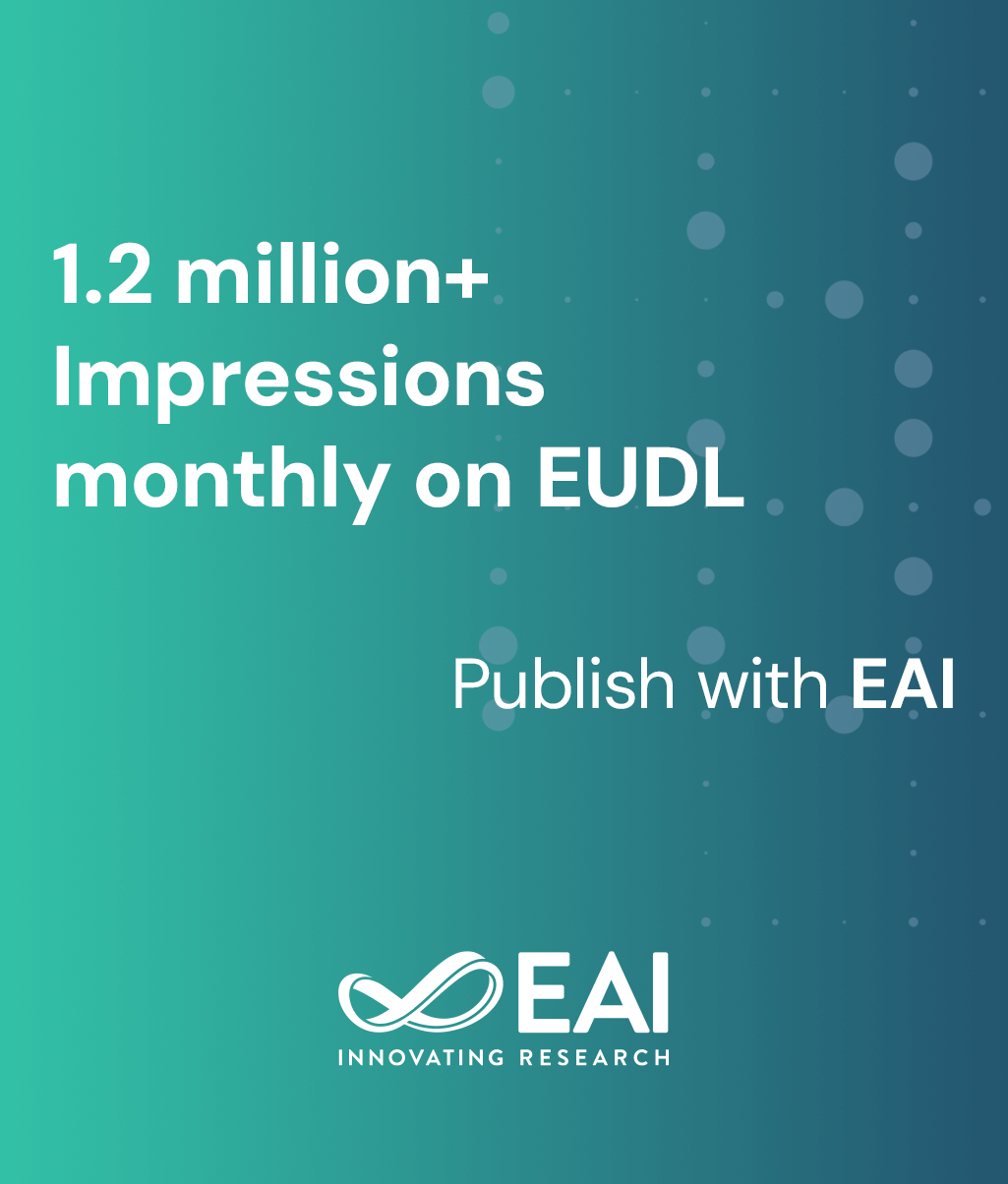
Research Article
Ensemble Deep Learning Algorithm for Forecasting of Rice Crop Yield based on Soil Nutrition Levels
@ARTICLE{10.4108/eetsis.v10i3.2610, author={Chandraprabha M. and Rajesh Kumar Dhanraj}, title={Ensemble Deep Learning Algorithm for Forecasting of Rice Crop Yield based on Soil Nutrition Levels}, journal={EAI Endorsed Transactions on Scalable Information Systems}, volume={10}, number={4}, publisher={EAI}, journal_a={SIS}, year={2023}, month={5}, keywords={Support Vector Machine, Deep Neural Network, Deep Belief Network, ensemble learning, Model Agnostic Meta Learning}, doi={10.4108/eetsis.v10i3.2610} }
- Chandraprabha M.
Rajesh Kumar Dhanraj
Year: 2023
Ensemble Deep Learning Algorithm for Forecasting of Rice Crop Yield based on Soil Nutrition Levels
SIS
EAI
DOI: 10.4108/eetsis.v10i3.2610
Abstract
Agriculture is critical to the development of a growing country like India. For the vast majority of the population, agriculture is their primary source of income. Crop yield estimates that are accurate and timely can give crucial information for determining agriculture policy and making investments. Crop yield forecasting and prediction will boost agricultural productivity, while crop rotation will improve soil fertility. When farmers are unaware of the soil nutrition and composition, crop yields are reduced to a minimum. To address these concerns, the proposed methodology creates an ensemble deep learning system for predicting rice crop production based on soil nutrition levels. Soil nutrients and crop production statistics are taken as the input for the proposed method. The soil nutrients dataset contains different nutrients level in the soil. Crop production statistics are the amount of crop yield in a particular area. Normalization and mean of the attribute techniques are used as pre-processing approaches to fill the missing values in the input dataset. The suggested process utilizes a stacking-based ensemble deep learning strategy termed Model Agnostic Meta-Learning (MAML) for classification. MAML receives output from three different classifiers, including Deep Neural Network (DNN), Deep Belief Network (DBN) and Support Vector Machine (SVM). Then the MAML produce the final output as how much amount of rice crop is predicted in the particular soil. The proposed method provides better accuracy of 89.5%. Thus the designed model predicted the crop yield prediction in an effective manner.
Copyright © 2023 M. Chandraprabha et al., licensed to EAI. This is an open access article distributed under the terms of the CC BYNC-SA 4.0, which permits copying, redistributing, remixing, transformation, and building upon the material in any medium so long as the original work is properly cited.