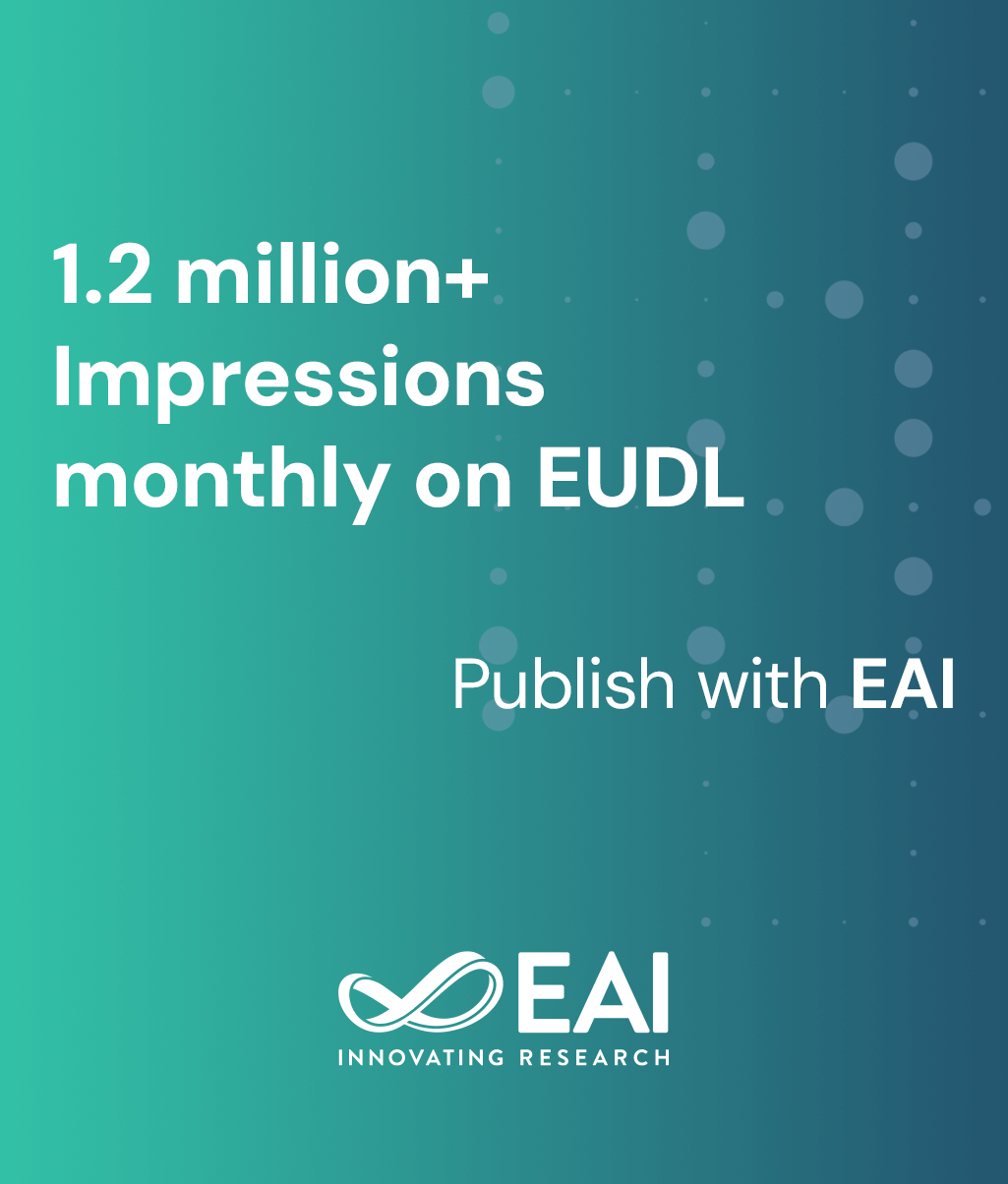
Research Article
A New Suppression-based Possibilistic Fuzzy c-means Clustering Algorithm
@ARTICLE{10.4108/eetsis.v10i3.2057, author={Jyoti Arora and Meena Tushir and Shivank Kumar Dadhwal}, title={A New Suppression-based Possibilistic Fuzzy c-means Clustering Algorithm}, journal={EAI Endorsed Transactions on Scalable Information Systems}, volume={10}, number={3}, publisher={EAI}, journal_a={SIS}, year={2023}, month={1}, keywords={fuzzy c-means, possibilistic c-means, possibilistic fuzzy c-means, suppression possibilistic fuzzy c-means}, doi={10.4108/eetsis.v10i3.2057} }
- Jyoti Arora
Meena Tushir
Shivank Kumar Dadhwal
Year: 2023
A New Suppression-based Possibilistic Fuzzy c-means Clustering Algorithm
SIS
EAI
DOI: 10.4108/eetsis.v10i3.2057
Abstract
Possibilistic fuzzy c-means (PFCM) is one of the most widely used clustering algorithm that solves the noise sensitivity problem of Fuzzy c-means (FCM) and coincident clusters problem of possibilistic c-means (PCM). Though PFCM is a highly reliable clustering algorithm but the efficiency of the algorithm can be further improved by introducing the concept of suppression. Suppression-based algorithms employ the winner and non-winner based suppression technique on the datasets, helping in performing better classification of real-world datasets into clusters. In this paper, we propose a suppression-based possibilistic fuzzy c-means clustering algorithm (SPFCM) for the process of clustering. The paper explores the performance of the proposed methodology based on number of misclassifications for various real datasets and synthetic datasets and it is found to perform better than other clustering techniques in the sequel, i.e., normal as well as suppression-based algorithms. The SPFCM is found to perform more efficiently and converges faster as compared to other clustering techniques.
Copyright © 2023 J. Arora et al., licensed to EAI. This is an open access article distributed under the terms of the CC BY-NC-SA 4.0, which permits copying, redistributing, remixing, transformation, and building upon the material in any medium so long as the original work is properly cited.