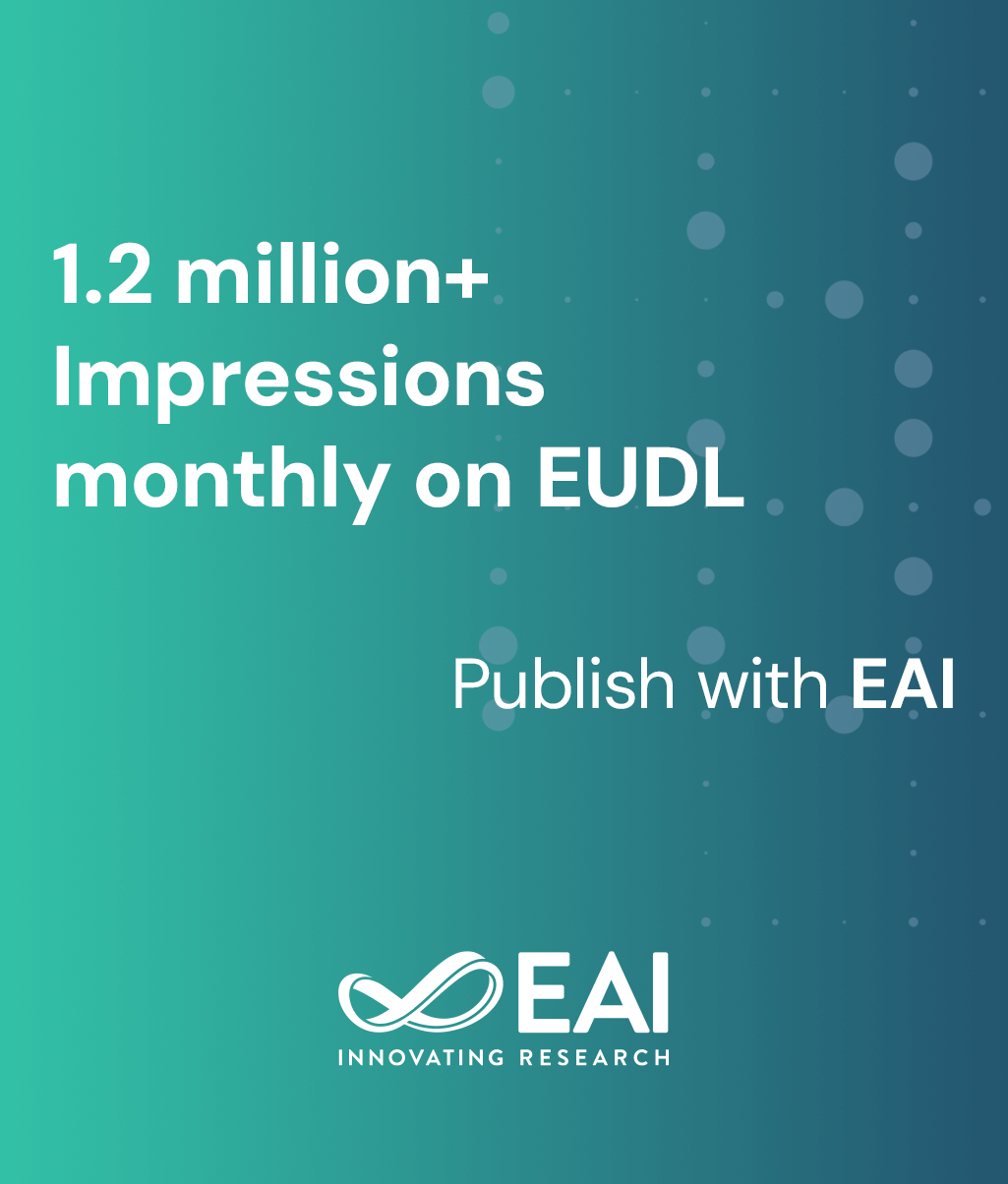
Research Article
Mixed Bayesian Stackelberg Strategies for Robust Adversarial Classifiers
@ARTICLE{10.4108/eetsis.7635, author={Hakeem Quadri}, title={Mixed Bayesian Stackelberg Strategies for Robust Adversarial Classifiers}, journal={EAI Endorsed Transactions on Scalable Information Systems}, volume={12}, number={1}, publisher={EAI}, journal_a={SIS}, year={2025}, month={4}, keywords={Convolution neural networks (CNN), Game theory, Stackelberg games, Mixed strategies, Adversarial Training}, doi={10.4108/eetsis.7635} }
- Hakeem Quadri
Year: 2025
Mixed Bayesian Stackelberg Strategies for Robust Adversarial Classifiers
SIS
EAI
DOI: 10.4108/eetsis.7635
Abstract
Deep neural networks (DNNs) have achieved state-of-the-art performance in classification tasks; however, they are susceptible to small perturbations that are seemingly imperceptible to the human eye but are enough to fool the network into misclassifying images. To develop more robust DNNs against adversarial attacks, research methods have focused on exploring the interaction between a machine learning classifier and a single adversary. However, these methods do not adequately model the real-world scenarios in which these classifiers are deployed. In this research paper, we address this gap and propose an adversarial learning algorithm with multiple adversaries using Bayesian Stackelberg games to model the interaction between the learner and multiple adversaries. We conclude that the nested Bayesian Stackelberg method is a useful strategy for developing adversarial learning algorithms to improve the robustness of DNNs. This strategy can serve as a benchmark in future defense attempts to create DNNs that resist adversarial attacks.
Copyright © 2024 H. Quadri, licensed to EAI. This is an open access article distributed under the terms of the Creative Commons Attribution license (http://creativecommons.org/licenses/by/4.0/), which permits unlimited use, distribution and reproduction in any medium so long as the original work is properly cited.