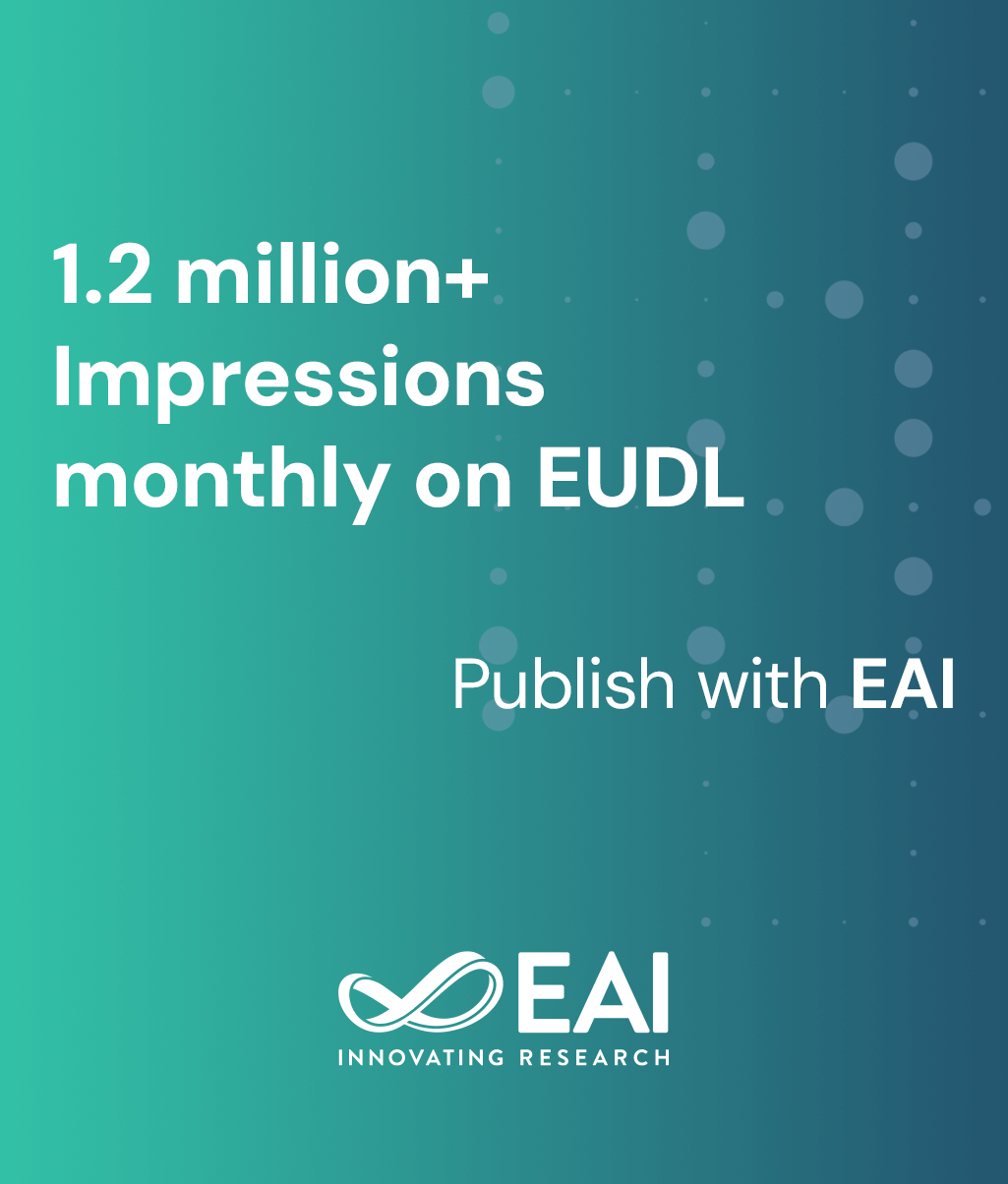
Research Article
Evaluation of a Microcontroller-based Smart Wearable Device in College Students' Sports Forging Application
@ARTICLE{10.4108/eetsis.5857, author={Yong Che and Kaixuan Che and Qinlong Li}, title={Evaluation of a Microcontroller-based Smart Wearable Device in College Students' Sports Forging Application}, journal={EAI Endorsed Transactions on Scalable Information Systems}, volume={11}, number={5}, publisher={EAI}, journal_a={SIS}, year={2024}, month={5}, keywords={smart wearable devices, university students' sports forging, application evaluation methods, zebra optimisation algorithm, convolutional neural network}, doi={10.4108/eetsis.5857} }
- Yong Che
Kaixuan Che
Qinlong Li
Year: 2024
Evaluation of a Microcontroller-based Smart Wearable Device in College Students' Sports Forging Application
SIS
EAI
DOI: 10.4108/eetsis.5857
Abstract
INTRODUCTION: The widespread use of smart wearable devices in various fields, including healthcare and sports, underscores the importance of their application in enhancing physical exercise among college students. Recent advancements in technology have facilitated the development of sophisticated methods to assess and predict physical activity outcomes, making their evaluation increasingly critical. OBJECTIVES: This study aims to develop a reliable assessment model for smart wearable devices used in college students' sports activities. The objective is to accurately predict and evaluate the effectiveness of these devices in improving students' physical health and promoting lifelong sports habits. Ultimately, the research seeks to integrate advanced computational methods to enhance the accuracy of physical exercise assessments. METHODS: The research introduces a novel assessment model that combines a zebra behavior-based heuristic optimization algorithm with a convolutional neural network (CNN). By analyzing user behavior data from wearable devices, the model constructs an evaluation index system tailored for college sports activities. The approach optimizes the parameters of the CNN using the zebra optimization algorithm, ensuring enhanced prediction accuracy. RESULTS: The evaluation model demonstrated high accuracy, with a significant improvement in predicting the outcomes of physical exercises among college students. Comparative analyses with traditional methods revealed that the new model reduced prediction errors and increased real-time performance metrics. Specifically, the model achieved a lower root mean square error (RMSE) in simulation tests, indicating more precise assessments. Figures and statistical data provided in the study illustrate the model's superior performance across various parameters. CONCLUSION: The developed assessment model significantly advances the application of smart wearable devices in monitoring and enhancing college students' physical activities. By integrating cutting-edge algorithms, the study not only improves the accuracy of exercise assessments but also contributes to the broader understanding of technology's role in health and fitness education. Future research could further refine this model by incorporating additional sensors and data points to expand its applicability and robustness.
Copyright © 2024 Che et al., licensed to EAI. This is an open access article distributed under the terms of the CC BY-NC-SA 4.0, which permits copying, redistributing, remixing, transformation, and building upon the material in any medium so long as the original work is properly cited.