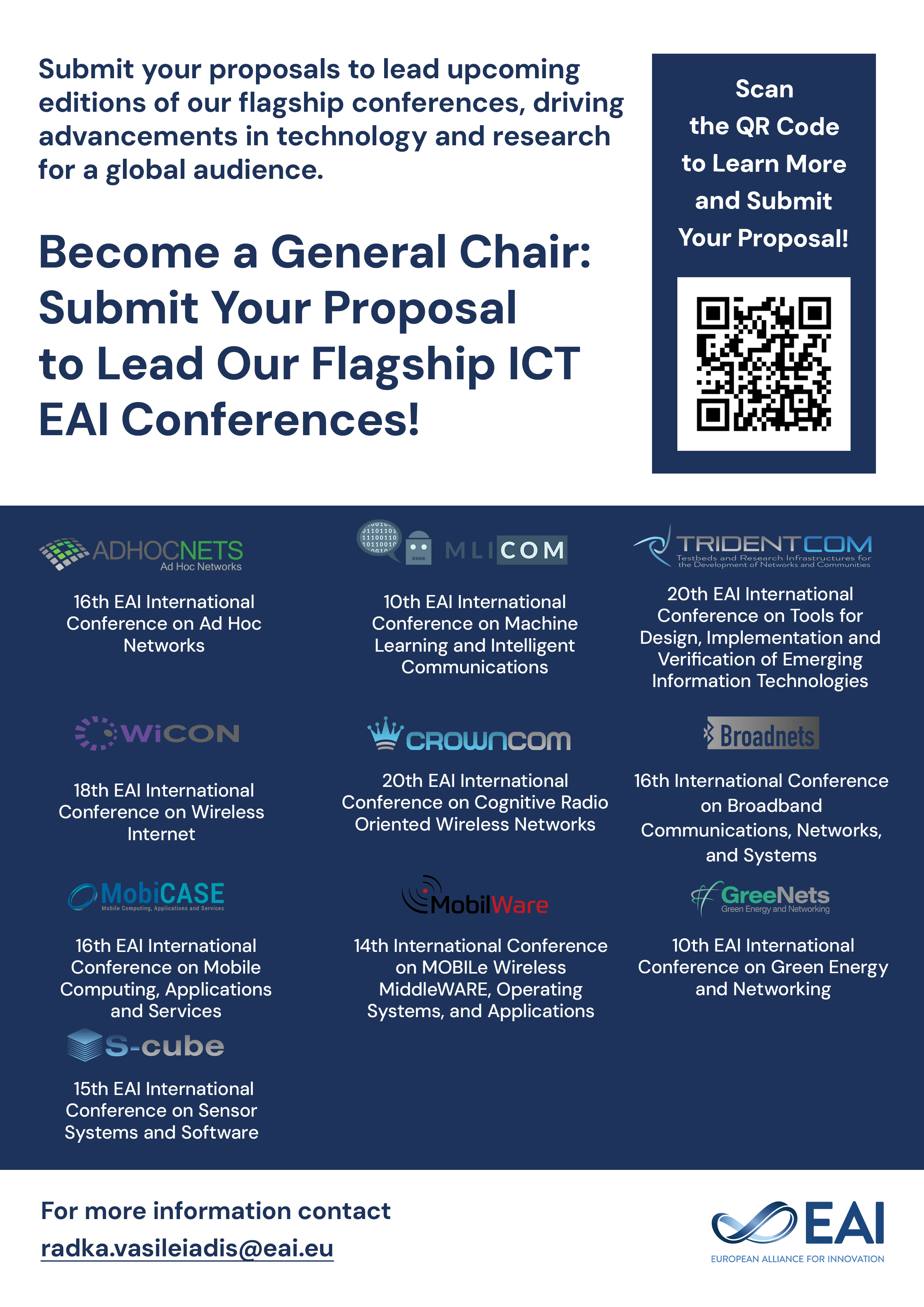
Research Article
Sentinel Shield: Leveraging ConvLSTM and Elephant Herd Optimization for Advanced Network Intrusion Detection
@ARTICLE{10.4108/eetsis.5737, author={Aparna Tiwari and Dinesh Kumar}, title={Sentinel Shield: Leveraging ConvLSTM and Elephant Herd Optimization for Advanced Network Intrusion Detection}, journal={EAI Endorsed Transactions on Scalable Information Systems}, volume={11}, number={6}, publisher={EAI}, journal_a={SIS}, year={2024}, month={6}, keywords={Traffic Prediction, ConvLSTM, EHO}, doi={10.4108/eetsis.5737} }
- Aparna Tiwari
Dinesh Kumar
Year: 2024
Sentinel Shield: Leveraging ConvLSTM and Elephant Herd Optimization for Advanced Network Intrusion Detection
SIS
EAI
DOI: 10.4108/eetsis.5737
Abstract
Given the escalating intricacy of network environments and the rising level of sophistication in cyber threats, there is an urgent requirement for resilient and effective network intrusion detection systems (NIDS). This document presents an innovative NIDS approach that utilizes Convolutional Long Short-Term Memory (ConvLSTM) networks and Elephant Herd Optimization (EHO) to achieve precise and timely intrusion detection. Our proposed model combines the strengths of ConvLSTM, which can effectively capture spatiotemporal dependencies in network traffic data, and EHO, which allow the model to focus on relevant information while filtering out noise. To achieve this, we first preprocess network traffic data into sequential form and use ConvLSTM layers to learn both spatial and temporal features. Subsequently, we introduce Elephant Herd Optimization that dynamically assigns different weights to different parts of the input data, emphasizing the regions most likely to contain malicious activity. To evaluate the effectiveness of our approach, we conducted extensive experiments on publicly available network intrusion CICIDS2017 Dataset. The experimental results demonstrate the efficacy of the proposed approach (Accuracy = 99.98%), underscoring its potential to revolutionize modern network intrusion detection and proactively safeguard digital assets.
Copyright © 2024 Author et al., licensed to EAI. This is an open access article distributed under the terms of theCC BY-NC-SA 4.0, which permits copying, redistributing, remixing, transformation, and building upon the material in any medium so long as the original work is properly cited.