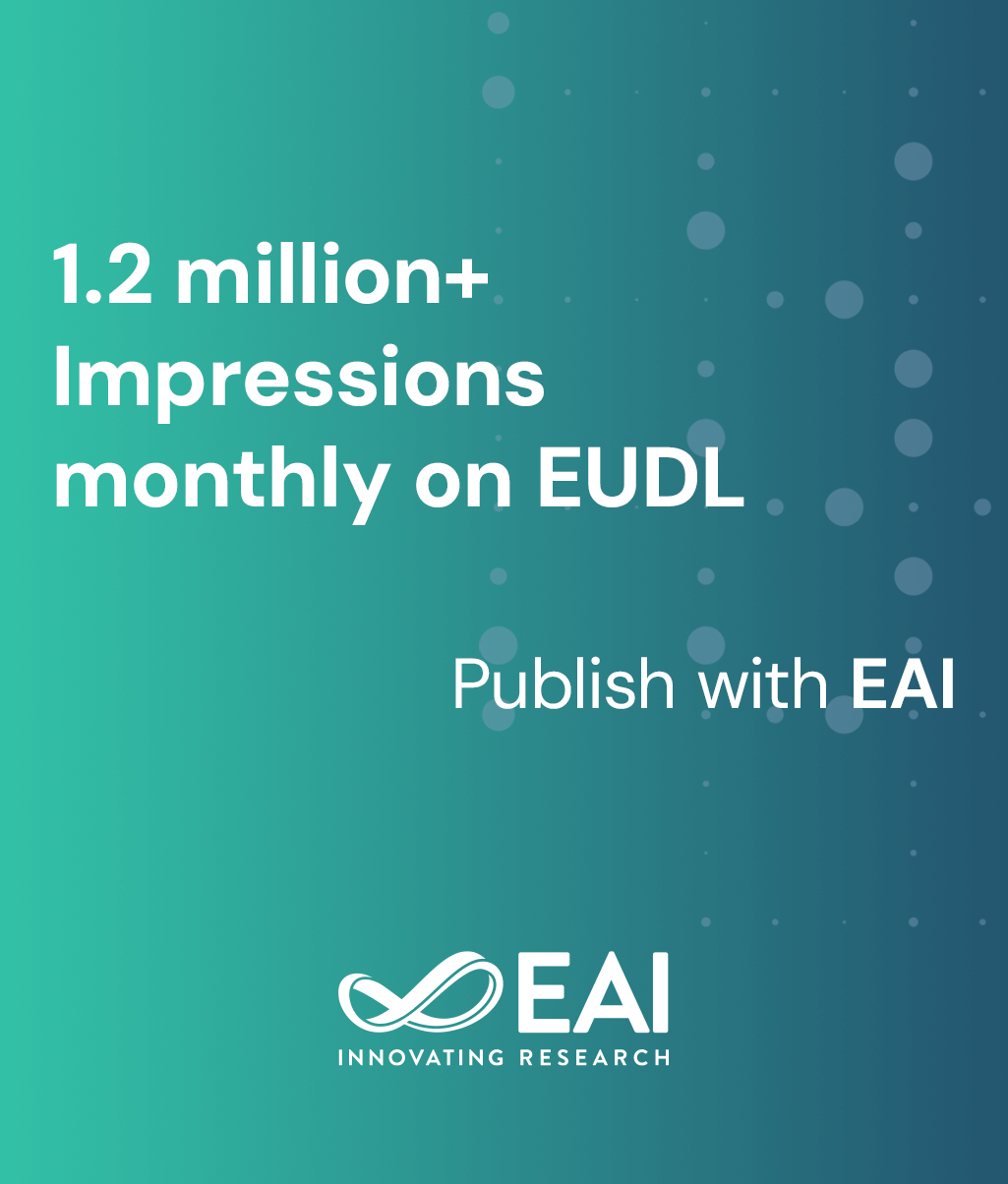
Research Article
Exploring the Impact of Mismatch Conditions, Noisy Backgrounds, and Speaker Health on Convolutional Autoencoder-Based Speaker Recognition System with Limited Dataset
@ARTICLE{10.4108/eetsis.5697, author={Arundhati Niwatkar and Yuvraj Kanse and Ajay Kumar Kushwaha}, title={Exploring the Impact of Mismatch Conditions, Noisy Backgrounds, and Speaker Health on Convolutional Autoencoder-Based Speaker Recognition System with Limited Dataset}, journal={EAI Endorsed Transactions on Scalable Information Systems}, volume={11}, number={6}, publisher={EAI}, journal_a={SIS}, year={2024}, month={4}, keywords={MPCC, pitch, jitter, shimmer, convolutional autoencoder}, doi={10.4108/eetsis.5697} }
- Arundhati Niwatkar
Yuvraj Kanse
Ajay Kumar Kushwaha
Year: 2024
Exploring the Impact of Mismatch Conditions, Noisy Backgrounds, and Speaker Health on Convolutional Autoencoder-Based Speaker Recognition System with Limited Dataset
SIS
EAI
DOI: 10.4108/eetsis.5697
Abstract
This paper presents a novel approach to enhance the success rate and accuracy of speaker recognition and identification systems. The methodology involves employing data augmentation techniques to enrich a small dataset with audio recordings from five speakers, covering both male and female voices. Python programming language is utilized for data processing, and a convolutional autoencoder is chosen as the model. Spectrograms are used to convert speech signals into images, serving as input for training the autoencoder. The developed speaker recognition system is compared against traditional systems relying on the MFCC feature extraction technique. In addition to addressing the challenges of a small dataset, the paper explores the impact of a "mismatch condition" by using different time durations of the audio signal during both training and testing phases. Through experiments involving various activation and loss functions, the optimal pair for the small dataset is identified, resulting in a high success rate of 92.4% in matched conditions. Traditionally, Mel-Frequency Cepstral Coefficients (MFCC) have been widely used for this purpose. However, the COVID-19 pandemic has drawn attention to the virus's impact on the human body, particularly on areas relevant to speech, such as the chest, throat, vocal cords, and related regions. COVID-19 symptoms, such as coughing, breathing difficulties, and throat swelling, raise questions about the influence of the virus on MFCC, pitch, jitter, and shimmer features. Therefore, this research aims to investigate and understand the potential effects of COVID-19 on these crucial features, contributing valuable insights to the development of robust speaker recognition systems.
Copyright © 2024 A. Niwatkar et al., licensed to EAI. This is an open access article distributed under the terms of the CC BY-NCSA 4.0, which permits copying, redistributing, remixing, transformation, and building upon the material in any medium so long as the original work is properly cited.