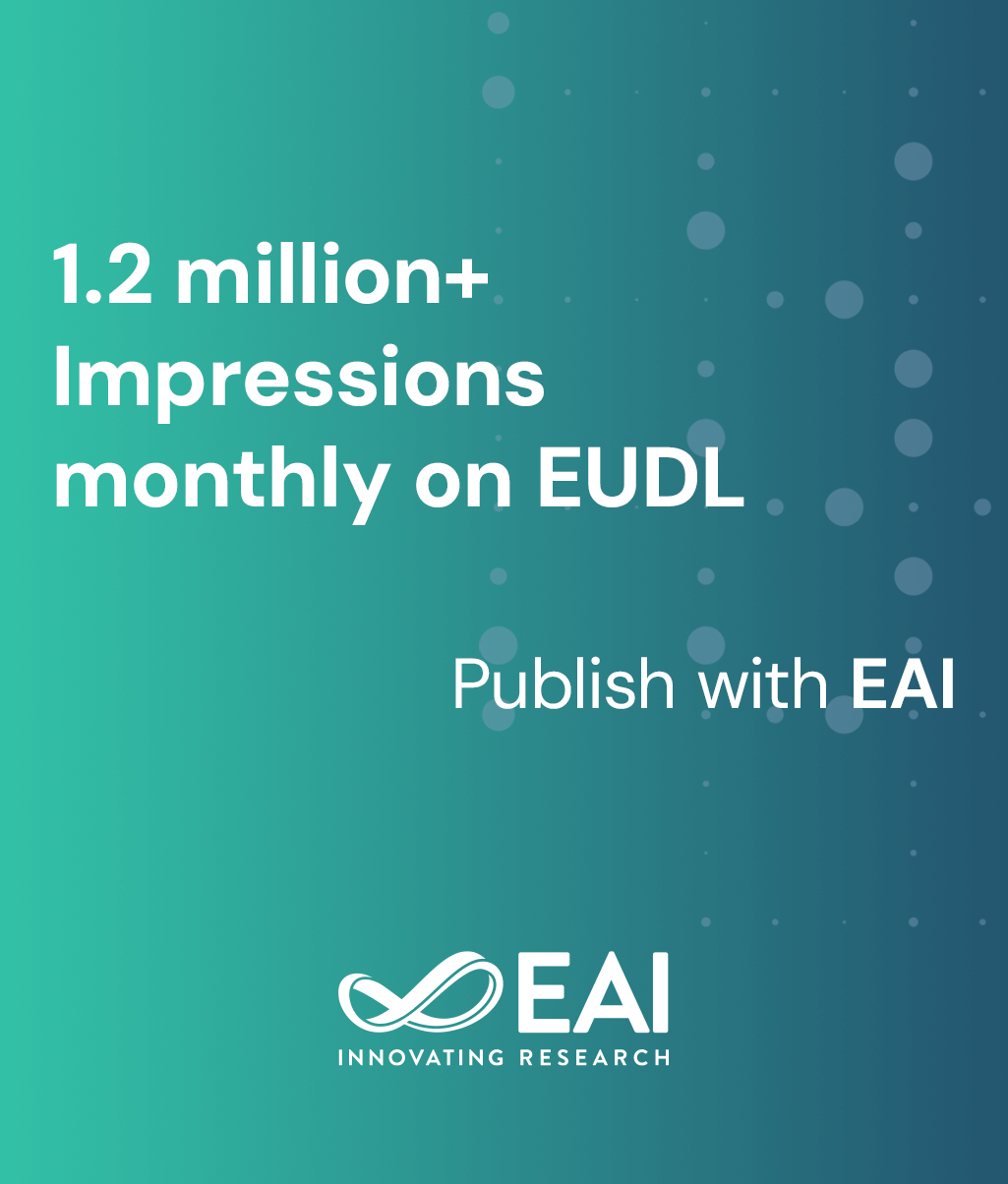
Editorial
Truculent Post Analysis for Hindi Text
@ARTICLE{10.4108/eetsis.5641, author={Mitali Agarwal and Poorvi Sahu and Nisha Singh and Jasleen and Puneet Sinha and Rahul Kumar Singh}, title={Truculent Post Analysis for Hindi Text}, journal={EAI Endorsed Transactions on Scalable Information Systems}, volume={11}, number={6}, publisher={EAI}, journal_a={SIS}, year={2024}, month={4}, keywords={Truculent Post, Hindi language, Sentiment Analysis, BERT, LSTM, NLP}, doi={10.4108/eetsis.5641} }
- Mitali Agarwal
Poorvi Sahu
Nisha Singh
Jasleen
Puneet Sinha
Rahul Kumar Singh
Year: 2024
Truculent Post Analysis for Hindi Text
SIS
EAI
DOI: 10.4108/eetsis.5641
Abstract
INTRODUCTION: With the rise of social media platforms, the prevalence of truculent posts has become a major concern. These posts, which exhibit anger, aggression, or rudeness, not only foster a hostile environment but also have the potential to stir up harm and violence. OBJECTIVES: It is essential to create efficient algorithms for detecting virulent posts so that they can recognise and delete such content from social media sites automatically. In order to improve accuracy and efficiency, this study evaluates the state-of-the-art in truculent post detection techniques and suggests a unique method that combines deep learning and natural language processing. The major goal of the proposed methodology is to successfully regulate hostile social media posts by keeping an eye on them. METHODS: In order to effectively identify the class labels and create a deep-learning method, we concentrated on comprehending the negation words, sarcasm, and irony using the LSTM model. We used multilingual BERT to produce precise word embedding and deliver semantic data. The phrases were also thoroughly tokenized, taking into consideration the Hindi language, thanks to the assistance of the Indic NLP library. RESULTS: The F1 scores for the various classes are given in the "Proposed approach” as follows: 84.22 for non-hostile, 49.26 for hostile, 68.69 for hatred, 49.81 for fake, and 39.92 for offensive CONCLUSION: We focused on understanding the negation words, sarcasm and irony using the LSTM model, to classify the class labels accurately and build a deep-learning strategy.
Copyright © 2024 M. Agarwal et al., licensed to EAI. This is an open access article distributed under the terms of the CC BY-NC-SA 4.0, which permits copying, redistributing, remixing, transformation, and building upon the material in any medium so long as the original work is properly cited.