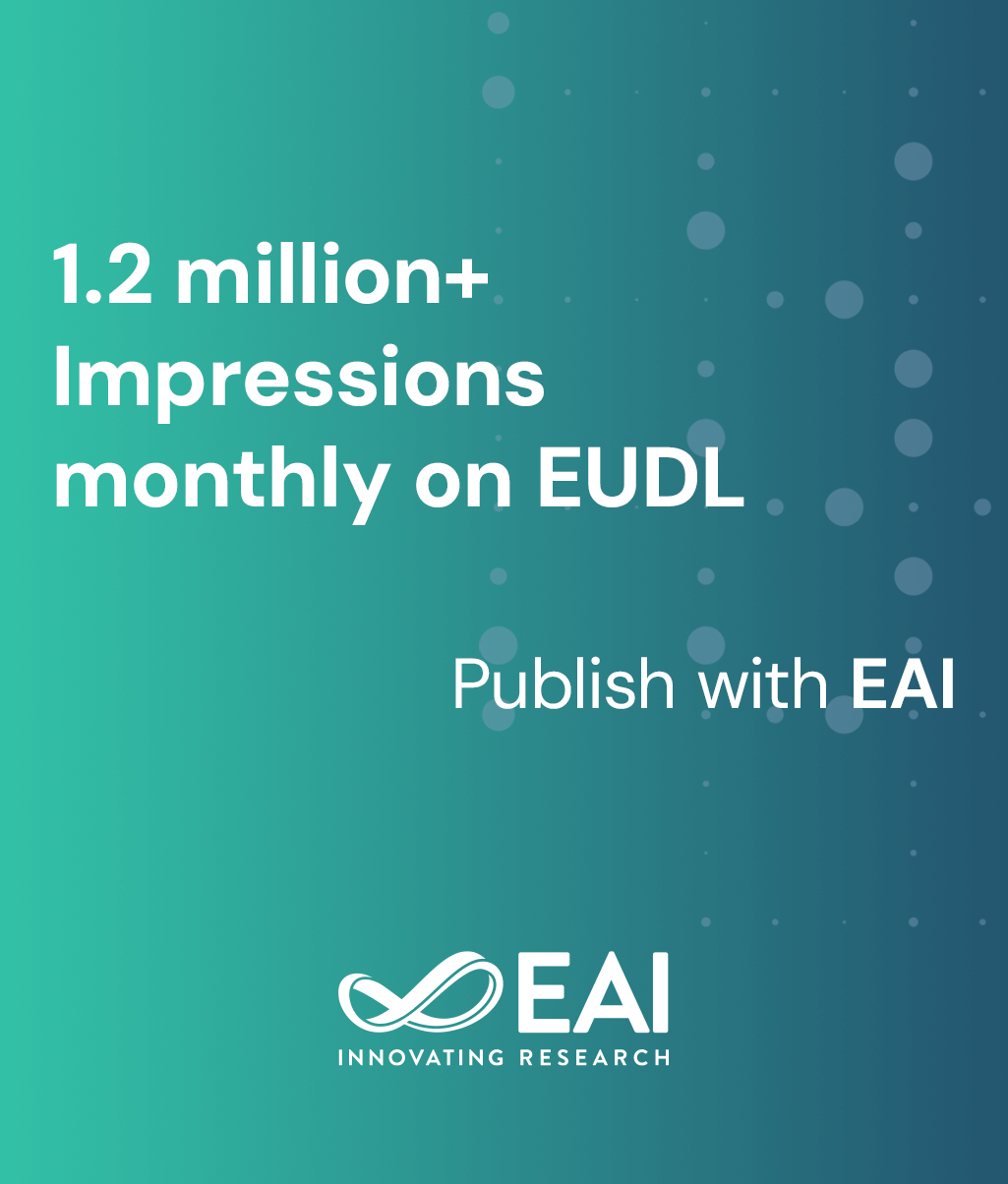
Research Article
Quantum Deep Neural Network Based Classification of Attack Vectors on the Ethereum Blockchain
@ARTICLE{10.4108/eetsis.5572, author={Anand Singh Rajawat and S B Goyal and Manoj Kumar and Saurabh Kumar}, title={Quantum Deep Neural Network Based Classification of Attack Vectors on the Ethereum Blockchain}, journal={EAI Endorsed Transactions on Scalable Information Systems}, volume={11}, number={6}, publisher={EAI}, journal_a={SIS}, year={2024}, month={3}, keywords={Quantum Computing, Quantum Restricted Boltzmann Machine, Ethereum, Blockchain, Attack Vectors, Cybersecurity}, doi={10.4108/eetsis.5572} }
- Anand Singh Rajawat
S B Goyal
Manoj Kumar
Saurabh Kumar
Year: 2024
Quantum Deep Neural Network Based Classification of Attack Vectors on the Ethereum Blockchain
SIS
EAI
DOI: 10.4108/eetsis.5572
Abstract
INTRODUCTION: The implementation of robust security protocols is imperative in light of the exponential growth of blockchain-based platforms such as Ethereum. The importance of developing more effective strategies to detect and counter potential attacks is growing in tandem with the sophistication of the methods employed by attackers. In this study, we present a novel approach that leverages quantum computing to identify and predict attack vectors on the Ethereum blockchain. OBJECTIVES: The primary objective of this study is to suggest an innovative methodology for enhancing the security of Ethereum by leveraging quantum computing. The purpose of this study is to demonstrate that QRBM and QDN are efficient in identifying and predicting security flaws in blockchain transactions. METHODS: We combined methods from quantum computing with social network research approaches. An enormous dataset containing both genuine Ethereum transactions and a carefully chosen spectrum of malicious activity indicative of popular attack vectors was used to train our model, the QRBM. Thanks to the dataset, the QRBM was able to learn to distinguish between typical and out-of-the-ordinary activities. RESULTS: In comparison to more conventional deep learning models, the QRBM showed substantially better accuracy when it came to identifying transaction behaviours. The model's improved scalability and efficiency were made possible by its quantum nature, which is defined by features like entanglement and superposition. Specifically, the QRBM handled non-informative inputs better and solved problems faster. CONCLUSION: This study paves the way for further investigation into quantum-enhanced cybersecurity measures and highlights the promise of quantum neural networks in strengthening the security of blockchain technology. According to our research, quantum computing has the potential to be an essential tool in creating Ethereum-style blockchain security systems that are more advanced, efficient, and resilient.
Copyright © 2024 A. S. Rajawat et al., licensed to EAI. This is an open access article distributed under the terms of the CC BY-NCSA 4.0, which permits copying, redistributing, remixing, transformation, and building upon the material in any medium so long as the original work is properly cited.