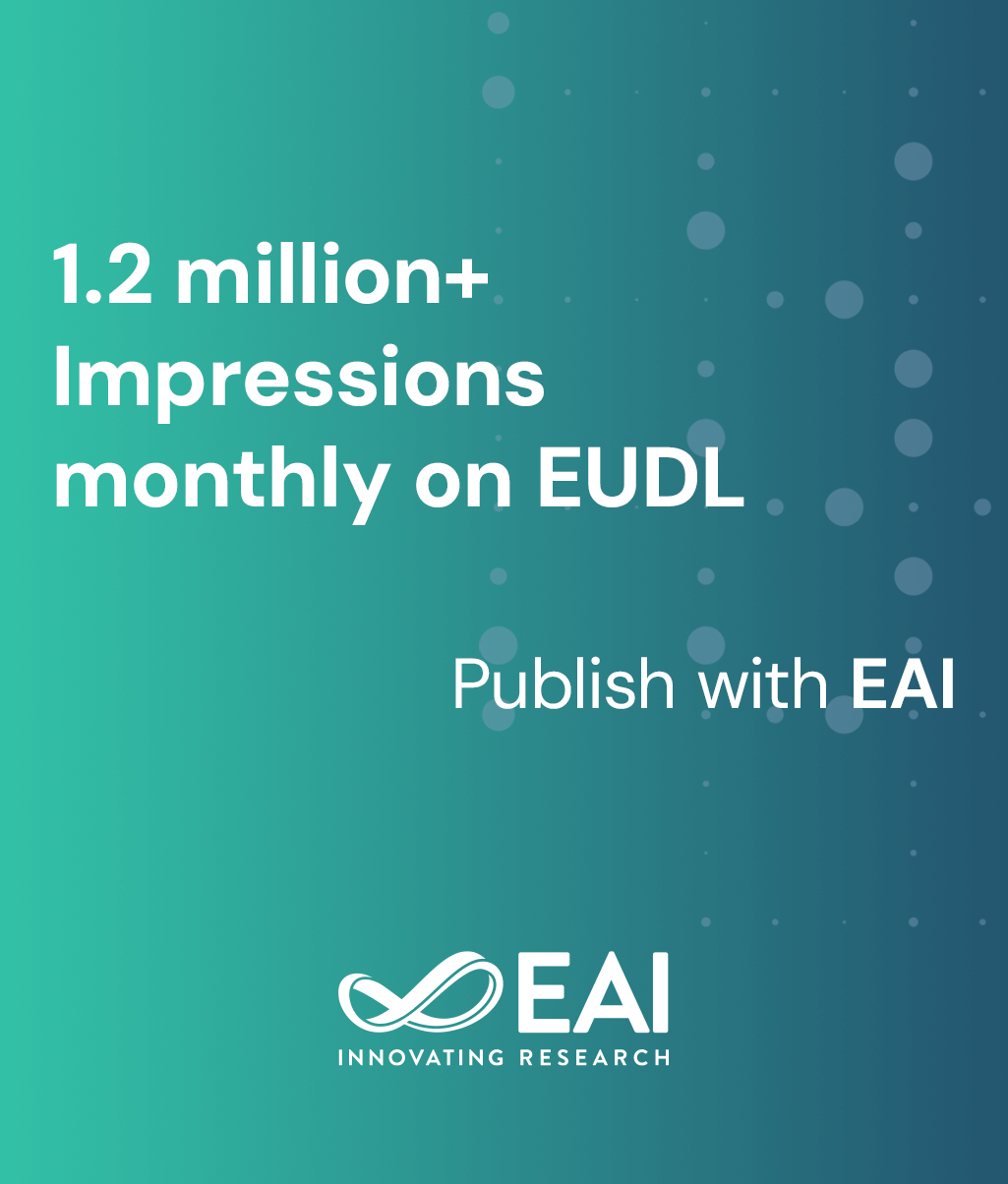
Research Article
Prediction of User Attrition in Telecommunication Using Neural Network
@ARTICLE{10.4108/eetsis.5242, author={Mageshkumar N and Vijayaraj A and Subba Reddy Chavva and Gururama Senthilvel}, title={Prediction of User Attrition in Telecommunication Using Neural Network}, journal={EAI Endorsed Transactions on Scalable Information Systems}, volume={11}, number={4}, publisher={EAI}, journal_a={SIS}, year={2024}, month={2}, keywords={Component, Churn in Telecom, Feature Selection, Data Analysis, Telecom Industry, Data mining, Classification}, doi={10.4108/eetsis.5242} }
- Mageshkumar N
Vijayaraj A
Subba Reddy Chavva
Gururama Senthilvel
Year: 2024
Prediction of User Attrition in Telecommunication Using Neural Network
SIS
EAI
DOI: 10.4108/eetsis.5242
Abstract
INTRODUCTION: The telecommunications industry faces significant challenges due to customer attrition, which directly impacts revenue. To better understand and address this issue, Companies are looking into techniques to determine the internal issues that affect customer churn. OBJECTIVES: This article offers an overview of customer attrition within the telecommunications sector. METHODS: It introduces an advanced churn prediction model harnessing state-of-the-art technologies, including neural networks, machine learning, and other cutting-edge innovations, to achieve remarkably high accuracy rates. By analyzing diverse parameters and datasets collected from multiple telecom companies, valuable insights can be gained. RESULTS: The model's performance on test data can be evaluated using metrics such as Accuracy Score, Area under Curve (AUC), Sensitivity, Specificity, and other performance indicators. CONCLUSION: In order to effectively manage extensive datasets, organizations can leverage Big Data technology. This empowers them to forecast the probability of customer churn and put in place proactive strategies to retain their customer base.
Copyright © 2024 Mageshkumar N et al., licensed to EAI. This is an open access article distributed under the terms of the CC BYNC-SA 4.0, which permits copying, redistributing, remixing, transformation, and building upon the material in any medium so long as the original work is properly cited.