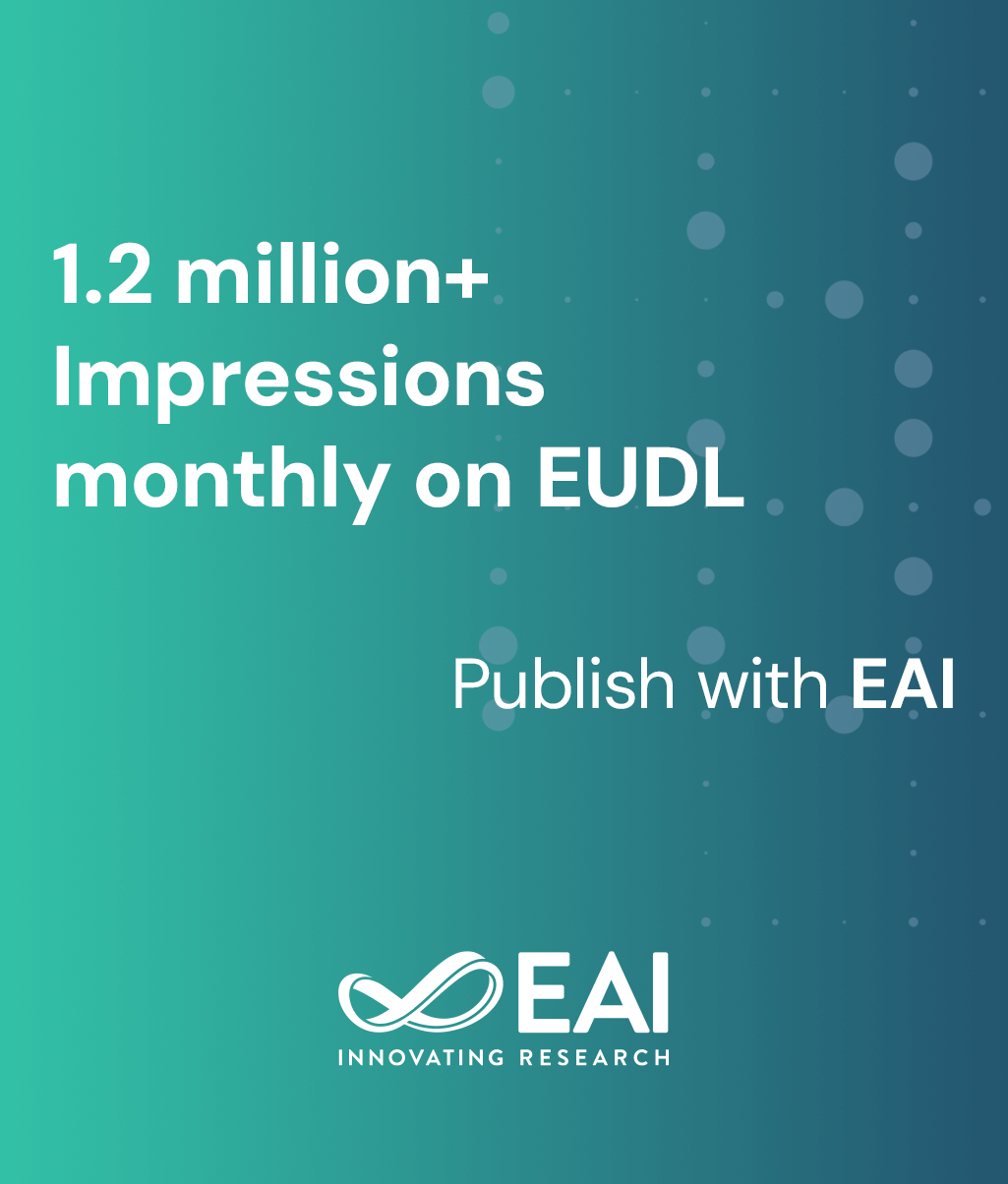
Research Article
Assessment of Global Forest Coverage through Machine Learning Algorithms
@ARTICLE{10.4108/eetsis.5122, author={P S Metkewar and Ravi Chauhan and A Prasanth and Malathy Sathyamoorthy}, title={Assessment of Global Forest Coverage through Machine Learning Algorithms}, journal={EAI Endorsed Transactions on Scalable Information Systems}, volume={11}, number={4}, publisher={EAI}, journal_a={SIS}, year={2024}, month={2}, keywords={Forest Coverage, Deforestation, Remote Sensing, Ground Surveys, Environmental Issues, Climate Change, Machine Learning, Mean Squared error, R2 Score, Mean Absolute Error, Root Mean Square Error}, doi={10.4108/eetsis.5122} }
- P S Metkewar
Ravi Chauhan
A Prasanth
Malathy Sathyamoorthy
Year: 2024
Assessment of Global Forest Coverage through Machine Learning Algorithms
SIS
EAI
DOI: 10.4108/eetsis.5122
Abstract
This exploration of paper presents an investigation of the Forest Region Inclusion Dataset that gives data on the backwoods inclusion of different nations overall from 1990 to 2020. The dataset contains country-wise information on population, population density, population development rate, total population rate, and forest region inclusion. We examined this dataset to decide the patterns in woodland region inclusion across various nations and mainlands, as well as the connection among populace and backwoods region inclusion. Our discoveries show that while certain nations have essentially expanded their forest region inclusion, others have encountered a decline. Besides, we found that population density and development rate are adversely related with forest area coverage. Authors have implemented four machine learning algorithms that are Linear Regression, Decision Tree, Random Forest and Support Vector Machine on the dataset.
Copyright © 2024 P. S. Metkewar et al., licensed to EAI. This is an open access article distributed under the terms of the CC BYNC-SA 4.0, which permits copying, redistributing, remixing, transformation, and building upon the material in any medium so long as the original work is properly cited.