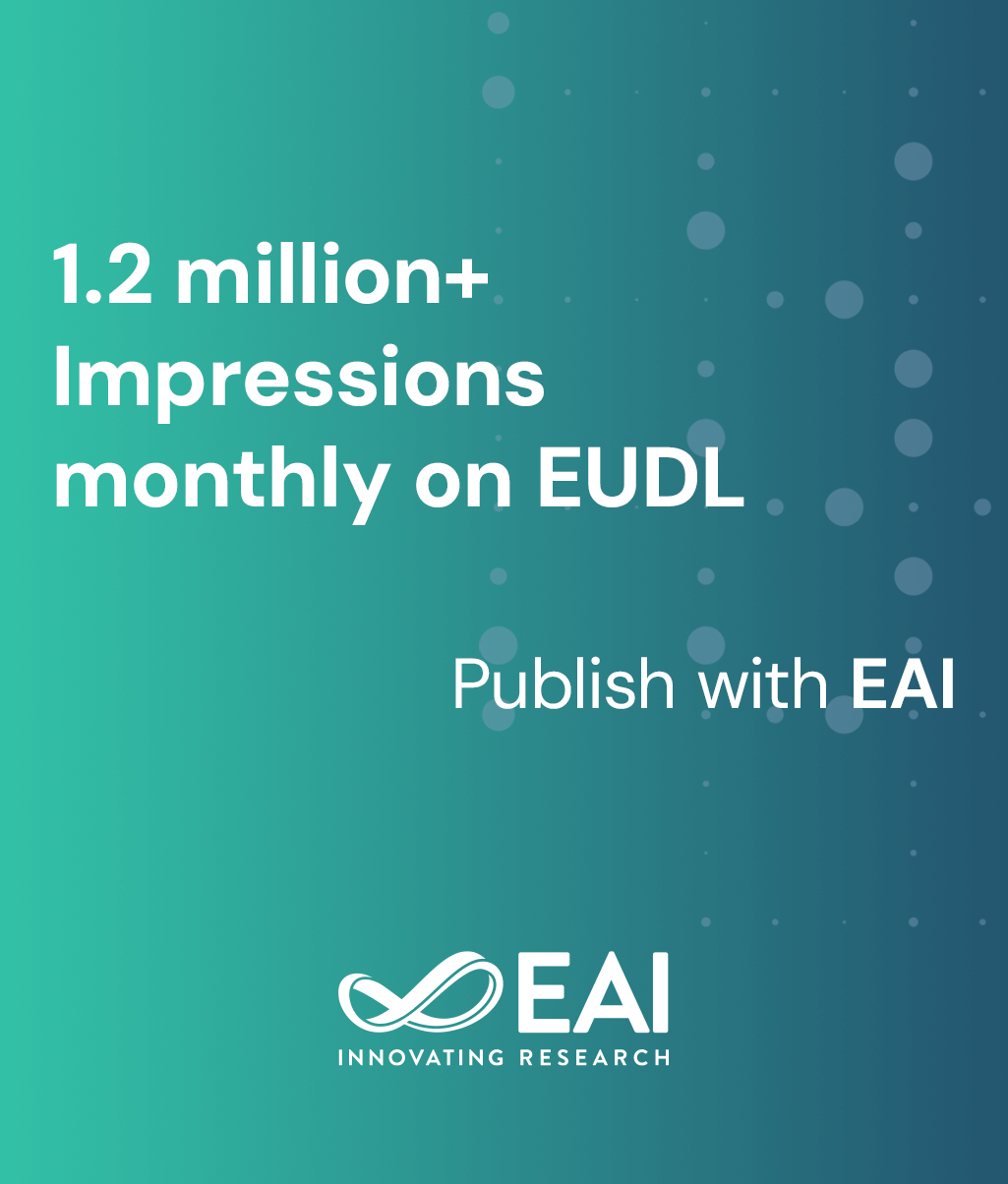
Research Article
Auto imputation enabled deep Temporal Convolutional Network (TCN) model for pm2.5 forecasting
@ARTICLE{10.4108/eetsis.5102, author={K. Krishna Rani Samal}, title={Auto imputation enabled deep Temporal Convolutional Network (TCN) model for pm2.5 forecasting}, journal={EAI Endorsed Transactions on Scalable Information Systems}, volume={12}, number={1}, publisher={EAI}, journal_a={SIS}, year={2024}, month={7}, keywords={Deep learning, Pollution, Imputation, Forecasting, PM2.5, TCN}, doi={10.4108/eetsis.5102} }
- K. Krishna Rani Samal
Year: 2024
Auto imputation enabled deep Temporal Convolutional Network (TCN) model for pm2.5 forecasting
SIS
EAI
DOI: 10.4108/eetsis.5102
Abstract
Data imputation of missing values is one of the critical issues for data engineering, such as air quality modeling. It is challenging to handle missing pollutant values because they are collected at irregular and different times. Accurate estimation of those missing values is critical for the air pollution prediction task. Effective forecasting is a significant part of air quality modeling for a robust early warning system. This study developed a neural network model, a Temporal Convolutional Network (TCN) with an imputation block (TCN-I), to simultaneously perform data imputation and forecasting tasks. As pollution sensor data suffer from different types of missing values whose causes are varied, TCN is attempted to impute those missing values in this study and perform prediction tasks in a single model. The results prove that the TCN-I model outperforms the baseline models.
Copyright © 2024 K. K. R. Samal et al., licensed to EAI. This is an open access article distributed under the terms of the CC BY-NC-SA 4.0, which permits copying, redistributing, remixing, transformation, and building upon the material in any medium so long as the original work is properly cited.