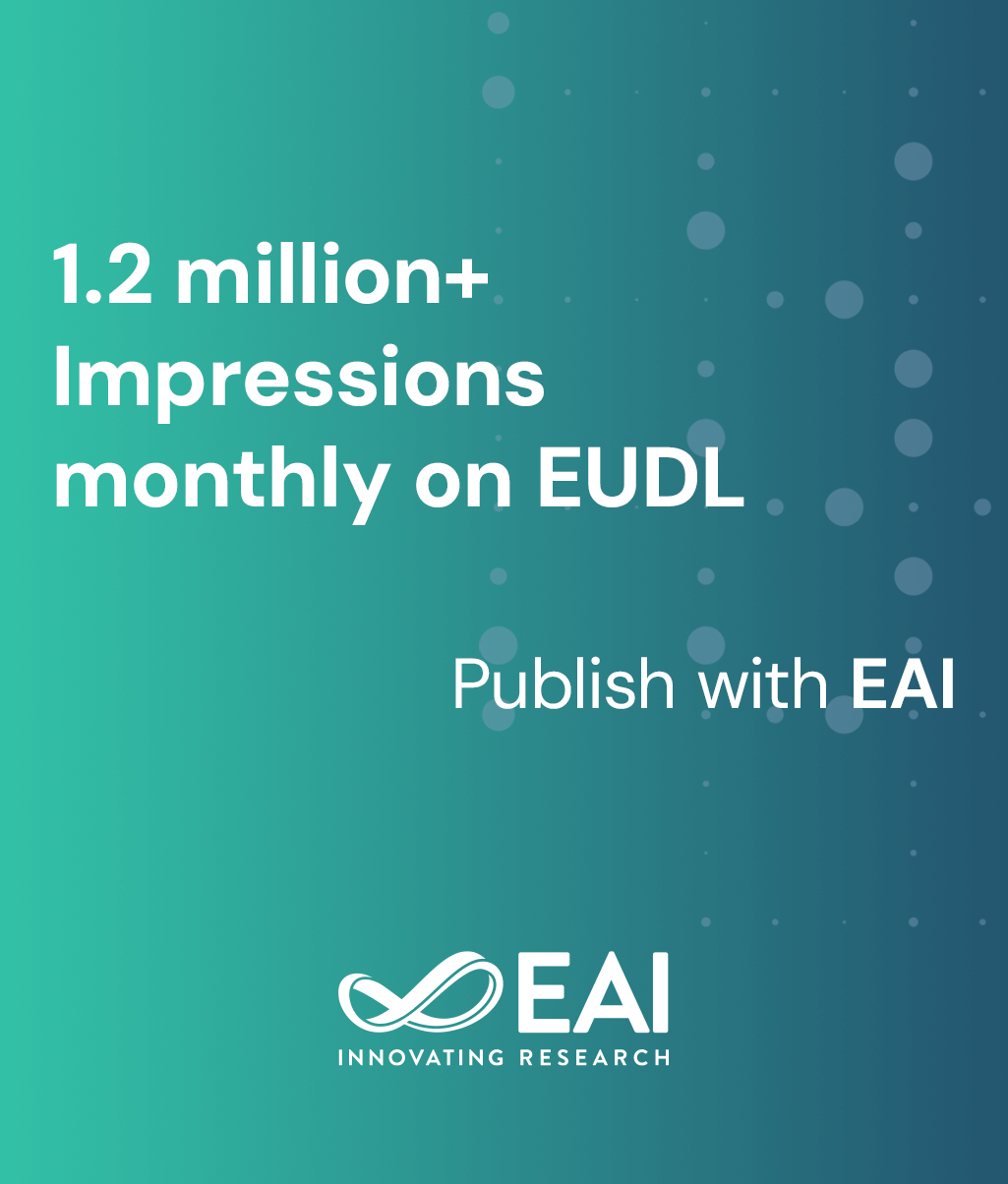
Research Article
Data Aggregation through Hybrid Optimal Probability in Wireless Sensor Networks
@ARTICLE{10.4108/eetsis.4996, author={S Balaji and S Jeevanandham and Mani Deepak Choudhry and M Sundarrajan and Rajesh Kumar Dhanaraj}, title={Data Aggregation through Hybrid Optimal Probability in Wireless Sensor Networks}, journal={EAI Endorsed Transactions on Scalable Information Systems}, volume={11}, number={4}, publisher={EAI}, journal_a={SIS}, year={2024}, month={2}, keywords={WSN, Data Collection, Energy Efficient, Probabalistic, LEACH, Secure Protocol}, doi={10.4108/eetsis.4996} }
- S Balaji
S Jeevanandham
Mani Deepak Choudhry
M Sundarrajan
Rajesh Kumar Dhanaraj
Year: 2024
Data Aggregation through Hybrid Optimal Probability in Wireless Sensor Networks
SIS
EAI
DOI: 10.4108/eetsis.4996
Abstract
INTRODUCTION: In the realm of Wireless Sensor Networks (WSN), effective data dissemination is vital for applications like traffic alerts, necessitating innovative solutions to tackle challenges such as broadcast storms. OBJECTIVES: This paper proposes a pioneering framework that leverages probabilistic data aggregation to optimize communication efficiency and minimize redundancy. METHODS: The proposed adaptable system extracts valuable insights from the knowledge base, enabling dynamic route adjustments based on application-specific criteria. Through simulations addressing bandwidth limitations and local broadcast issues, we establish a robust WSN-based traffic information system. RESULTS: By employing primal-dual decomposition, the proposed approach identifies optimal packet aggregation probabilities and durations, resulting in reduced energy consumption while meeting latency requirements. CONCLUSION: The efficacy of proposed method is demonstrated across various traffic and topology scenarios, affirming that probabilistic data aggregation effectively mitigates the local broadcast problem, ultimately leading to decreased bandwidth demands.
Copyright © 2024 S. Balaji et al., licensed to EAI. This is an open access article distributed under the terms of the CC BY-NC-SA 4.0, which permits copying, redistributing, remixing, transformation, and building upon the material in any medium so long as the original work is properly cited.