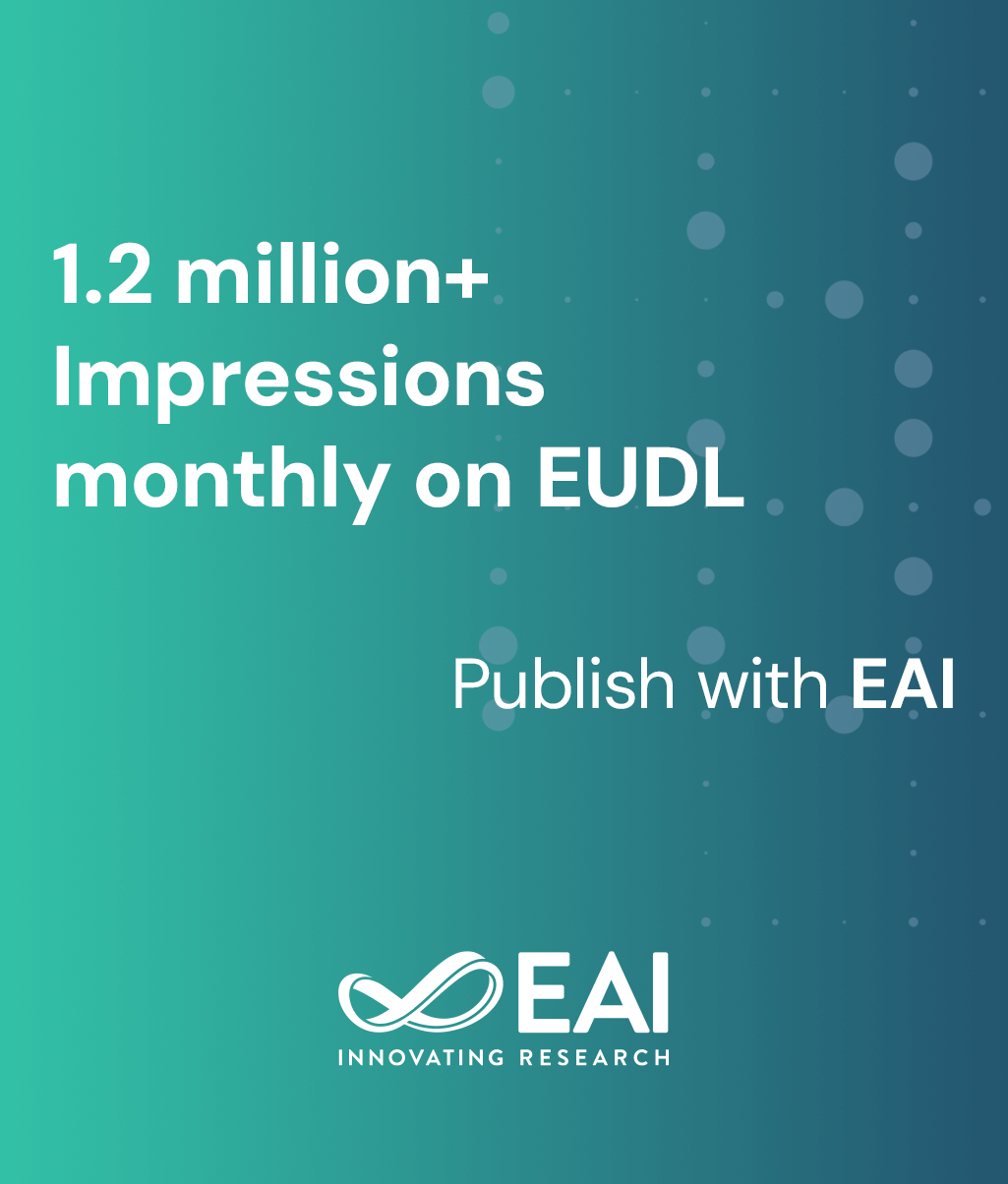
Research Article
Research on Music Classification Technology Based on Integrated Deep Learning Methods
@ARTICLE{10.4108/eetsis.4954, author={Sujie He and Yuxian Li}, title={Research on Music Classification Technology Based on Integrated Deep Learning Methods}, journal={EAI Endorsed Transactions on Scalable Information Systems}, volume={11}, number={5}, publisher={EAI}, journal_a={SIS}, year={2025}, month={4}, keywords={deep learning, music classification, convolutional networks, recurrent neural networks}, doi={10.4108/eetsis.4954} }
- Sujie He
Yuxian Li
Year: 2025
Research on Music Classification Technology Based on Integrated Deep Learning Methods
SIS
EAI
DOI: 10.4108/eetsis.4954
Abstract
INTRODUCTION: Music classification techniques are of great importance in the current era of digitized music. With the dramatic increase in music data, effectively categorizing music has become a challenging task. Traditional music classification methods have some limitations, so this study aims to explore music classification techniques based on integrated deep-learning methods to improve classification accuracy and robustness. OBJECTIVES: The purpose of this study is to improve the performance of music classification by using an integrated deep learning approach that combines the advantages of different deep learning models. The author aims to explore the effectiveness of this approach in coping with the diversity and complexity of music and to compare its performance differences with traditional approaches. METHODS: The study employs several deep learning models including, but not limited to, Convolutional Neural Networks (CNN), Recurrent Neural Networks (RNN), and Long Short-Term Memory Networks (LSTM). These models were integrated into an overall framework to perform the final music classification by combining their predictions. The training dataset contains rich music samples covering different styles, genres and emotions. RESULTS: Experimental results show that music classification techniques based on integrated deep learning methods perform better in terms of classification accuracy and robustness compared to traditional methods. The advantages of integrating different deep learning models are fully utilized, enabling the system to better adapt to different types of music inputs. CONCLUSION: This study demonstrates the effectiveness of the integrated deep learning approach in music classification tasks and provides valuable insights for further improving music classification techniques. This approach not only improves the classification performance but also promises to be applied to other areas and promote the application of deep learning techniques in music analysis.
Copyright © 2024 He & Li et al., licensed to EAI. This is an open access article distributed under the terms of the CC BY-NC-SA 4.0, which permits copying, redistributing, remixing, transformation, and building upon the material in any medium so long as the original work is properly cited.