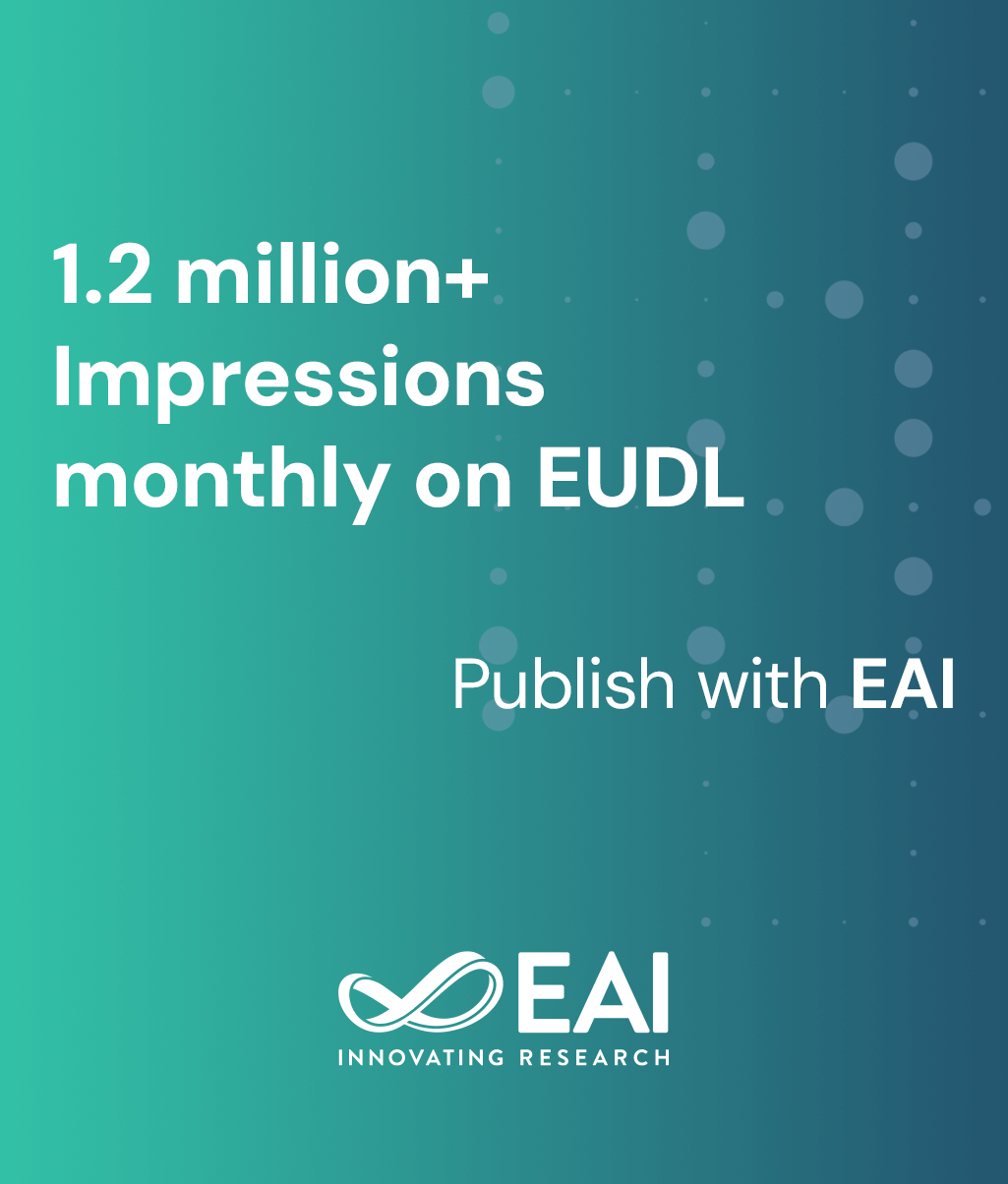
Research Article
Stock Price Prediction using Multi-Layered Sequential LSTM
@ARTICLE{10.4108/eetsis.4585, author={Jyoti Prakash Behura}, title={Stock Price Prediction using Multi-Layered Sequential LSTM}, journal={EAI Endorsed Transactions on Scalable Information Systems}, volume={11}, number={4}, publisher={EAI}, journal_a={SIS}, year={2023}, month={12}, keywords={Forecasting, deep learning, stock prices, RNN, CNN, MLS-LSTM, Adam optimizer, NSE, ARIMA, SVM}, doi={10.4108/eetsis.4585} }
- Jyoti Prakash Behura
Year: 2023
Stock Price Prediction using Multi-Layered Sequential LSTM
SIS
EAI
DOI: 10.4108/eetsis.4585
Abstract
Stock markets are frequently among the most volatile locations to invest in. The choice to buy or sell stocks is heavily influenced by statistical analysis of prior stock performance and external circumstances. All these variables are employed to maximize profitability. Stock value prediction is a hard undertaking that necessitates a solid computational foundation to compute longer-term share values. Stock prices are connected inside the market, making it harder to forecast expenses. Financial data is a category that includes past data from time series that provides a lot of knowledge and is frequently employed in data analysis tasks. This research provides a unique optimisation strategy for stock price prediction based on a Multi-Layer Sequential Long Short Term Memory (MLS LSTM) model and the adam optimizer in this context. Furthermore, to make reliable predictions, the MLS LSTM algorithm uses normalised time series data separated into time steps to assess the relationship between past and future values. Furthermore, it solves the vanishing gradient problem that plagues basic recurrent neural networks.
Copyright © 2023 J. P. Behura et al., licensed to EAI. This is an open access article distributed under the terms of the CC BY-NC-SA 4.0, which permits copying, redistributing, remixing, transformation, and building upon the material in any medium so long as the original work is properly cited.